Ensemble of Multi-time Resolution Recurrent Neural Networks for Enhanced Feature Extraction in High-Rate Time Series
Conference proceedings of the Society for Experimental Mechanics(2021)
摘要
Systems experiencing high-rate dynamic events, termed high-rate systems, typically undergo accelerations of amplitudes higher than 100 g in less than 10 ms. Examples include adaptive airbag deployment systems, hypersonic vehicles, and active blast mitigation systems. Given the critical functions of such systems, accurate and fast modeling tools are necessary for ensuring the target performance. However, the unique characteristics of these systems, which consist of (1) large uncertainties in the external loads, (2) high levels of nonstationarities and heavy disturbances, and (3) unmodeled dynamics generated from changes in system configurations, in combination with the fast-changing environment, limit the applicability of physical modeling tools. In this chapter, a neural network-based approach is proposed to model and predict high-rate systems. It consists of an ensemble of recurrent neural networks (RNNs) with short-sequence long short-term memory (LSTM) cells which are concurrently trained. To empower multi-step-ahead predictions, the input space for each RNN is selected individually using principal component analysis that extracts different resolutions on the dynamics. The proposed algorithm is validated on experimental data obtained from a high-rate system. Results showed that this algorithm significantly improves the quality of step-ahead predictions over a heuristic approach in constructing the input spaces.
更多查看译文
关键词
Recurrent neural network, Long short-term memory, Prediction, High rate, Nonstationary, Time series
AI 理解论文
溯源树
样例
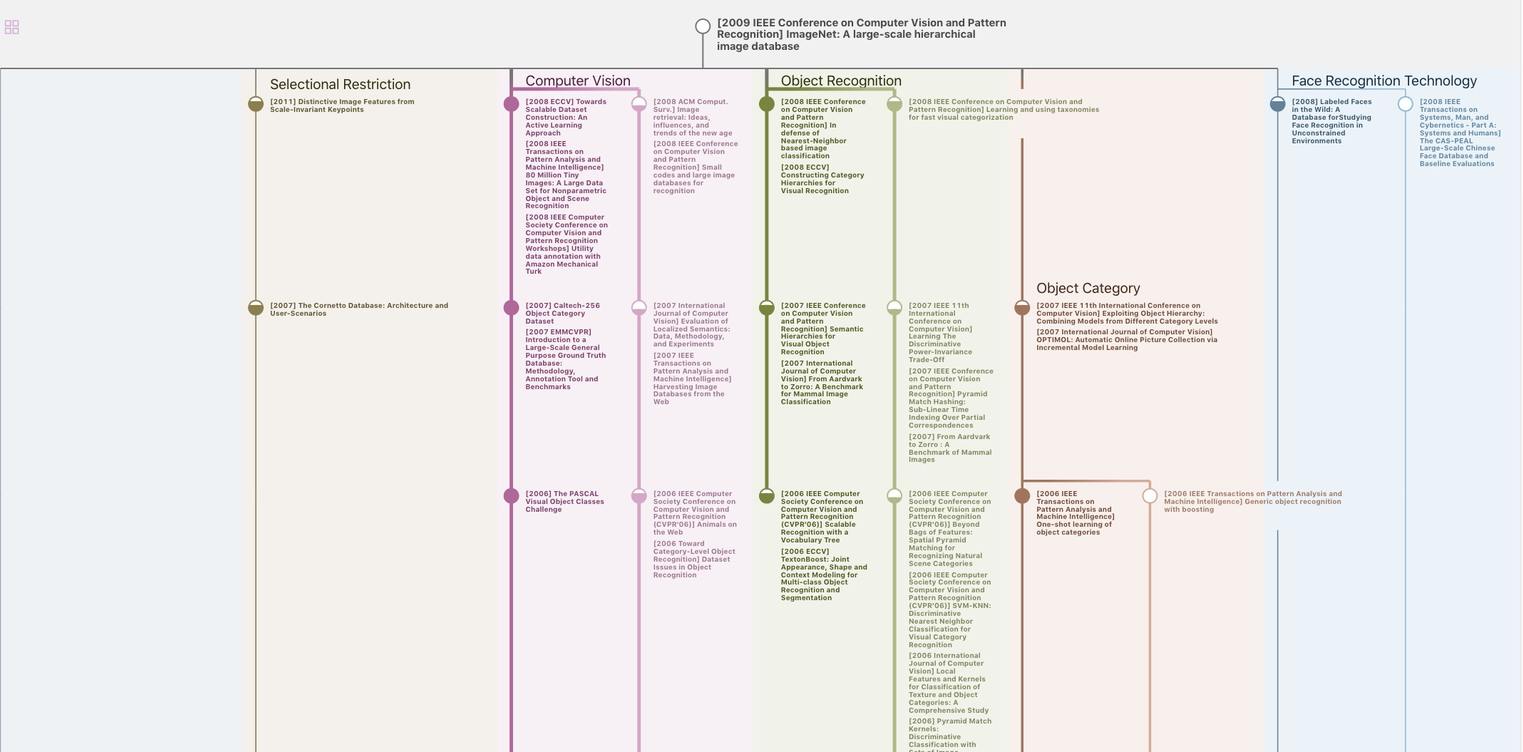
生成溯源树,研究论文发展脉络
Chat Paper
正在生成论文摘要