Adapting Machine Learning Techniques for Credit Card Fraud Detection
springer
摘要
Credit card fraud is a form of financial frauds growing every year and causing losses to financial multinationals as well as government sector. Traditional methods like manually detecting credit card frauds are feasible only for small datasets, but with the rise of big data, these methods are of no worth, hence has been proved as an unrealistic and time-consuming solution. Therefore, the finance industry is looking for help from data mining and machine learning methodologies in order to handle problems. But constructing rule for fraud detection algorithms is difficult because of imbalance nature of credit card dataset. Several challenges while mining credit card dataset with the experimental solution are presented through this paper. Undersampling and SMOTE oversampling techniques are applied to balance the real-time credit card transaction dataset, and then several supervised machine learning algorithms are compared to classify credit card frauds precisely. Credit card fraud detection model using logistic regression is pragmatic as the best classifier when compare to decision tree, support vector machine, and K-nearest neighbor. Confusion matrix and several other matrices are used during experiments to evaluate the performances of the credit card fraud detection model.
更多查看译文
关键词
Machine learning, Data mining, Credit card fraud, Big data, Finance industry
AI 理解论文
溯源树
样例
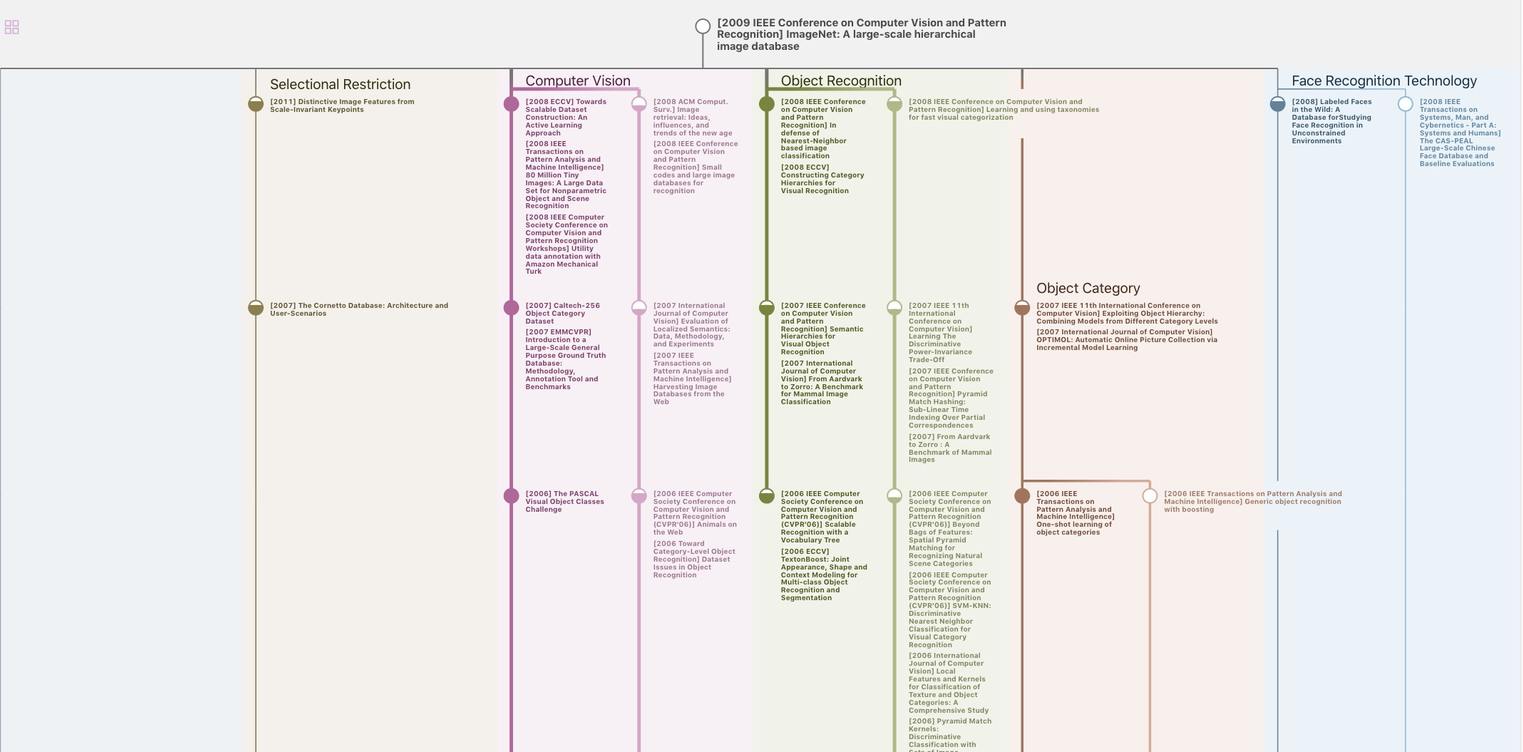
生成溯源树,研究论文发展脉络
Chat Paper
正在生成论文摘要