Robotic Grasp Synthesis Using Deep Learning Approaches: A Survey
Advances in intelligent systems and computing(2021)
Abstract
Deep learning methods are derived and inspired from the structure and activities of a brain. In an intricate state, learning from the past experiences helps human to accomplish the task in efficient way. This paper addresses such deep learning approaches practiced in the robotic grasp detection for an unknown object. A grasp is defined as lifting of an object by the end effector of robotic manipulator without tumbling. To realize this task, a robot must be able to identify the grasp area of the target object autonomously by means of visual or touch feedback. During the last decade, there is an enormous improvements in hardware computational capabilities, and hence, deep learning methods play a vital role in making robots autonomous. Review of such deep learning methods, used in robotic grasping, is carried out in this work. Deep learning is a subset of machine learning with more neurons then artificial neural networks. Primarily four types of network architectures in deep learning are identified and discussed in this work. They are unsupervised pre-trained network, convolution neural network, recurrent neural network and recursive neural network architectures. Types and availability of general training datasets are discussed wherein deep learning models can be trained and benchmarked. Unavailability of extensive training datasets for the specific task application is a major hurdle in implementing deep learning methods. Performance of these deep learning methods is also debated in this work. The potential research directions are thus proposed in the paper.
MoreTranslated text
Key words
Robotic grasping, Deep learning, Convolution neural network, Reinforcement learning
AI Read Science
Must-Reading Tree
Example
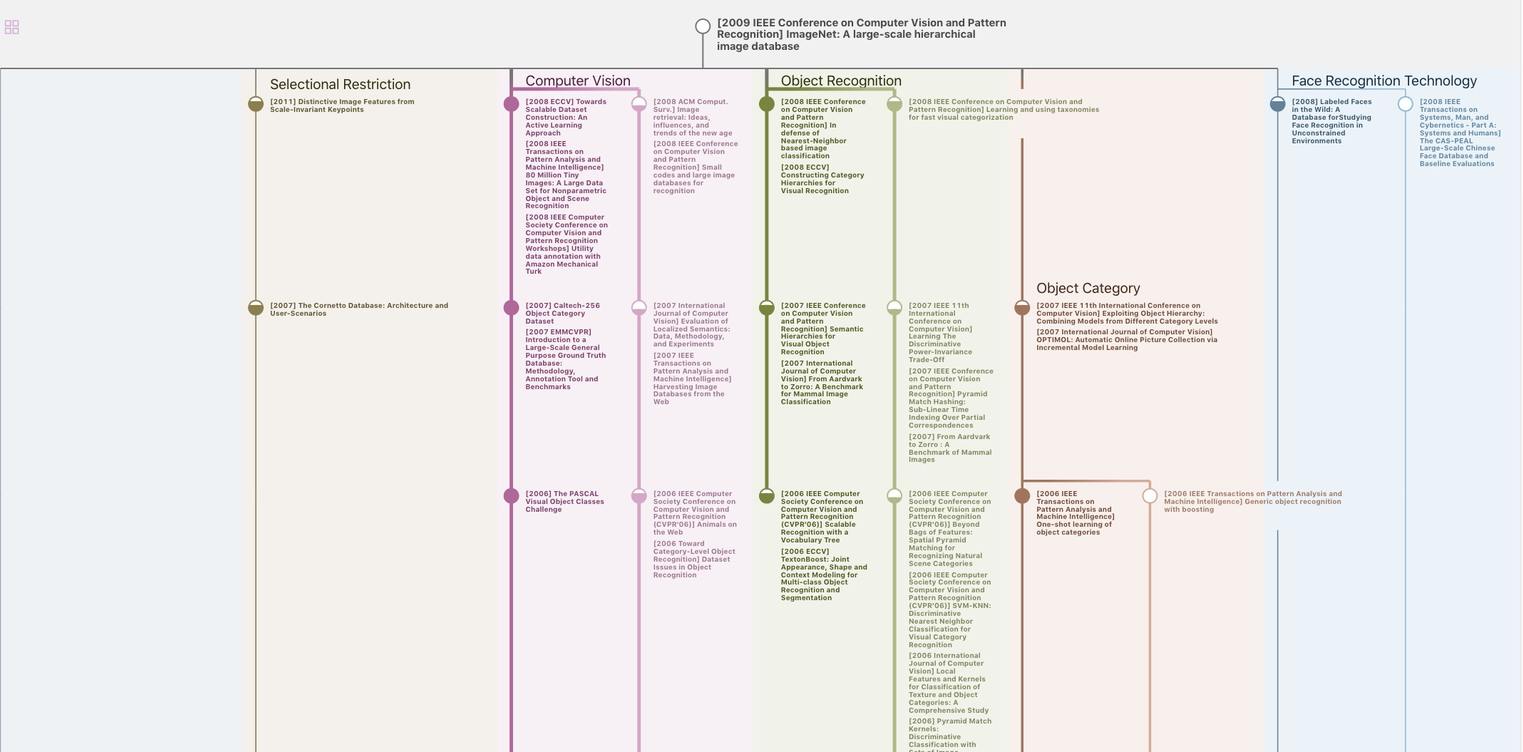
Generate MRT to find the research sequence of this paper
Chat Paper
Summary is being generated by the instructions you defined