NSRL: Named Entity Recognition with Noisy Labels via Selective Review Learning
Communications in computer and information science(2021)
摘要
Named entity recognition (NER) is a task of identifying both types and spans in the sentences. Previous works always assume that the NER datasets are correctly annotated. However, not all samples help with generalization. There are many noisy samples from a variety of sources (e.g., weak, pseudo, or distant annotations). Meanwhile existing methods are prone to cause error propagation in self-training process because of ignoring the overfitting, and becomes particularly challenging. In this paper, we propose a robust Selective Review Learning (NSRL) framework for NER task with noisy labels. Specifically, we design a Status Loss Function (SLF) which helps the model review the previous knowledge continuously when learning new knowledge, and prevents model from overfitting noisy samples in self-training process. In addition, we propose a novel Confidence Estimate Mechanism (CEM), which utilizes the difference between logit values to identify positive samples. Experiments on four distant supervision datasets and two real-world datasets show that the NSRL significantly outperforms previous methods.
更多查看译文
关键词
Overfitting,Self-training,Status loss function,Confidence estimation
AI 理解论文
溯源树
样例
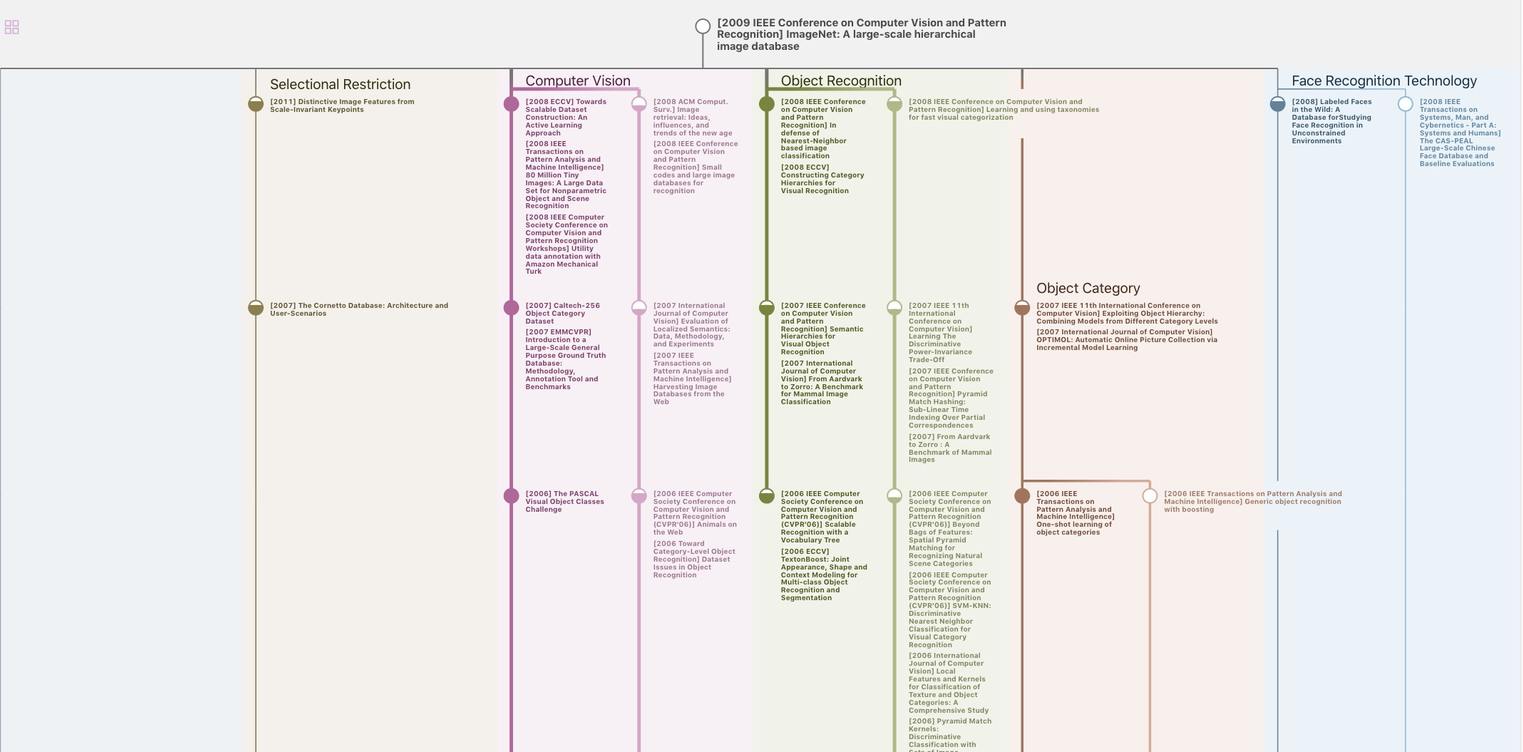
生成溯源树,研究论文发展脉络
Chat Paper
正在生成论文摘要