Ultrasound Image Preprocessing Method for Deep-Learning-Based Fatty Liver Diagnosis
Advances in Graphic Communication, Printing and Packaging Technology and MaterialsLecture Notes in Electrical Engineering(2021)
摘要
An iterative image processing method is proposed to improve the performance of Convolutional Neural Network (CNN) model for diagnosing fatty liver. A dataset that contains 17,400 ultrasound images of liver are used in this paper. These liver image samples are preprocessed for noise reduction and texture enhancement firstly. Then the processed image samples are used for training CNN model LeNet-5. According to the accuracy of the trained LeNet-5, the optimal combination of noise reduction and enhancement is obtained. The experimental results show that the accuracy of LeNet-5 model based on original data set is 80.58% and area under the Receiver Operation Characteristic (ROC) Curve value (AUC) is 0.574. And the optimal image processing combination is Butterworth low pass (BLP) and Exposure based Sub Image Histogram Equalization (ESIHE). The model accuracy is 10.01% higher than original data set, and reaches 90.59%. The AUC value of the optimal model has reached 0.845, which is 0.271 higher than the AUC value of the original model. Therefore, iterative image processing is a feasible method to improve the performance of CNN model in diagnosing fatty liver.
更多查看译文
关键词
Image processing, Convolutional neural network, Deep learning, Automatic diagnostic
AI 理解论文
溯源树
样例
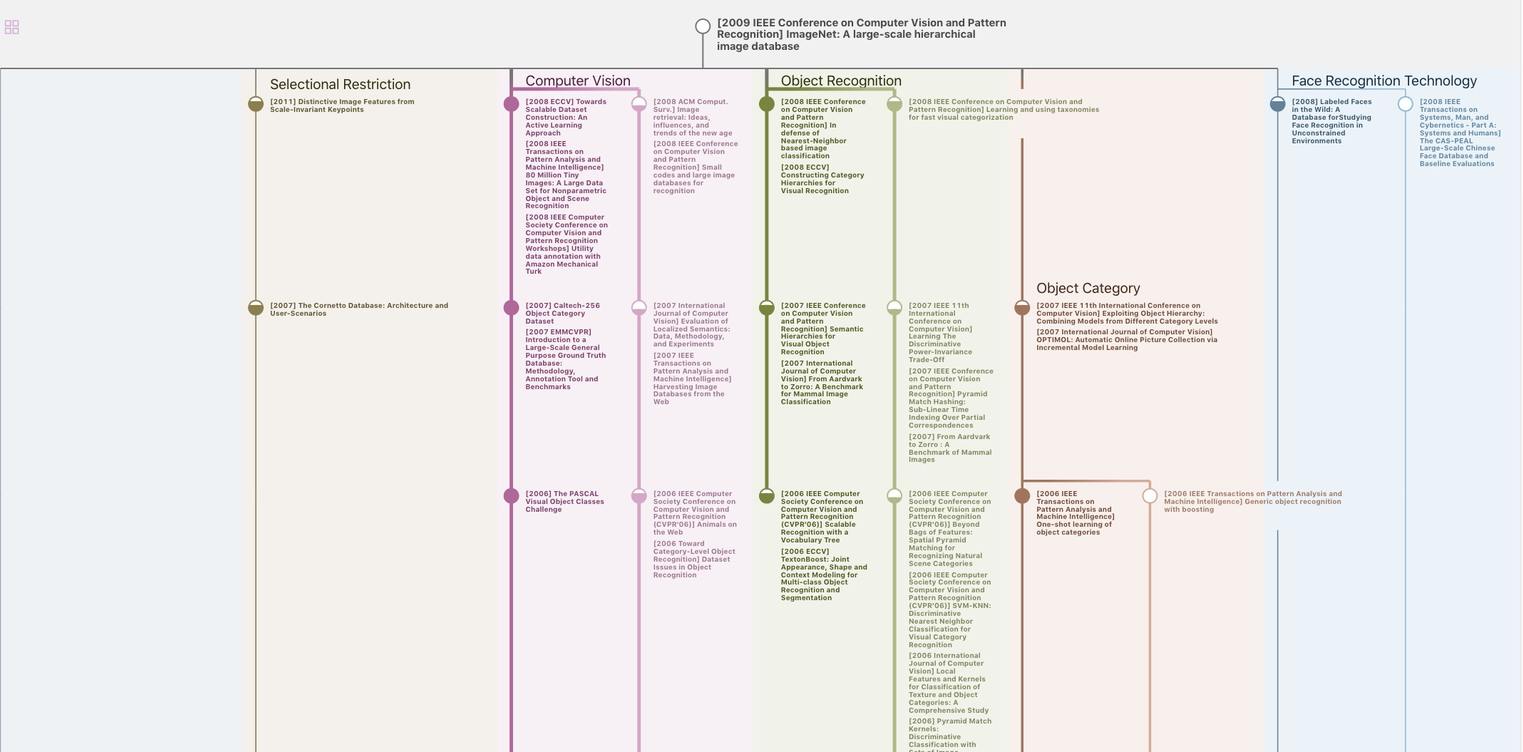
生成溯源树,研究论文发展脉络
Chat Paper
正在生成论文摘要