Fleet-Oriented Pattern Mining Combined with Time Series Signature Extraction for Understanding of Wind Farm Response to Storm Conditions
Lecture Notes in Mechanical EngineeringAdvances in Condition Monitoring and Structural Health Monitoring(2021)
摘要
Offshore wind turbine installations are rapidly spreading around Europe and all over the world. These turbines are typically installed in large wind farms combining turbines of the same type. Farm owners target maximal performance of the farm in general and particularly predictability of behaviour. The latter is getting increasingly important since offshore wind farms are being managed more and more as conventional power plants driven by the electricity market supply and demand considerations. The context of zero subsidy farms exposes farm operators to fluctuations in electricity market prices. As such, deep understanding of farm behaviour is essential to come up with a good strategy to deal with these fluctuations. This paper focusses on the automated extraction of farm-wide response to storm conditions. The input data for the analysis are status logs and SCADA 1-second data. The status logs record the important turbine controller events. Typically, they consist of a number, a time of occurrence, and a time of de-activation. The number is linked to a detailed description. The SCADA data consists of time series of the most important sensors in the turbine: power produced, RPM, wind speed,… The advantage of the 1-sec data over the traditional 10-minute averages is that the dynamic event content is much more preserved. Data of several offshore wind farms is used in the analysis to have a solid dataset. In total, 5 years of data of more than 50 turbines is used. We show a novel farm-wide pattern mining approach that extracts events occurring for multiple turbines in the same time period. This allows us to identify those events that are predominantly driven by global wind excitations (e.g., gusts) or grid events (e.g., low voltage ride through). From the extracted events, we lift out the storm conditions. For these conditions, a further investigation of the time series data is done. Using event detection algorithms, we extract the signatures of the stop events that each turbine is performing from the time series data. We show that the extreme change in wind speed and wind direction leads to an excessive misalignment of the turbines in the farm, followed by a stop of those turbines. The extracted patterns are compared to the time signatures to show their correlation and complementarity. As such, the typical turbine response to this event is identified. This can serve as input for identification of novel controller approaches by the farm owner and turbine manufacturer to deal with this problem.
更多查看译文
关键词
Pattern mining, Condition monitoring, Wind turbine, Dynamic event, Fleet, Wind farm
AI 理解论文
溯源树
样例
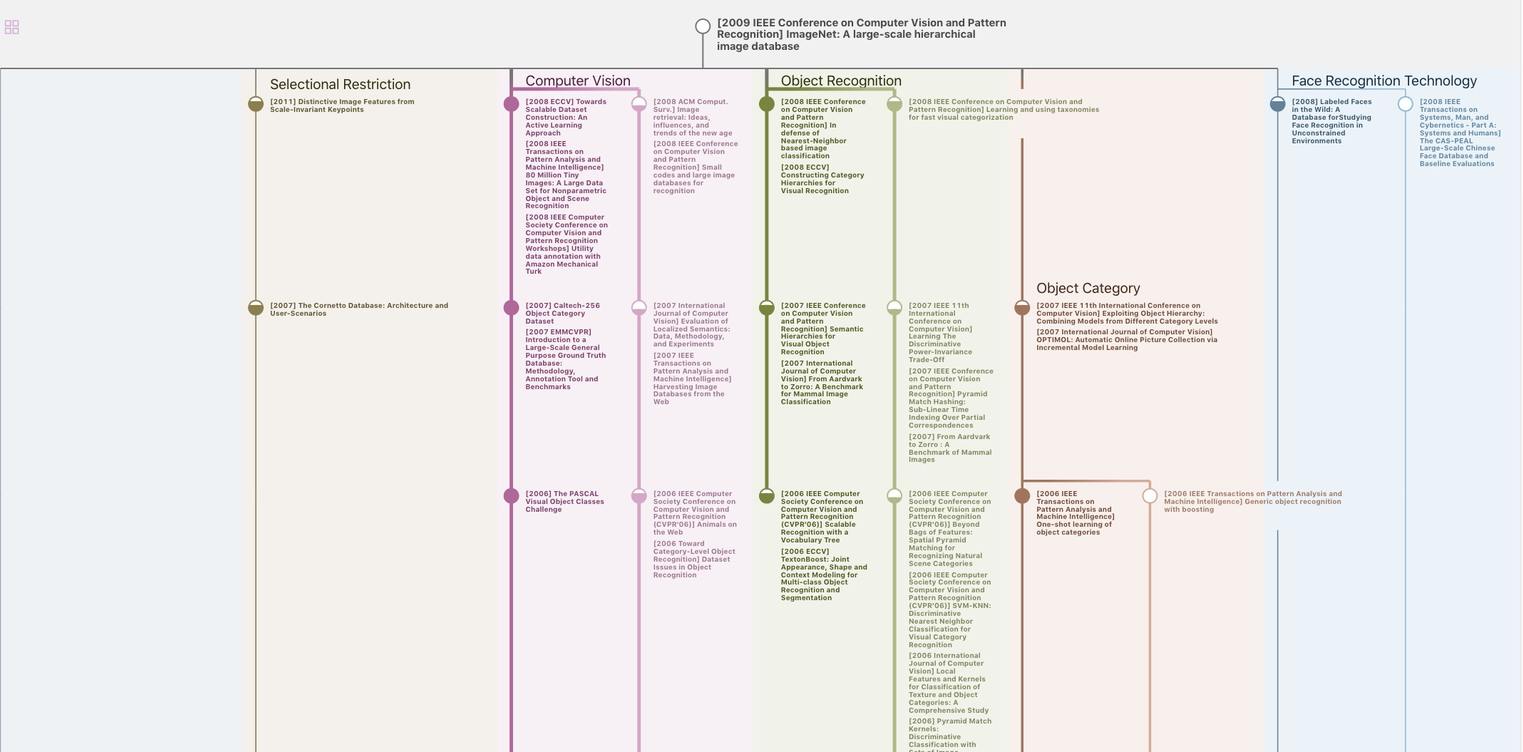
生成溯源树,研究论文发展脉络
Chat Paper
正在生成论文摘要