Sirius: Sequential Recommendation with Feature Augmented Graph Neural Networks
Lecture Notes in Computer Science(2021)
摘要
Many practical recommender systems recommend personalized items for different users by mining user-item interaction sequences. The interaction sequences, as a whole, imply the manifold collaborative relations among users and items. Further, from the view of users, the item orders and time intervals between interactions could expose the evolution of user interests, and from the view of items, attributes of the items on interaction sequences may reveal the variation of item popularity. However, most of the existing recommendation models ignore those valuable information, and cannot fully explore the intrinsic implication of interaction sequences. In the paper, we propose a method named Sirius, which develops GNNs (Graph Neural Networks) to model the collaborative relations and capture the dynamics of time and attribute features in sequences. We give the workflow of the Sirius method, and describe the implementations about graph construction, item embedding generation, sequence embedding generation and next-item prediction. Finally, we give an example of Sirius recommendations, which visually shows the impact of feature information on the recommendation results. At present, Sirius has been adopted by MX Player, one of India’s largest streaming platforms, recommending movies for thousands of users.
更多查看译文
关键词
Recommender system,Deep learning,Graph neural network,Sequential recommendation
AI 理解论文
溯源树
样例
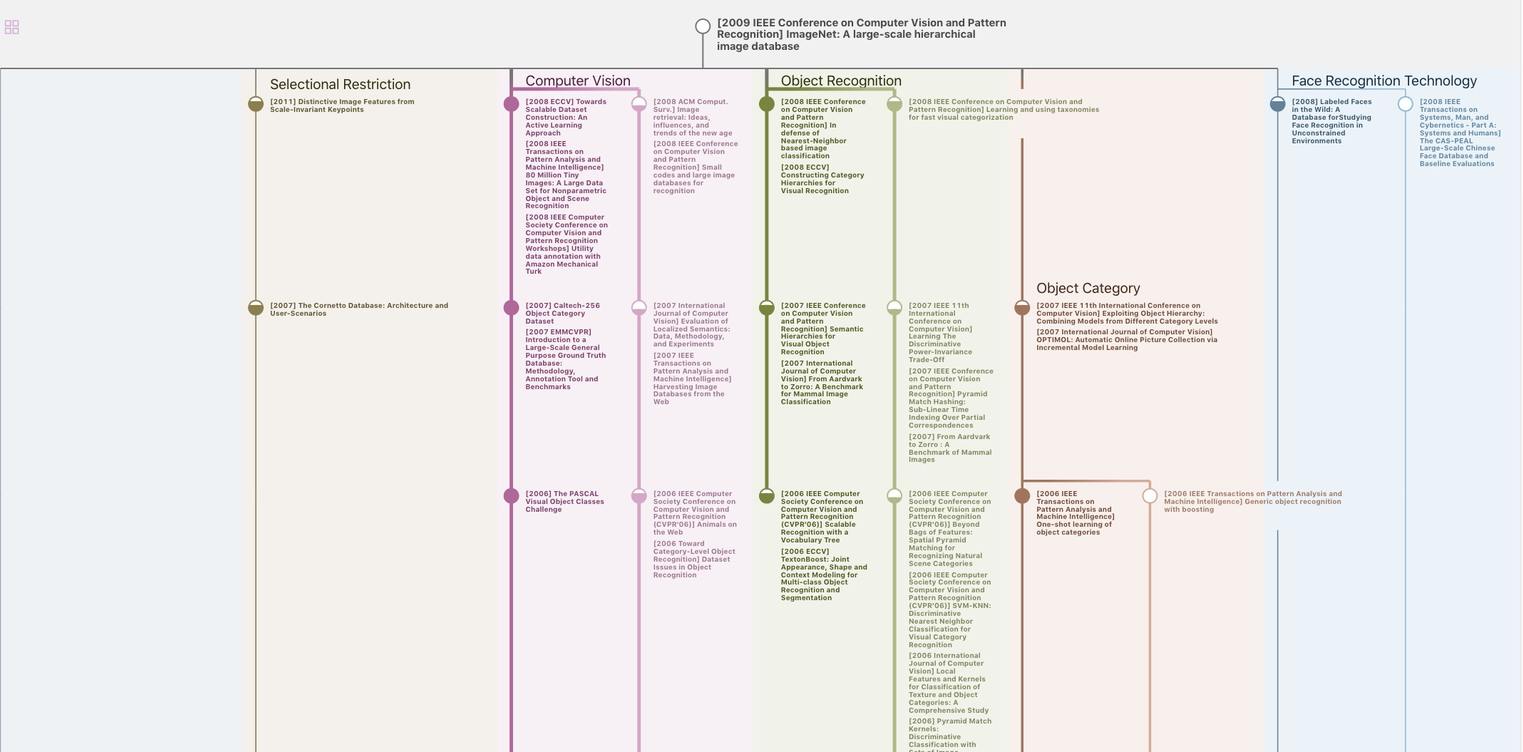
生成溯源树,研究论文发展脉络
Chat Paper
正在生成论文摘要