Detection of Black Spot of Rose Based on Hyperspectral Imaging and Convolutional Neural Network
AgriEngineering(2020)
摘要
Black spot is one of the seriously damaging plant diseases in China, especially in rose production. Hyperspectral technology reflects both external features and internal structure information of measured samples, which can be used to identify the disease. In this research, both the spectral and image features of two infected roses with black spot were used to train a convolutional neural network (CNN) model. Multiple scattering correction (MSC) and standard normal variable (SNV) methods were applied to preprocess the spectral data. Cropping, median filtering and binarization were pretreatments used on the hyperspectral images. Three CNN models based on Alexnet, VGG16 and neural discriminative dimensionality reduction (NDDR) were evaluated by analyzing the classification accuracy and loss function. The results show that the CNN model based on the fusion of features has higher accuracy. The highest accuracies of detection of blackspot in different roses are 12–26 (100%) and 13–54 (99.95%), applying the NDDR-CNN model. Therefore, this research indicates that the spectral analysis based on CNN can detect black spot of roses, which provides a reference for the detection of other plant diseases, and has favorable research significance as well as prospect for development.
更多查看译文
关键词
hyperspectral imaging, black spot, infected samples, non-destructive detection, preprocessing, CNN model
AI 理解论文
溯源树
样例
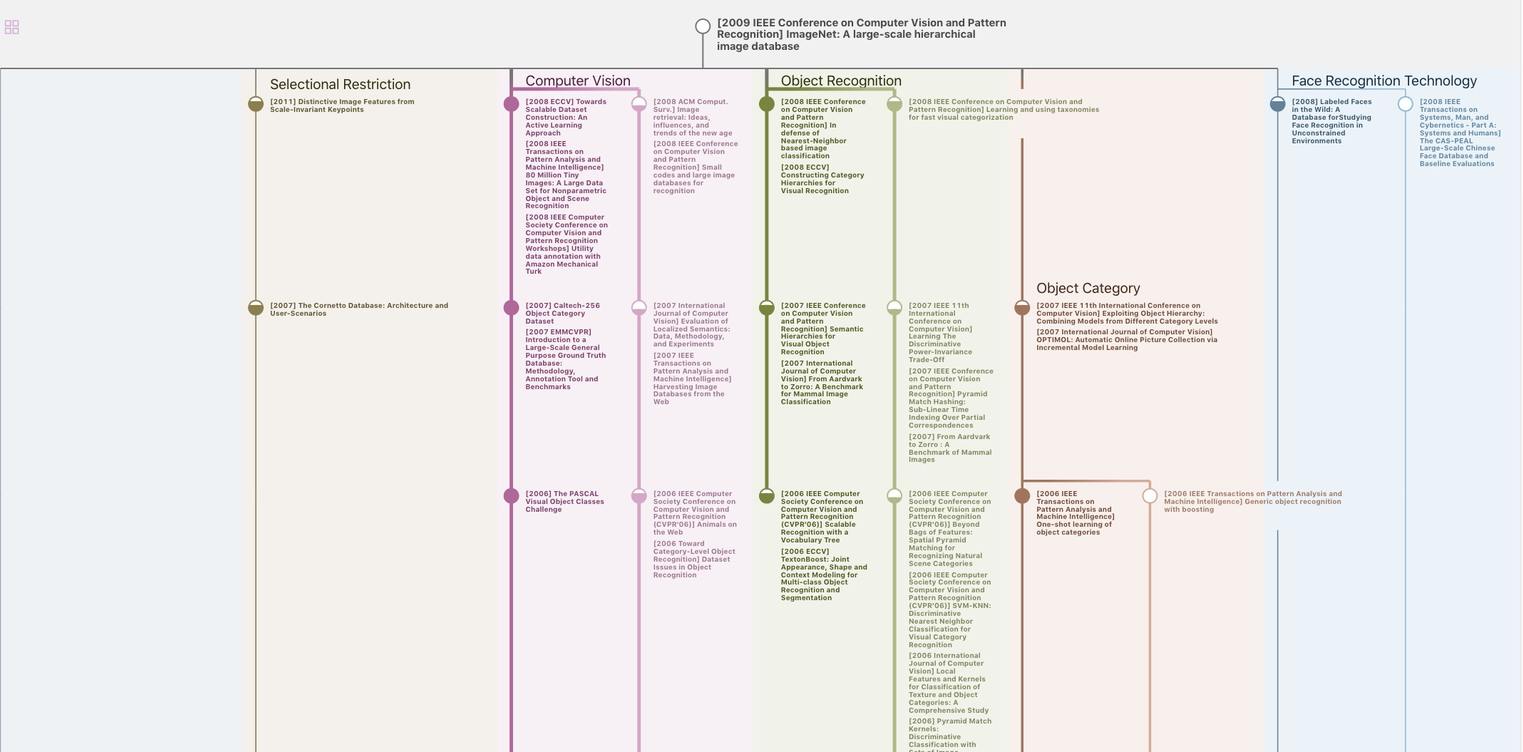
生成溯源树,研究论文发展脉络
Chat Paper
正在生成论文摘要