A Layered Recurrent Neural Network for Imputing Air Pollutants Missing Data and Prediction of NO2, O3, PM10, and PM2.5
Forecasting in Mathematics - Recent Advances, New Perspectives and Applications(2021)
摘要
To fulfill the national air quality standards, many countries have created emissions monitoring strategies on air quality. Nowadays, policymakers and air quality executives depend on scientific computation and prediction models to monitor that cause air pollution, especially in industrial cities. Air pollution is considered one of the primary problems that could cause many human health problems such as asthma, damage to lungs, and even death. In this study, we present investigated development forecasting models for air pollutant attributes including Particulate Matters (PM2.5, PM10), ground-level Ozone (O3), and Nitrogen Oxides (NO2). The dataset used was collected from Dubrovnik city, which is located in the east of Croatia. The collected data has missing values. Therefore, we suggested the use of a Layered Recurrent Neural Network (L-RNN) to impute the missing value(s) of air pollutant attributes then build forecasting models. We adopted four regression models to forecast air pollutant attributes, which are: Multiple Linear Regression (MLR), Decision Tree Regression (DTR), Artificial Neural Network (ANN) and L-RNN. The obtained results show that the proposed method enhances the overall performance of other forecasting models.
更多查看译文
关键词
air pollutants missing data,layered recurrent neural network,neural network,prediction
AI 理解论文
溯源树
样例
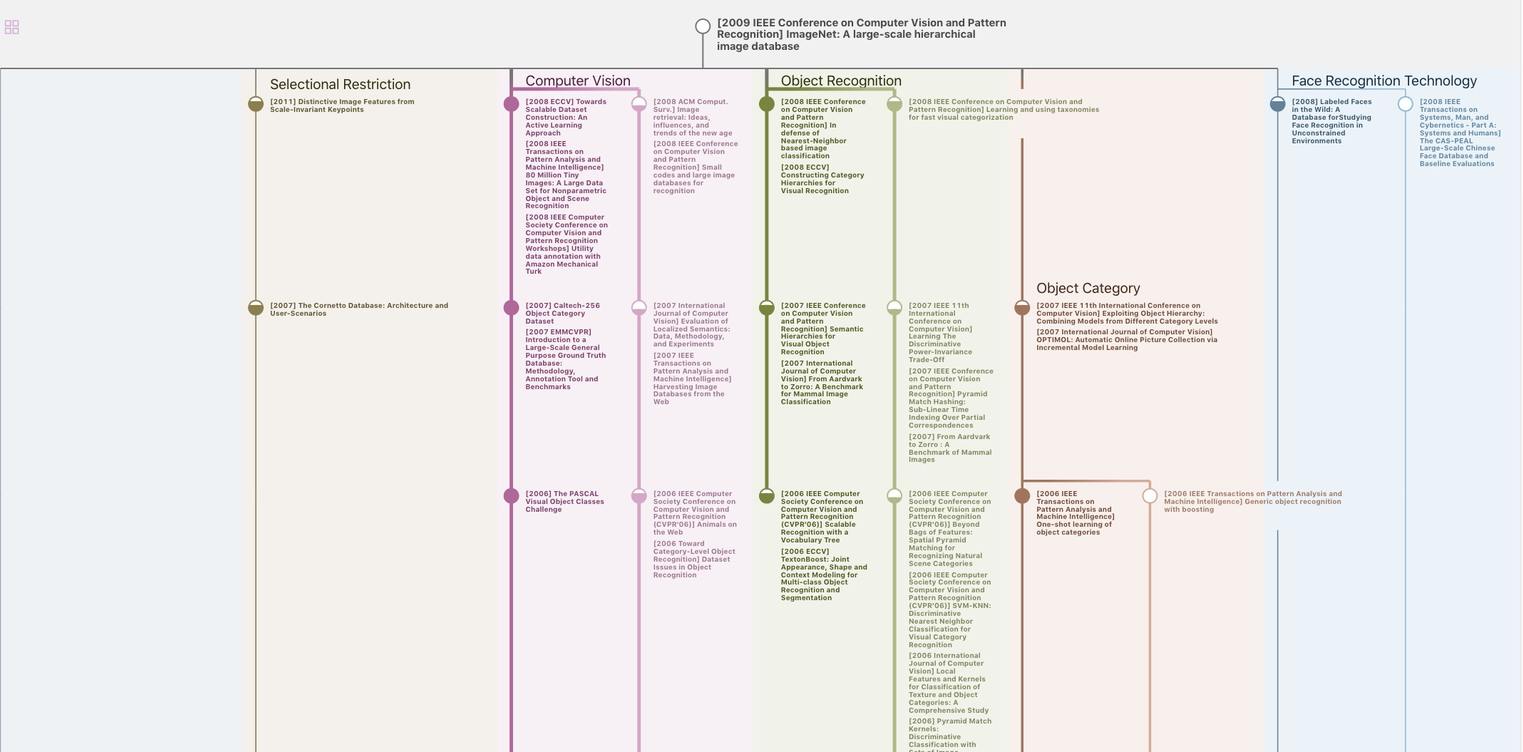
生成溯源树,研究论文发展脉络
Chat Paper
正在生成论文摘要