DreamingV2: Reinforcement Learning with Discrete World Models without Reconstruction.
IEEE/RJS International Conference on Intelligent RObots and Systems (IROS)(2022)
摘要
The present paper proposes a novel reinforcement learning method with world models, DreamingV2, a collaborative extension of DreamerV2 and Dreaming. DreamerV2 is a cutting-edge model-based reinforcement learning from pixels that uses discrete world models to represent latent states with categorical variables. Dreaming is also a form of reinforcement learning from pixels that attempts to avoid the autoencoding process in general world model training by involving a reconstruction-free contrastive learning objective. The proposed DreamingV2 is a novel approach of adopting both the discrete representation of DreamingV2 and the reconstruction-free objective of Dreaming. Compared to DreamerV2 and other recent model-based methods without reconstruction, DreamingV2 achieves the best scores on five simulated challenging 3D robot arm tasks. We believe that DreamingV2 will be a reliable solution for robot learning since its discrete representation is suitable to describe discontinuous environments, and the reconstruction-free fashion well manages complex vision observations.
更多查看译文
关键词
discrete world models,reinforcement learning
AI 理解论文
溯源树
样例
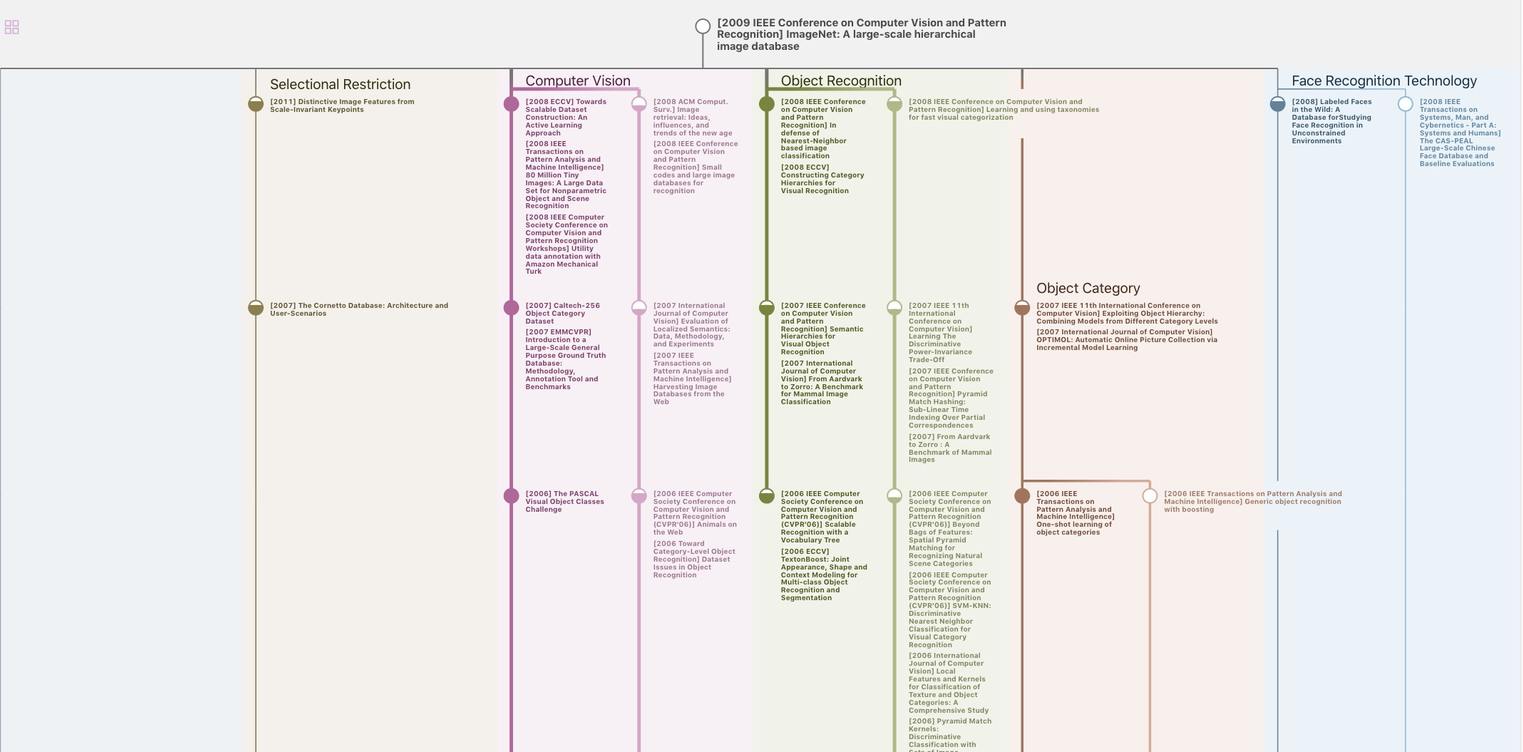
生成溯源树,研究论文发展脉络
Chat Paper
正在生成论文摘要