Optimal Transport Based Multi-layer Domain Adaptation Model for Industrial Fault Diagnosis
Lecture Notes in Electrical EngineeringProceedings of 2021 Chinese Intelligent Systems Conference(2021)
Abstract
Bearing and gearbox are important parts of transmission system, which can directly affect the efficiency of the industrial system. However, due to the varying working load and environmental change, the training and test data can not follow the same distribution, causing that the performance of traditional machine learning methods is significantly reduced. In this paper, A multi-layer optimal transport adaptation network (MLOT) is proposed. Firstly, the stacked autoencoder is trained layer by layer to extract the abstract information hidden in the data. Secondly, a Wasserstein distance is embedded into the autoencoder to reduce the discrepancies between the source and target features, which can improve the generalization of the model in the target domain. Finally, the label predictor (Softmax) trained on source domain can be directly applied in the target domain and reduce the cost of recollecting annotation data. The experimental results show that the proposed algorithm has superior domain adaptation learning ability and fault diagnosis effect.
MoreTranslated text
Key words
Domain adaptation, Optimal transport, Stacked autoencoder, Wasserstein distance
AI Read Science
Must-Reading Tree
Example
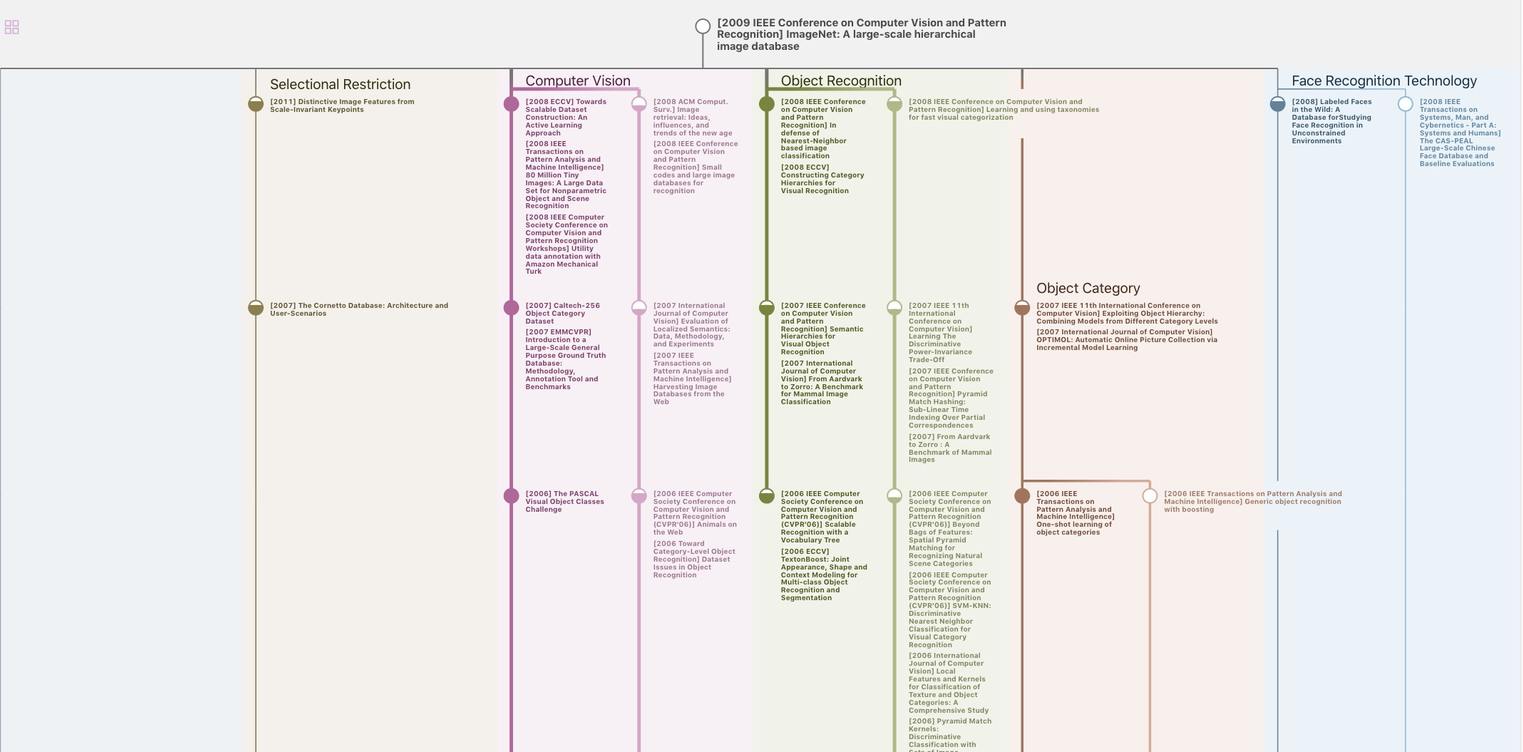
Generate MRT to find the research sequence of this paper
Chat Paper
Summary is being generated by the instructions you defined