Feature Selection and Classification of Leukemia Cancer Using Machine Learning Techniques
Machine Learning Research(2020)
Abstract
Leukemia cancer is one of the most leading detrimental cancer diseases in worldwide. A huge number of genes are responsible for cancer diseases. Therefore, it is necessary to identify the most informative genes of Leukemia cancer. The main objectives of this study are to: (i) identify the most informative genes using five feature selection techniques (FST) and (ii) adopt six classifiers to classify the cancer disease and compare them. Leukemia cancer data has been taken from Kent ridge biomedical data repository, USA. There are 7129 genes and 72 patients. Among them, 47 patients are cancer and 25 are control. We have used five FST as t-test; Wilcoxon sign rank sum (WCSRS) test, random forest (RF), Boruta and least absolute shrinkage and selection operator (LASSO). We have also used six classifiers as Adaboost (AB), classification and regression tree (CART), artificial neural network (ANN), random forest (RF), linear discriminant analysis (LDA) and naive Bayes (NB). The performances of these classifiers are evaluated by accuracy (ACC), sensitivity (SE), specificity (SP), positive predictive value (PPV), negative predictive value (NPV), and F-measure (FM). We used simulated dataset to check the validity of proposed method. The results indicate that the combination of LASSO based FST and NB classifier gives the highest classification accuracy of 99.95%. On the basis of the results, we can conclude that the combination of LASSO based FST and NB classifier predicts the leukemia cancer more accurately compare to any other combination of FST and classifiers utilized in this study.
MoreTranslated text
Key words
feature selection,machine learning techniques,leukemia cancer,machine learning,classification
AI Read Science
Must-Reading Tree
Example
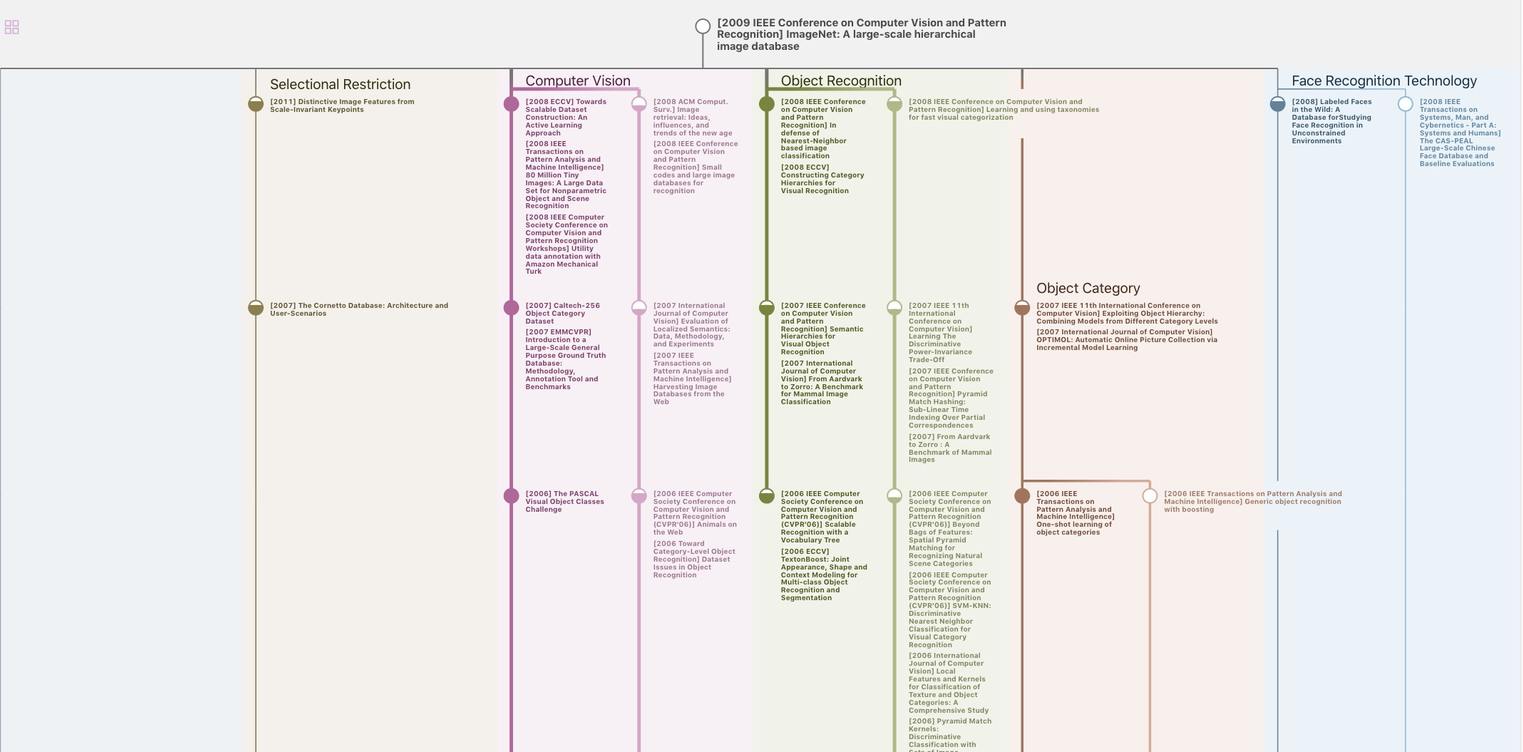
Generate MRT to find the research sequence of this paper
Chat Paper
Summary is being generated by the instructions you defined