Deep Feature Mining via the Attention-Based Bidirectional Long Short Term Memory Graph Convolutional Neural Network for Human Motor Imagery Recognition
FRONTIERS IN BIOENGINEERING AND BIOTECHNOLOGY(2022)
摘要
Recognition accuracy and response time are both critically essential ahead of building the practical electroencephalography (EEG)-based brain-computer interface (BCI). However, recent approaches have compromised either the classification accuracy or the responding time. This paper presents a novel deep learning approach designed toward both remarkably accurate and responsive motor imagery (MI) recognition based on scalp EEG. Bidirectional long short-term memory (BiLSTM) with the attention mechanism is employed, and the graph convolutional neural network (GCN) promotes the decoding performance by cooperating with the topological structure of features, which are estimated from the overall data. Particularly, this method is trained and tested on the short EEG recording with only 0.4 s in length, and the result has shown effective and efficient prediction based on individual and groupwise training, with 98.81% and 94.64% accuracy, respectively, which outperformed all the state-of-the-art studies. The introduced deep feature mining approach can precisely recognize human motion intents from raw and almost-instant EEG signals, which paves the road to translate the EEG-based MI recognition to practical BCI systems.
更多查看译文
关键词
brain-computer interface (BCI), electroencephalography (EEG), motor imagery (MI), bidirectional long short-term memory (BiLSTM), graph convolutional neural network (GCN)
AI 理解论文
溯源树
样例
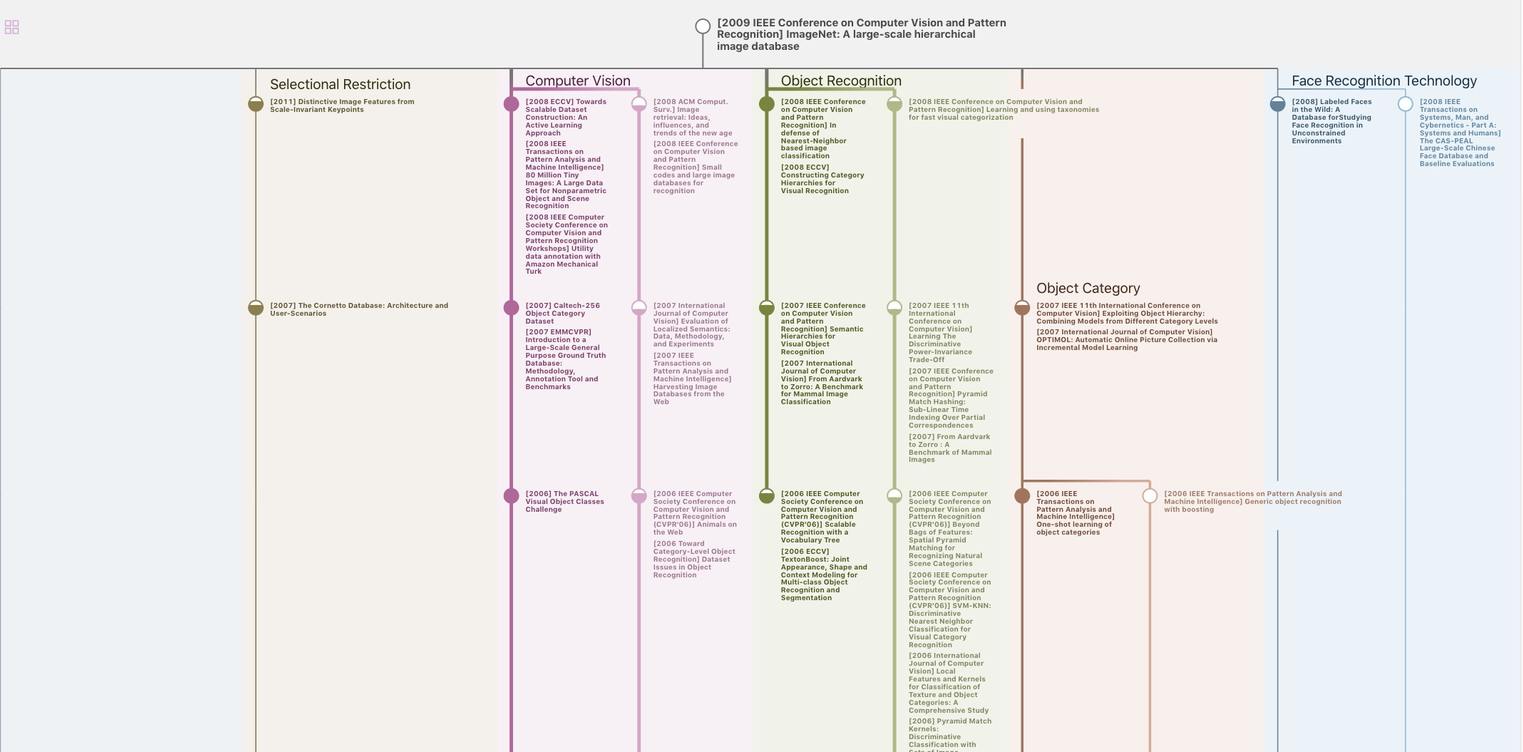
生成溯源树,研究论文发展脉络
Chat Paper
正在生成论文摘要