Overcoming Limitations in Evaluation of Memristor Technologies for Neural Networks
ECS Meeting Abstracts(2020)
摘要
Intrinsic switching characteristics of two resistive memory technologies, hafnia (RRAM)- and carbon nanotube (CNT)- based, are evaluated with respect to their implementation in deep neural networks (DNN) and possible mitigation approaches to performance degradation. Both RRAM (poly-crystalline metal oxide) and NRAM (fabric of interlocking matrix of CNTs) operations employ modulations of the conductive paths through a semi-isolating layer sandwiched between electrodes. RRAM and NRAM characteristics demonstrate similar features when evaluated employing commonly used switching times > 100 ns: variability of the weights update values linked to stochasticity of the switching processes, asymmetric weight change, and memory update saturation. Though materials are different, the observed undesirable trends have a common cause that is excessive energy released within the duration of switching operations: it induces gradual changes in the material structure surrounding conductive paths. Such changes, in turn, affect temperature distribution and related structural rearrangements during the subsequent switching. In particular, memory update saturation reflects on exhaustion of the number of structural blocks (atoms, CNTs) available for expanding the conductive path under specific used operation conditions. As follows, by reducing energy released in each operation we may suppress excessive structural changes, hence related performance degradation features. Indeed, under sub-ns switching conditions relevant to high-frequency circuitry operations, both RRAM and NRAM demonstrate significant performance improvement making them promising for DNN implementations.
更多查看译文
AI 理解论文
溯源树
样例
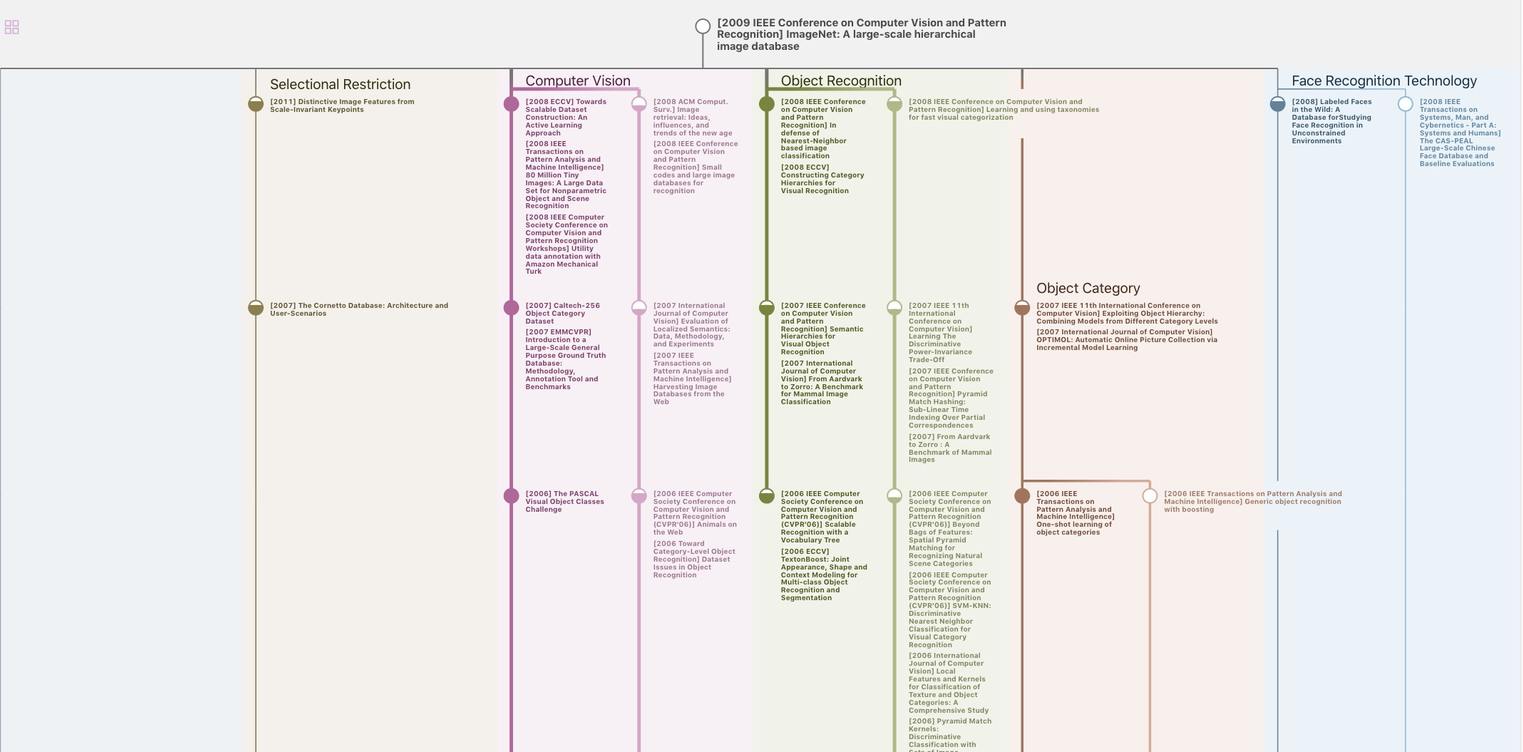
生成溯源树,研究论文发展脉络
Chat Paper
正在生成论文摘要