Effects of Domain Randomization on Simulation-to-Reality Transfer of Reinforcement Learning Policies for Industrial Robots
ADVANCES IN ARTIFICIAL INTELLIGENCE AND APPLIED COGNITIVE COMPUTING(2021)
摘要
A requirement for a significant amount of training as well as the exploration of potentially expensive or safety-critical states limits the applicability of reinforcement learning for real-world robotics. One potential solution is given by pretraining models in simulations before transferring them to the real world. In this chapter, we investigate the concept of domain randomization to train robust agents in simulation to control an industrial robot. We examine the effects of different degrees of randomization with respect to the transferability to the real world. In addition, we use attention maps to gain insights into the agents’ decision-making processes. We find that attention maps enable a qualitative assessment for the data-efficiency of a pretrained agent when transferred to the real-world setup.
更多查看译文
关键词
Simulation-to-Reality, Transfer Learning, Attention Maps, Deep Reinforcement Learning
AI 理解论文
溯源树
样例
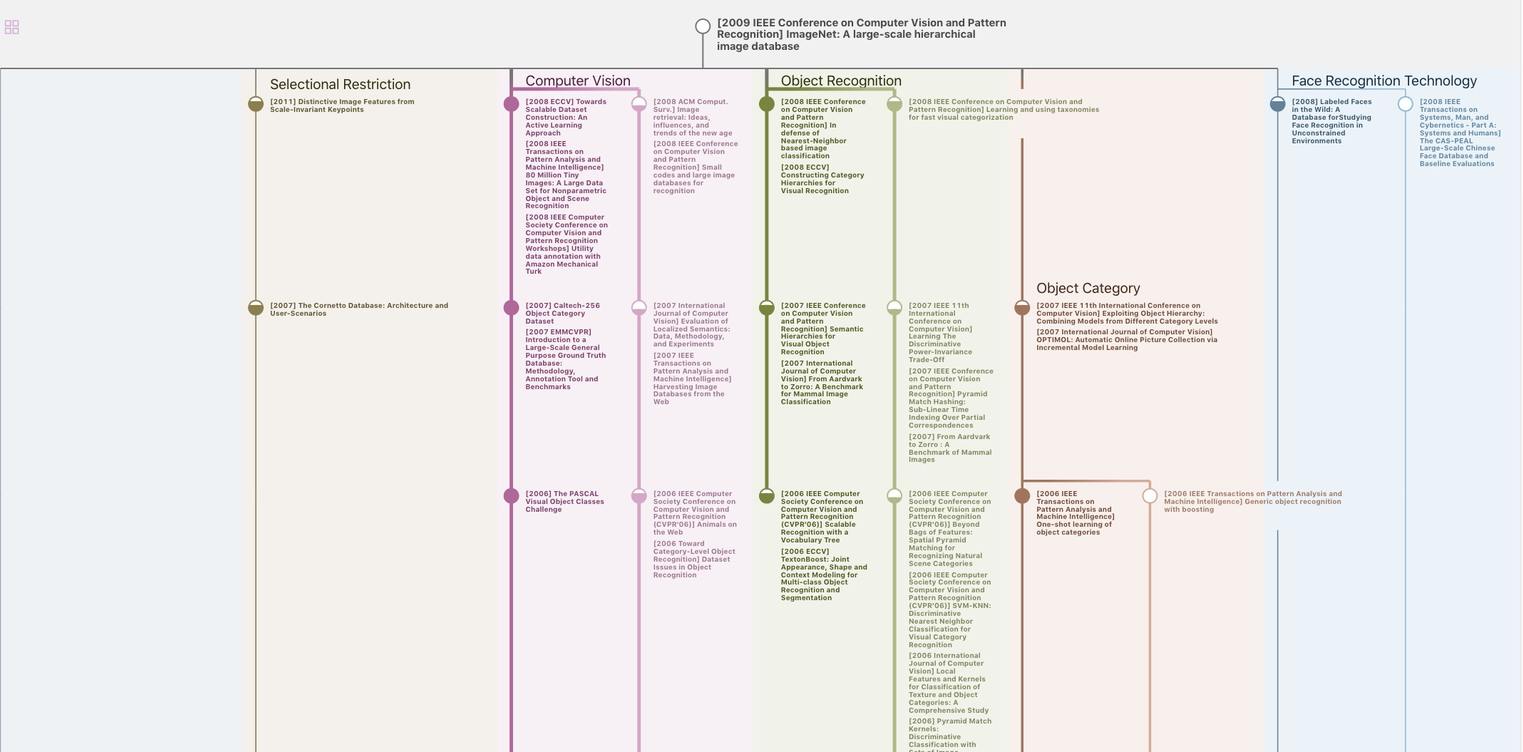
生成溯源树,研究论文发展脉络
Chat Paper
正在生成论文摘要