Comparison of Classification Methods for Spectral Data of Laser-Induced Fluorescence
Enhancing CBRNE Safety & Security Proceedings of the SICC 2017 Conference(2018)
摘要
Online detection of CBRNE is a research field of growing importance due to its relevance for public security and defense. The selectivity of machine learning has reached maturity in order to distinguish very similar laser-induced fluorescence (LIF) spectra of different samples—establishing the basis for an automatic classification. The work in this contribution applies the classification process of decision trees, support vector machines, and artificial neural networks to LIF spectra. Two experimental setups with two excitation wavelengths each (280 and 355 nm; 266 and 355 nm) and different spectral resolutions of about 1 nm and 12 nm, respectively, have been performed. In the first setup, the discrimination of seven bacteria species with an accuracy of over 90% is demonstrated. The data of the second setup with lower spectral resolution are equally sufficient for a subsequent classification. The results are compared and represented in a low-dimensional subspace for the purpose of visualization.
更多查看译文
关键词
Standoff detection, Laser-induced fluorescence spectra, Classification models, Machine learning, Decision trees, Support vector machines, Artificial neural networks
AI 理解论文
溯源树
样例
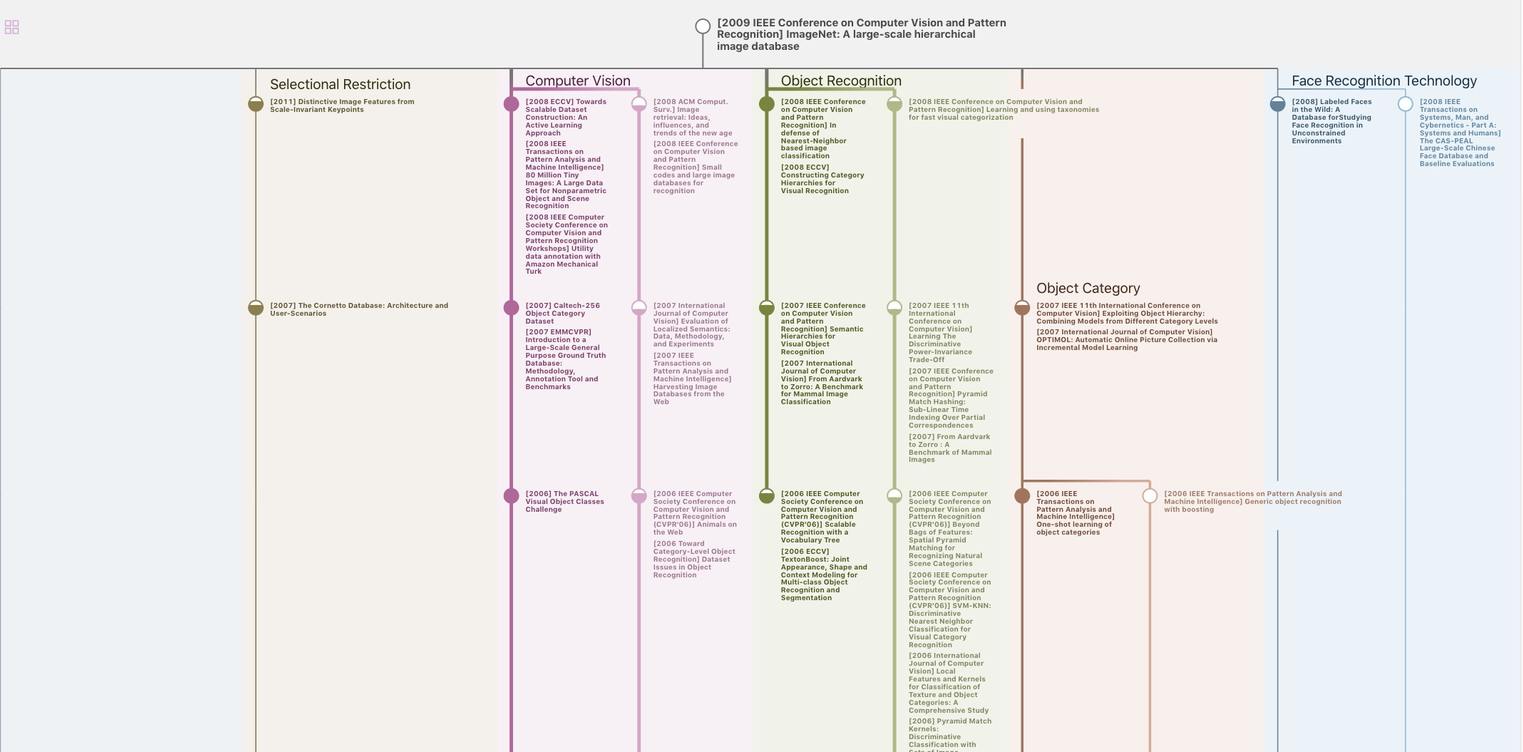
生成溯源树,研究论文发展脉络
Chat Paper
正在生成论文摘要