Comparison of Complexity Measures for Structural Health Monitoring
Model Validation and Uncertainty Quantification, Volume 3Conference Proceedings of the Society for Experimental Mechanics Series(2020)
摘要
The field of structural health monitoring (SHM) applies damage detection techniques to provide timely in-situ system condition assessment. Previously, researchers have suggested a fundamental axiom for SHM that states, “damage will increase the complexity of a system.” One way this increased complexity can manifest itself is in the increased complexity of sensor data recorded from the structure when damage occurs. The question then becomes how to best quantify the increase in complexity of those data. Information complexity is one such approach and within this framework various information entropy quantities have been proposed as measures of complexity. The literature has shown that there are multiple information entropy measures, including; Shannon Entropy, Rényi Entropy, Permutation Entropy, Sample Entropy, Approximate Entropy, and Spectral Entropy. With multiple measures proposed to quantify information entropy; a study to compare the relative effectiveness of these entropy measures in the context of SHM is needed. Therefore, the objective of this paper is to compare the effectiveness of entropy-based methods in distinguishing between “Healthy” and “Unhealthy” labeled datasets. The labeled datasets considered in this study were obtained from a 4DOF impact oscillator, a rotating machine with a damaged bearing, and an impact oscillator excited by a rotating machine. Furthermore, two methods were used in this study to classify the results from the different entropy measures; Naïve-Bayes classification, and K-means clustering. Effectiveness of a given entropy measure is determined by the number of misclassifications produced when compared to the true labels. The analysis showed that entropy measures obtained from data corresponding to sensors closer to the damage source had fewer misclassifications for the datasets tested. For the datasets considered in this study, the researchers concluded that each dataset had a different most effective entropy measure. The study would need to be expanded to include other classification methods and other datasets to define more precisely which entropy measure is the most effective in identifying the increase in complexity associated with damage and, hence, distinguishing between healthy and damaged data.
更多查看译文
关键词
Structural health monitoring, Vibration, Entropy methods, Damage detection, Thresholding, Rotating machinery, Structural dynamics
AI 理解论文
溯源树
样例
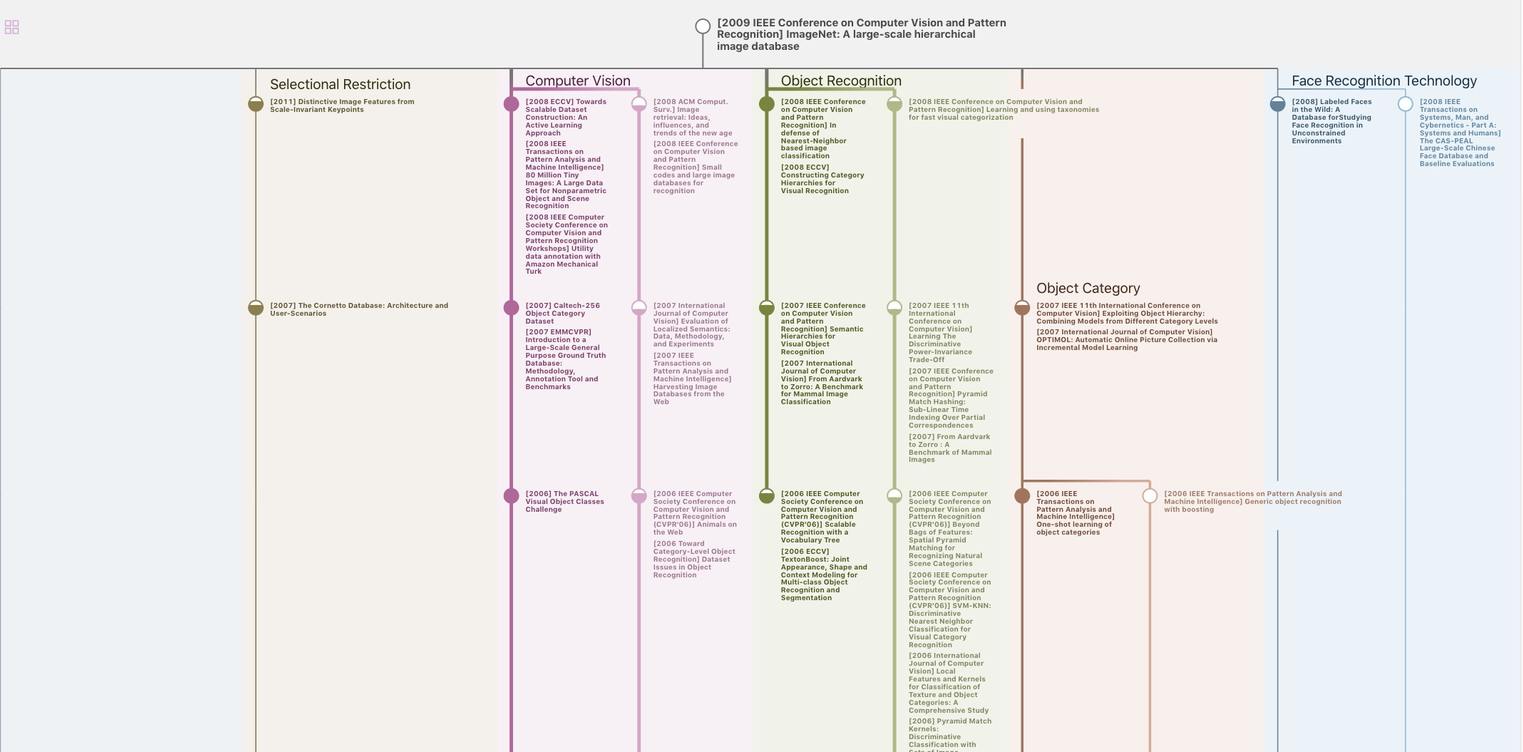
生成溯源树,研究论文发展脉络
Chat Paper
正在生成论文摘要