Parallel Defect Detection Model on Uncertain Data for GPUs Computing by a Novel Ensemble Learning
Applications of Artificial Intelligence for Smart TechnologyAdvances in Computational Intelligence and Robotics(2021)
关键词
gpus computing,novel ensemble learning,uncertain data,defect
AI 理解论文
溯源树
样例
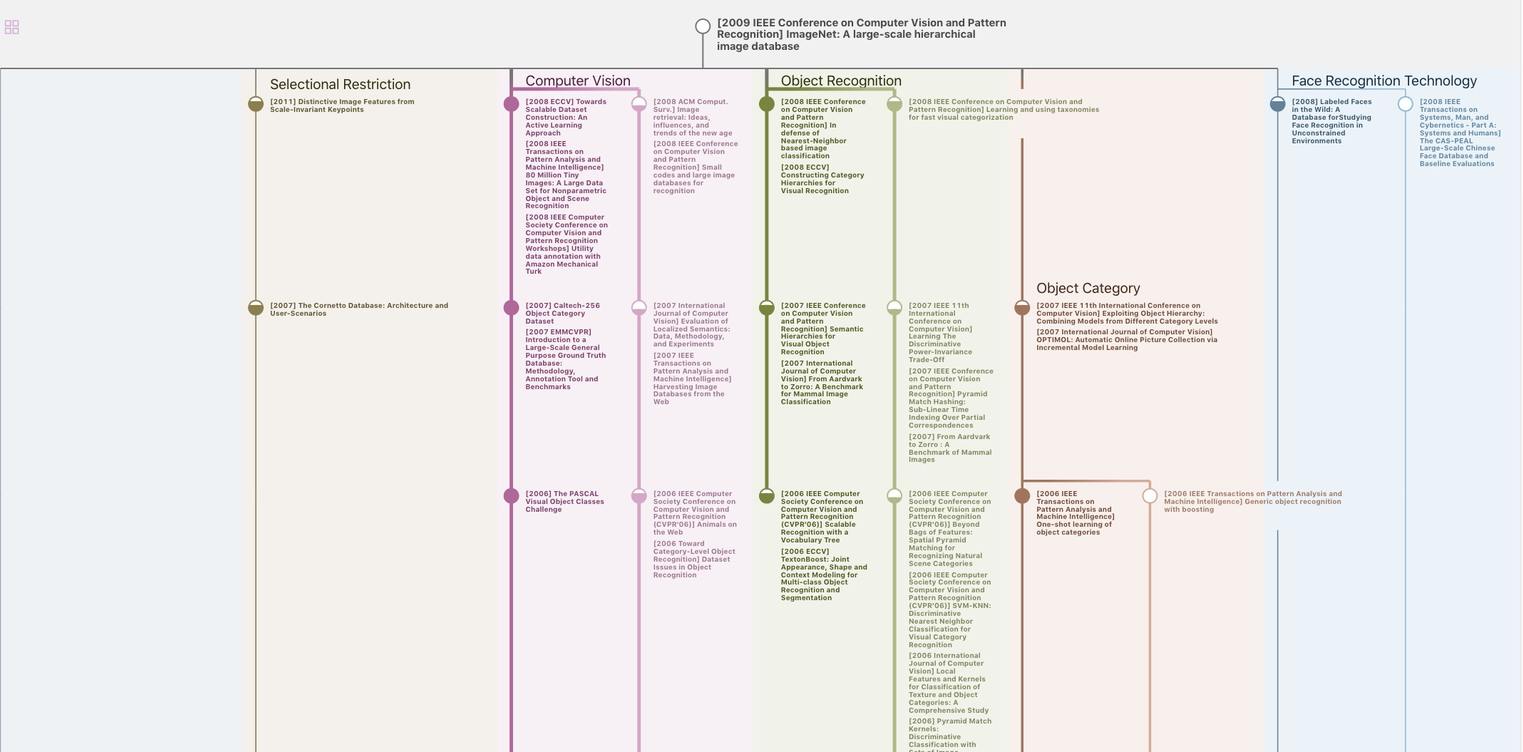
生成溯源树,研究论文发展脉络
Chat Paper
正在生成论文摘要