Drug Properties Prediction Based on Deep Learning
Pharmaceutics(2022)
摘要
In recent research on the formulation prediction of oral dissolving drugs, deep learning models with significantly improved performance compared to machine learning models were proposed. However, the performance degradation due to limitations of an imbalanced dataset with a small size and inefficient neural network structure has still not been resolved. Therefore, we propose new deep learning-based prediction models that maximize the prediction performance for disintegration time of oral fast disintegrating films (OFDF) and cumulative dissolution profiles of sustained-release matrix tablets (SRMT). In the case of OFDF, we use principal component analysis (PCA) to reduce the dimensionality of the dataset, thereby improving the prediction performance and reducing the training time. In the case of SRMT, the Wasserstein generative adversarial network (WGAN), a neural network-based generative model, is used to overcome the limitation of performance improvement due to the lack of experimental data. To the best of our knowledge, this is the first work that utilizes WGAN for pharmaceutical formulation prediction. Experimental results show that the proposed methods have superior performance than the existing methods for all the performance metrics considered.
更多查看译文
关键词
deep learning,imbalanced data,small data,principal component analysis,Wasserstein GAN,pharmaceutical formulation
AI 理解论文
溯源树
样例
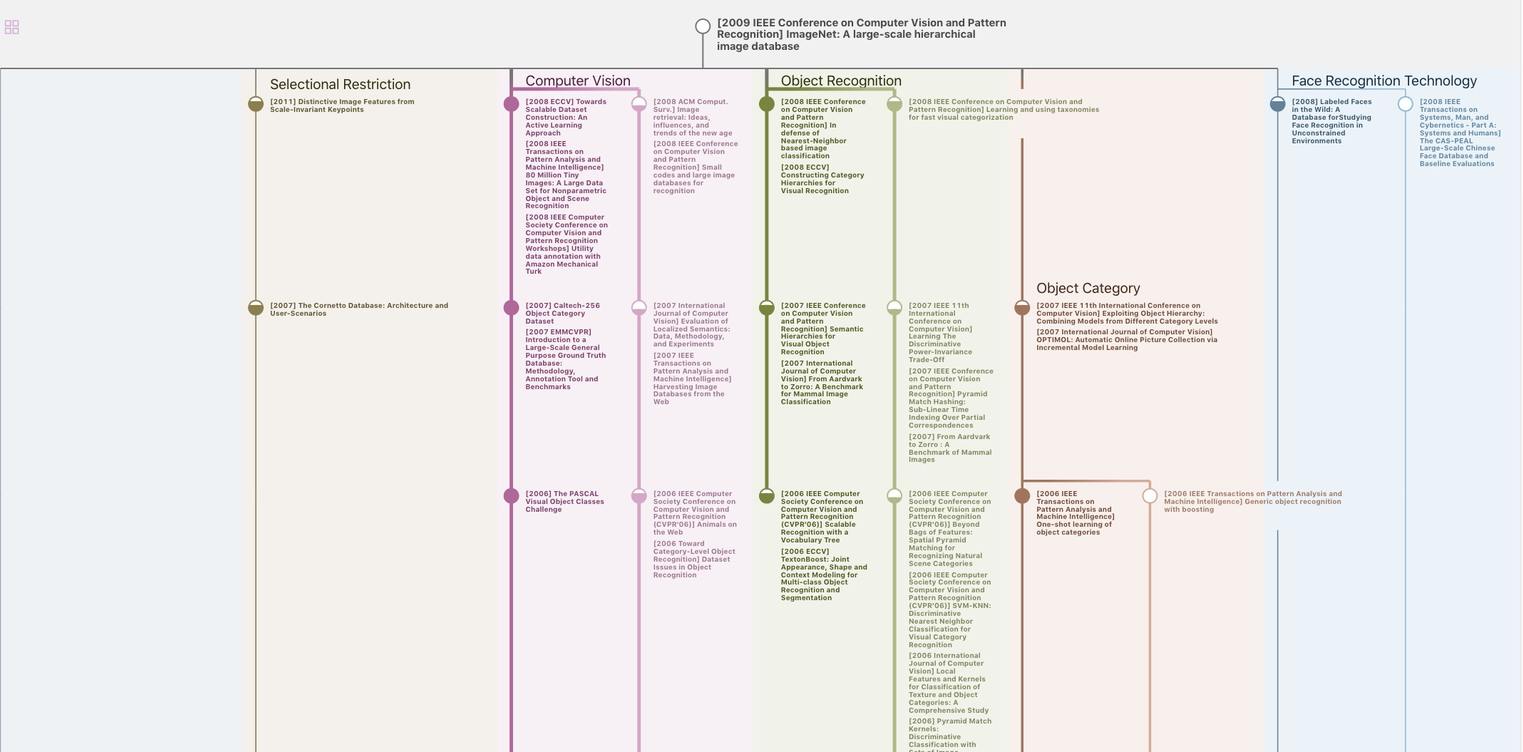
生成溯源树,研究论文发展脉络
Chat Paper
正在生成论文摘要