A Transformational Characterization of Unconditionally Equivalent Bayesian Networks
CoRR(2022)
摘要
We consider the problem of characterizing Bayesian networks up to unconditional equivalence, i.e., when directed acyclic graphs (DAGs) have the same set of unconditional $d$-separation statements. Each unconditional equivalence class (UEC) is uniquely represented with an undirected graph whose clique structure encodes the members of the class. Via this structure, we provide a transformational characterization of unconditional equivalence; i.e., we show that two DAGs are in the same UEC if and only if one can be transformed into the other via a finite sequence of specified moves. We also extend this characterization to the essential graphs representing the Markov equivalence classes (MECs) in the UEC. UECs partition the space of MECs and are easily estimable from marginal independence tests. Thus, a characterization of unconditional equivalence has applications in methods that involve searching the space of MECs of Bayesian networks.
更多查看译文
关键词
bayesian networks,transformational characterization
AI 理解论文
溯源树
样例
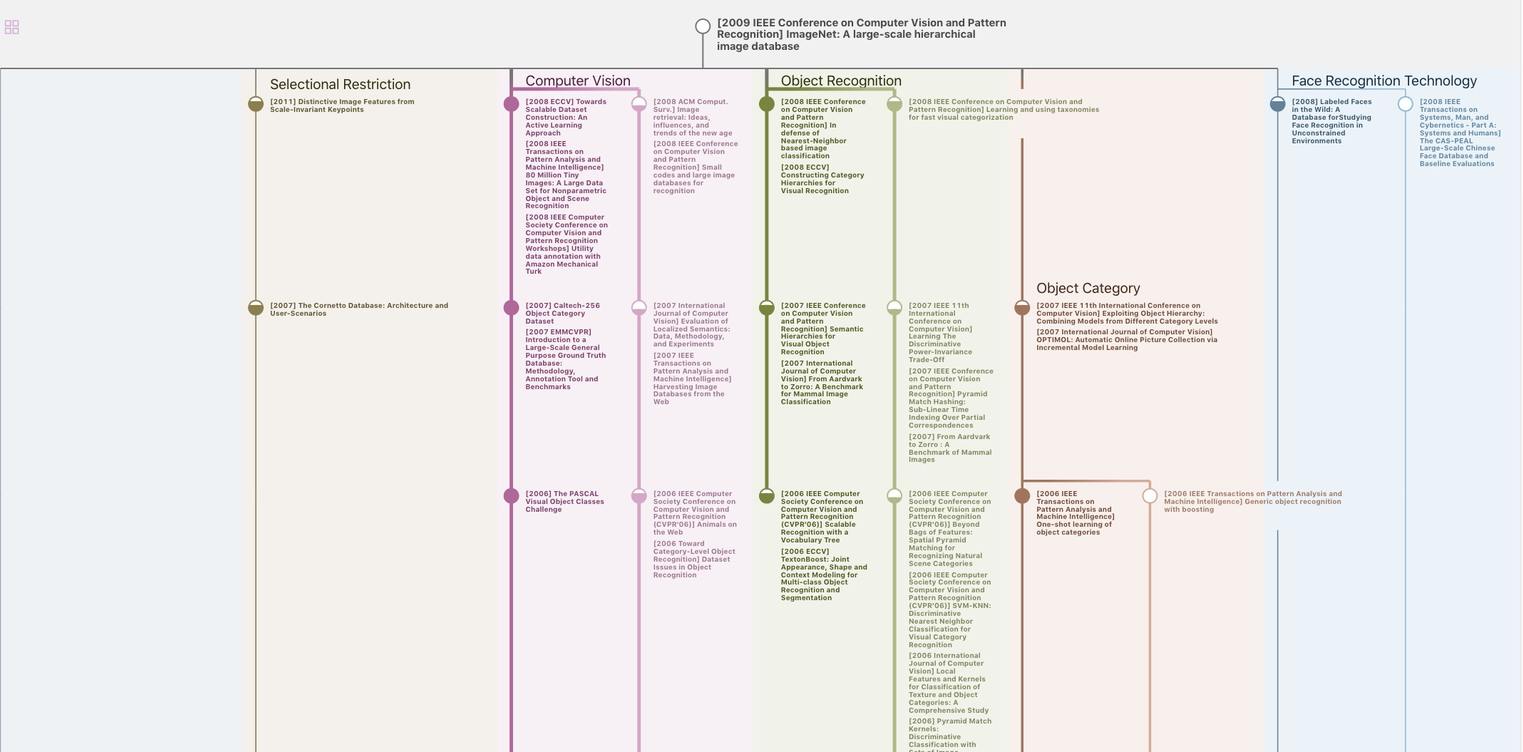
生成溯源树,研究论文发展脉络
Chat Paper
正在生成论文摘要