Artificial Neural Network-Based ECG Signal Classification and the Cardiac Arrhythmia Identification
Lecture notes in networks and systems(2021)
Abstract
Electrocardiogram is an essential tool to determine the clinical condition of cardiac muscle. An immediate and the precise detection of cardiac arrhythmia is highly preferred for aiding good and healthy life, and it leads to healthy survival for the humans. In this study, utilizing MATLAB tools the feature extraction is made by various statistical parameters from both normal and the abnormal categorization of ECG signals. These features are inclusive of variance, arithmetic mean, kurtosis, standard deviation, and skewness. The feature vector values reveal the informational data with respect to a cardiac clinical health state. The focus on this study is made by utilizing the classifier of artificial neural network in order to identify the ECG abnormalities. Levenberg–Marquardt backpropagation neural network (LM-BPNN) technique is being utilized for the cardiac arrhythmia classification. The ECG data are extracted from the MIT-BIH cardiac arrhythmia database, and the data are tested which is utilized for further classification of ECG arrhythmia. The comparison for the results of classification is made in terms of accuracy, positive predictivity, sensitivity, and specificity. The results of experimentation have been validated based on its accuracy of classification through tenfold cross-validation technique. It has resulted with an average accuracy of 99.5% in predicting the cardiac arrhythmias of a different class.
MoreTranslated text
Key words
Artificial neural networks, Electrocardiogram, Feature extraction, Classification, MIT-BIH arrhythmia database, Levenberg–Marquardt (LM) algorithm
AI Read Science
Must-Reading Tree
Example
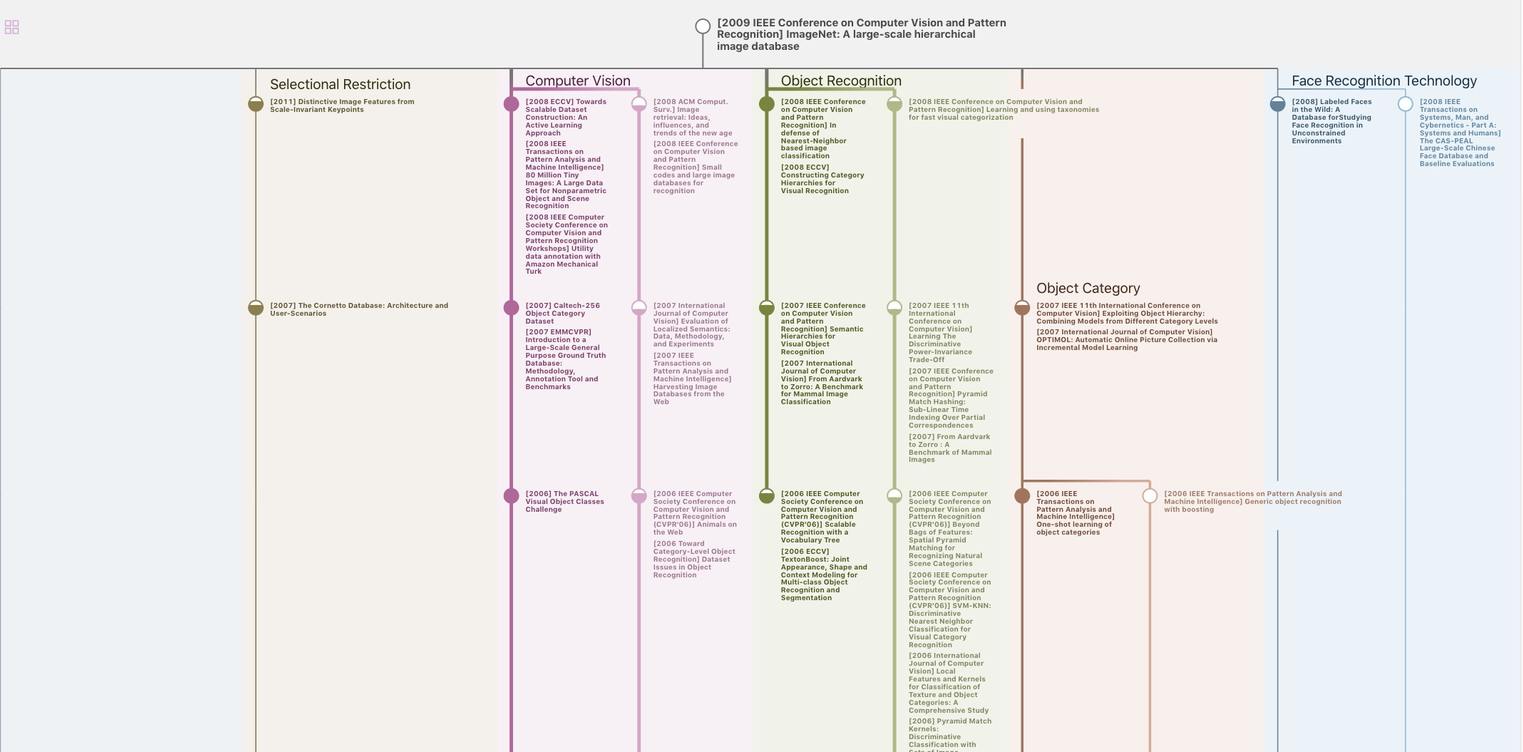
Generate MRT to find the research sequence of this paper
Chat Paper
Summary is being generated by the instructions you defined