Organizational Policy Learning and Evaluation Using Monte Carlo Methods
Encyclopedia of Organizational Knowledge, Administration, and TechnologyAdvances in Logistics, Operations, and Management Science(2021)
摘要
Organizations need to constantly learn, develop, and evaluate new strategies and policies for their effective operation. Unsupervised reinforcement learning is becoming a highly useful tool, since rewards and punishments in different forms are pervasive and present in a wide variety of decision-making scenarios. By observing the outcome of a sufficient number of repeated trials, one would gradually learn the value and usefulness of a particular policy or strategy. However, in a given environment, the outcomes resulting from different trials are subject to external chance influence and variations. In learning about the usefulness of a given policy, significant costs are involved in systematically undertaking the sequential trials; therefore, in most learning episodes, one would wish to keep the cost within bounds by adopting learning efficient stopping rules. In this Chapter, we explain the deployment of different learning strategies in given environments for reinforcement learning policy evaluation and review, and we present suggestions for their practical use and applications.
更多查看译文
关键词
organizational policy learning,evaluation
AI 理解论文
溯源树
样例
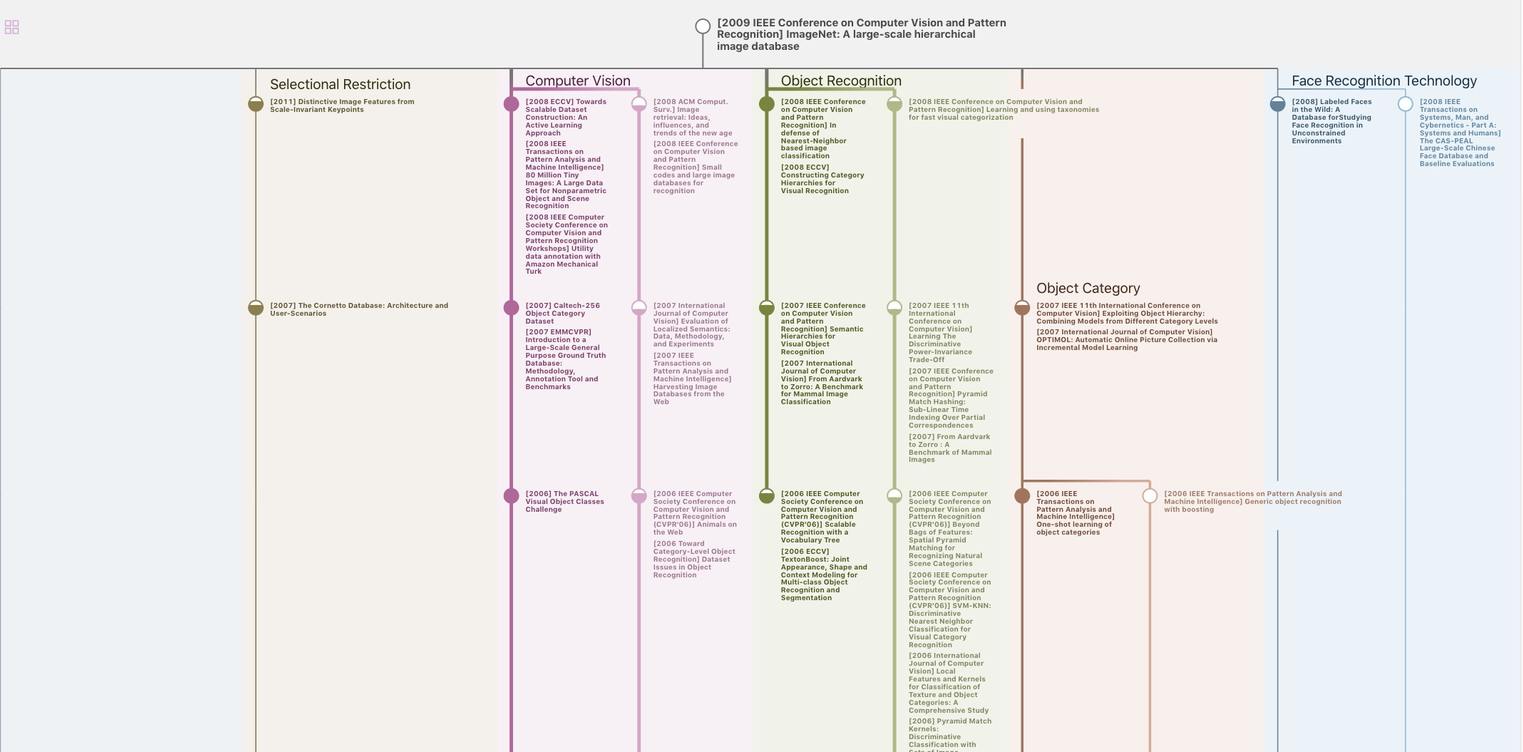
生成溯源树,研究论文发展脉络
Chat Paper
正在生成论文摘要