RestainNet: a self-supervised digital re-stainer for stain normalization
COMPUTERS & ELECTRICAL ENGINEERING(2022)
摘要
Color inconsistency is an inevitable challenge in computational pathology, which generally happens because of stain intensity variations or sections scanned by different scanners. It harms the pathological image analysis methods, especially the learning-based models. A series of approaches have been proposed for stain normalization. However, most of them are lack flexibility in practice. In this paper, we formulated stain normalization as a digital re-staining process and proposed a self-supervised learning model, which is called RestainNet. Our network is regarded as a digital restainer which learns how to re-stain an unstained (grayscale) image. Two digital stains, Hematoxylin (H) and Eosin (E) were extracted from the original image by Beer-Lambert's Law. We proposed a staining loss to maintain the correctness of stain intensity during the restaining process. Thanks to the self-supervised nature, paired training samples are no longer necessary, which demonstrates great flexibility in practical usage. Our RestainNet outperforms existing approaches and achieves state-of-the-art performance with regard to color correctness and structure preservation. We further conducted experiments on the segmentation and classification tasks and the proposed RestainNet achieved outstanding performance compared with SOTA methods. The self-supervised design allows the network to learn any staining style with no extra effort.
更多查看译文
关键词
Computational pathology,Stain normalization,Self-supervised learning,Generative adversarial networks,Whole slide images
AI 理解论文
溯源树
样例
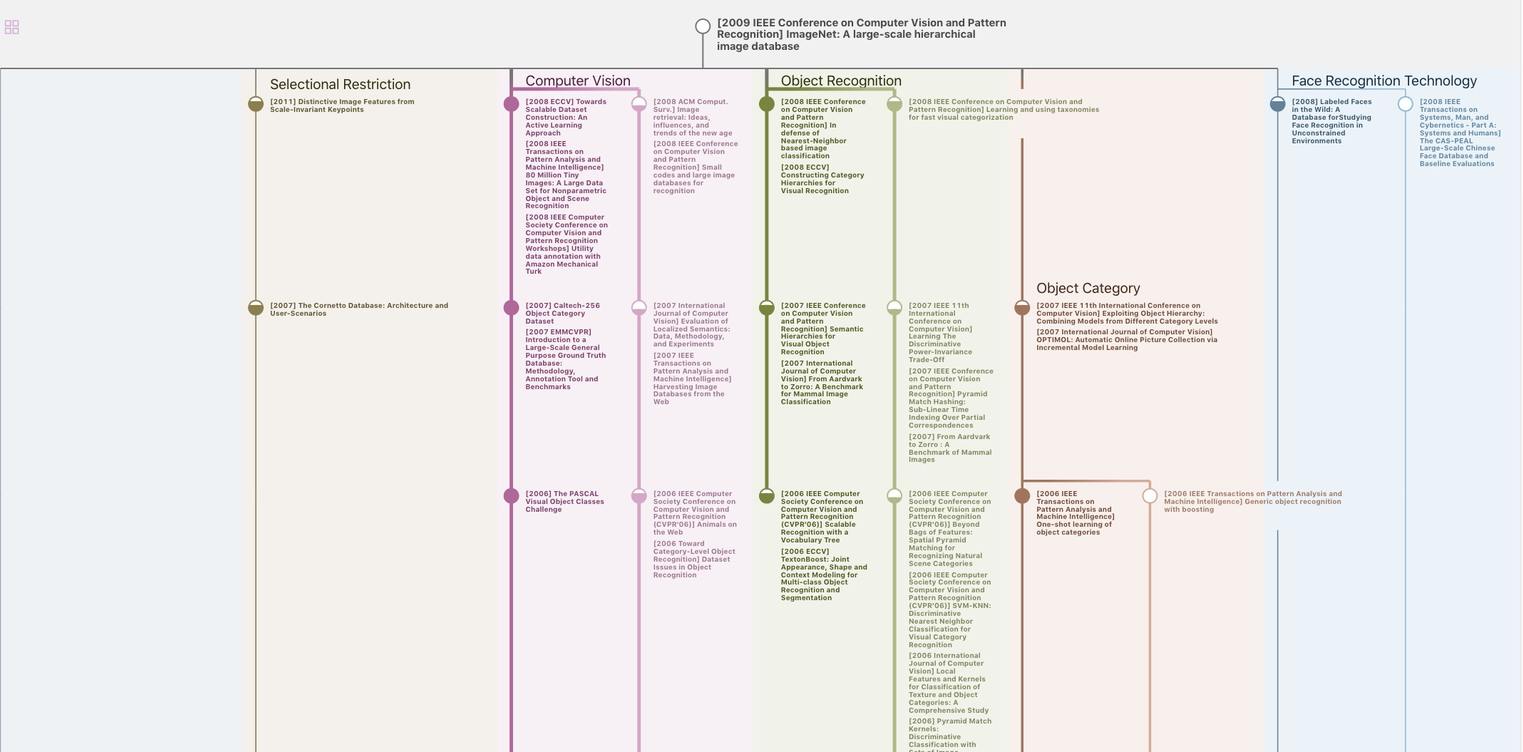
生成溯源树,研究论文发展脉络
Chat Paper
正在生成论文摘要