Performance Evaluation of BPSO & PCA as Feature Reduction Techniques for Bearing Fault Diagnosis
Lecture Notes in Electrical EngineeringRecent Trends in Mechatronics Towards Industry 40(2021)
摘要
Vibration-based signal processing is the most popular and effective approach for fault diagnosis of bearing. In this paper, time-frequency domain analysis, i.e. empirical mode decomposition (EMD) was applied to the raw vibration signal. Intrinsic mode function (IMF) containing the characteristics of vibration data was analysed to obtain 90 statistical features. Two feature reduction algorithms, namely principal components analysis (PCA) and binary particle swarm optimiser (BPSO) were applied individually for feature reduction. The reduced feature subsets were 12 and 35 for PCA and BPSO, respectively. K-Nearest Neighbours (K-NN) was used as an intelligent method for fault diagnosis. K-NN was applied to the entire feature set and individually on the selected feature subset of PCA and BPSO. The reduced feature subset with PCA performed the finest in all the measurements taken. For BPSO, although it effectively reduced the feature dimension and classification time, the testing accuracy was slightly lower. Comparing the output accuracy of the K-NN classifier for the selected methods demonstrated the effectiveness of PCA and BPSO as efficacious feature reduction techniques.
更多查看译文
关键词
Feature reduction, Principal components analysis, Binary particle swarm optimiser, Empirical mode decomposition
AI 理解论文
溯源树
样例
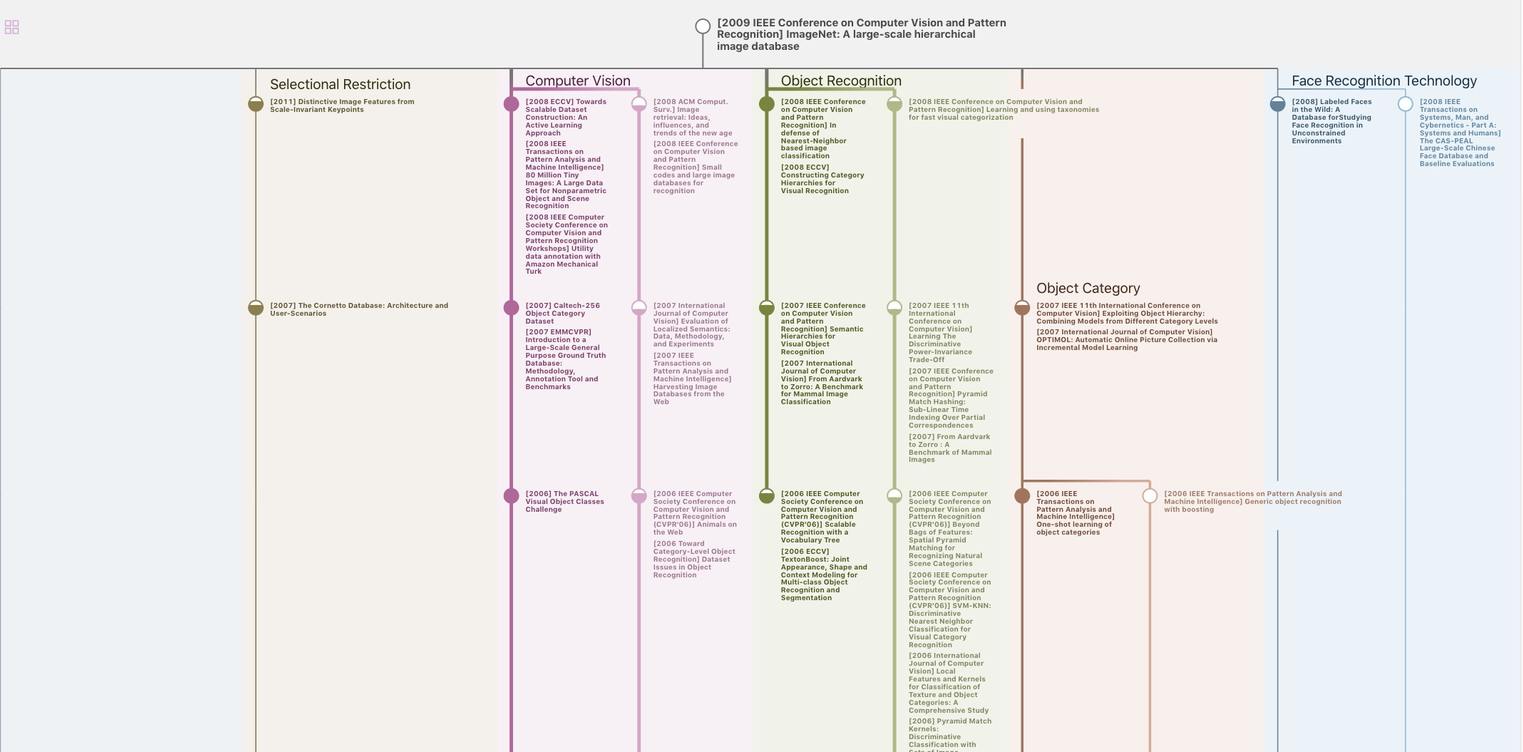
生成溯源树,研究论文发展脉络
Chat Paper
正在生成论文摘要