A Machine Learning Approach to the Early Diagnosis of Alzheimer’s Disease Based on an Ensemble of Classifiers
springer
摘要
The present article discusses the ability of an ensemble of machine learning models to implement classification strategies to discriminate among mild cognitive impairment (MCI), Alzheimer’s disease (AD) and Cognitive Unimpaired (CU). For this, a battery of games that assesses the most relevant markers for the early diagnosis of cognitive impairment (i.e., memory, executive functions, attention and gnosias) was implemented, and a pilot study was carried out with 64 individuals (28 CU, 16 MCI and 20 AD). Participants were administered a collection of classical pen-and-paper tests, and then interacted with all games in the battery. A set of four ensembles of classifiers were applied and relevant metrics were computed to assess the classification power of this approach. According to the classification metrics computed, best classification results are obtained using the Random Forest Classifier algorithm, with average F1 score = 0.99, accuracy = 1.00, and Cohen’s kappa = 0.98. The experiments performed indicate that artificial intelligence techniques and serious games can be used to automate some aspects of the clinical diagnosis of individuals with cognitive impairment. However, more research is needed to obtain the required normative data for clinical validity.
更多查看译文
关键词
Machine learning,Ensemble methods,Alzheimer’s disease,Early detection,Cognitive impairment,Serious games
AI 理解论文
溯源树
样例
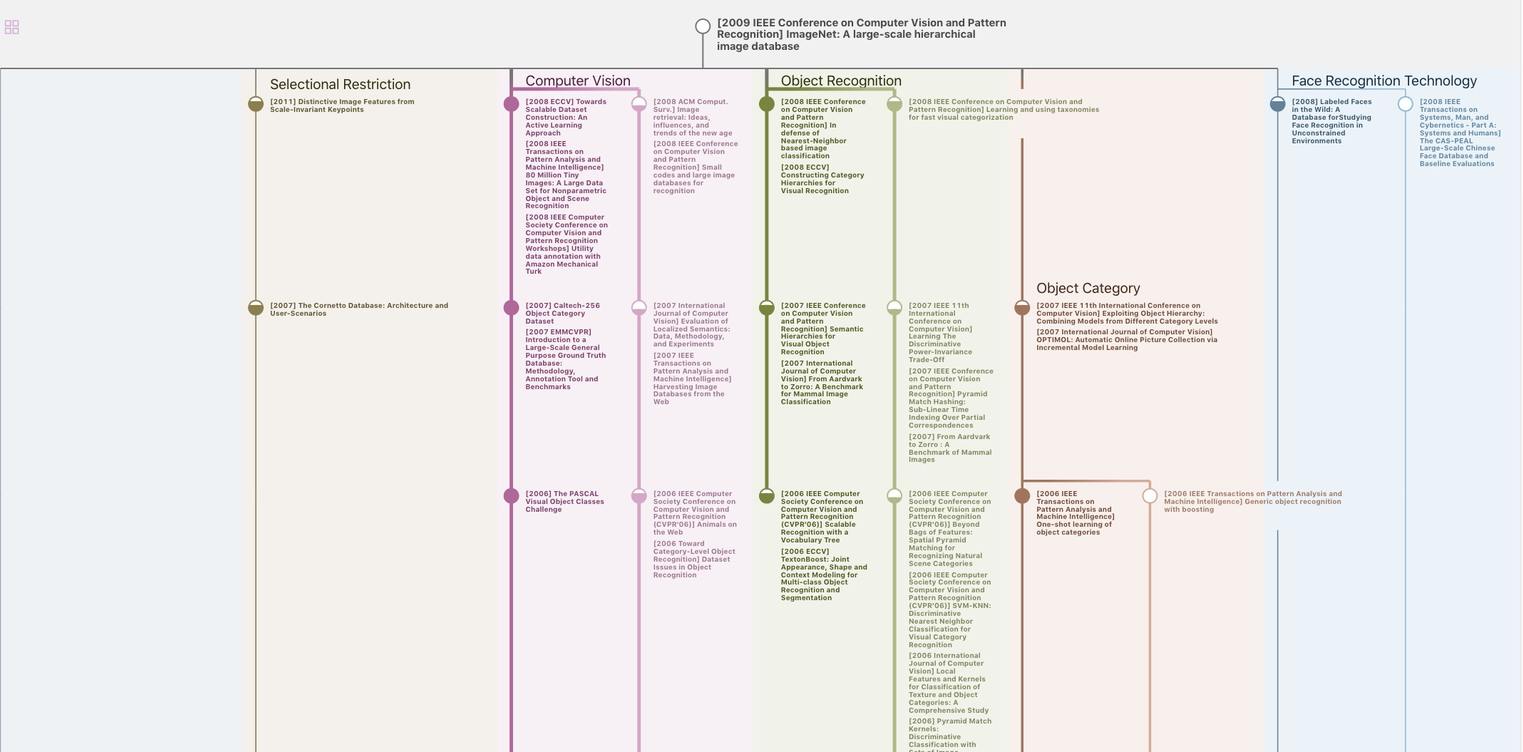
生成溯源树,研究论文发展脉络
Chat Paper
正在生成论文摘要