Quantitative Analysis of Radiation-Associated Parenchymal Lung Change
CANCERS(2022)
Abstract
Simple Summary Radiotherapy is commonly used to treat inoperable locally advanced lung cancer. Despite the use of sophisticated modern planning and imaging techniques to target the tumour and minimise dose to normal lung tissue, patients can suffer from acute and chronic respiratory problems after treatment. Currently, our understanding of the impact that radiotherapy has on patients' lungs is inadequate. We have, therefore, proposed a novel classification of the damage to the lung tissue, as seen on CT scans after a course of radiotherapy to a lung tumour. We have used deep learning algorithms to allow large numbers of CT scans to be labelled at the level of the individual voxel according to the degree of damage. The dose delivered to the tumour and the change in lung function of the patient after treatment both correlated well to the degree of radiological change measured. Our novel, automated classification combined with a dedicated image registration method has demonstrated an important clinical application that could be used to improve radiotherapy delivery in the future by allowing us to precisely track the changes seen after radiation treatment. We present a novel classification system of the parenchymal features of radiation-induced lung damage (RILD). We developed a deep learning network to automate the delineation of five classes of parenchymal textures. We quantify the volumetric change in classes after radiotherapy in order to allow detailed, quantitative descriptions of the evolution of lung parenchyma up to 24 months after RT, and correlate these with radiotherapy dose and respiratory outcomes. Diagnostic CTs were available pre-RT, and at 3, 6, 12 and 24 months post-RT, for 46 subjects enrolled in a clinical trial of chemoradiotherapy for non-small cell lung cancer. All 230 CT scans were segmented using our network. The five parenchymal classes showed distinct temporal patterns. Moderate correlation was seen between change in tissue class volume and clinical and dosimetric parameters, e.g., the Pearson correlation coefficient was <= 0.49 between V30 and change in Class 2, and was 0.39 between change in Class 1 and decline in FVC. The effect of the local dose on tissue class revealed a strong dose-dependent relationship. Respiratory function measured by spirometry and MRC dyspnoea scores after radiotherapy correlated with the measured radiological RILD. We demonstrate the potential of using our approach to analyse and understand the morphological and functional evolution of RILD in greater detail than previously possible.
MoreTranslated text
Key words
radiotherapy-induced lung damage, lung cancer, deep learning
AI Read Science
Must-Reading Tree
Example
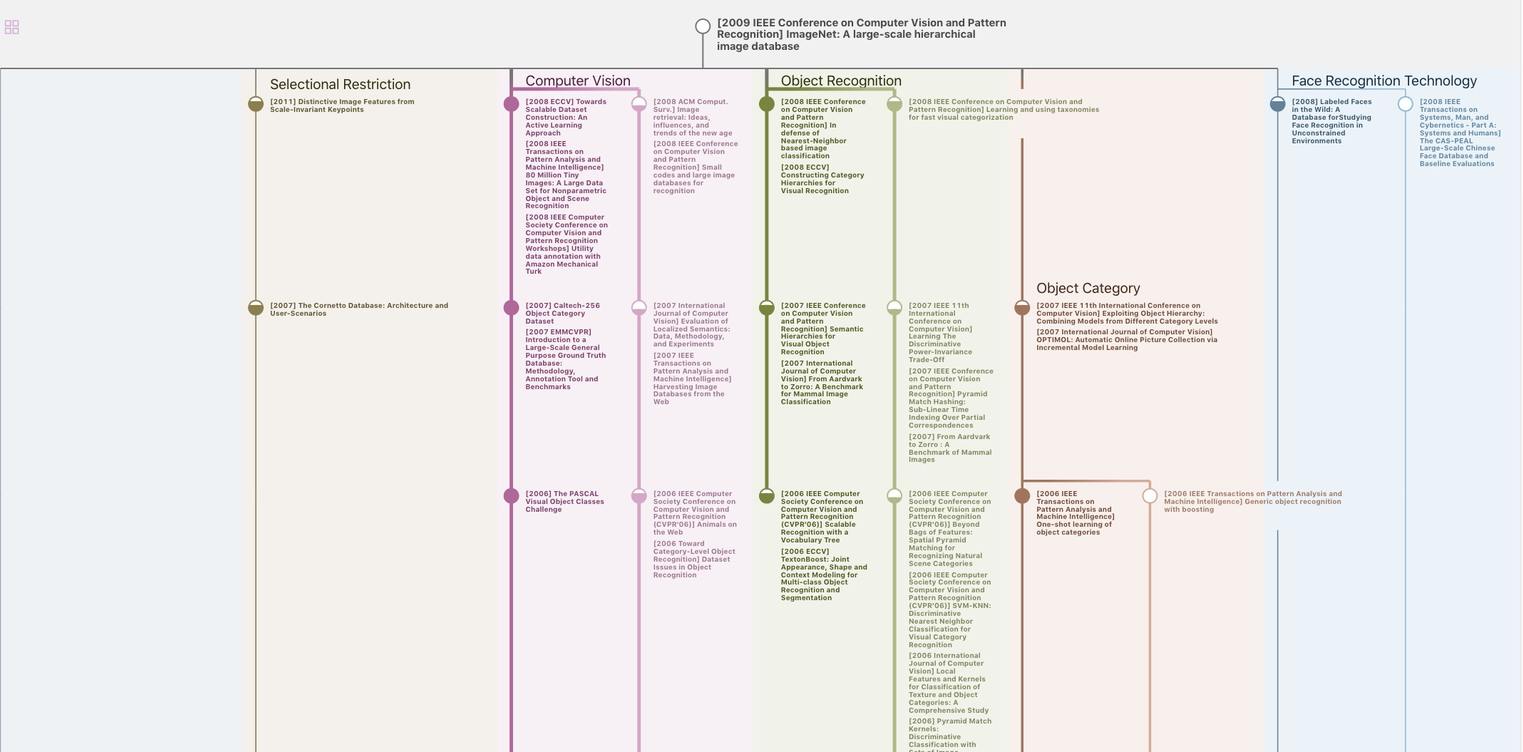
Generate MRT to find the research sequence of this paper
Chat Paper
Summary is being generated by the instructions you defined