Pro-ML IDeAS: A Probabilistic Framework for Explicit Inverse Design using Invertible Neural Network
AIAA Scitech 2021 Forum(2021)
Abstract
An inverse design process has the potential to positively impact the difficulties of the traditional iterative process if the design candidates are ``explicitly'' generated from target performance metrics. The explicit inverse design methods can not only bypass the time-consuming iterative process, it can also allow the designer to explore the design space beyond their initial intended target performance in a much more efficient manner. With recent advances in Machine Learning new methods have revolutionized the inverse design process. However, the current approaches struggle with high-dimensional design space, require large amounts of high-fidelity training data, and have difficulty dealing with ill-posed, ill-conditioned issues. In this work we developed a Probabilistic Machine Learning for Inverse Design of Aerodynamic Systems (Pro-ML IDeAS) framework that overcomes these challenges and achieves the desired outcome with limited budget. The framework follows a two-step process. In the first step an efficient probabilistic generative model of the forward process is built using limited data. In the second step a surrogate of the inverse process is built using Invertible Neural Network (INN) using the data sampled from the generative model. In this work, we will demonstrate the framework on analytical functions and aerodynamic design of gas turbine airfoils.
MoreTranslated text
Key words
explicit inverse design,invertible neural network,neural network,probabilistic framework
AI Read Science
Must-Reading Tree
Example
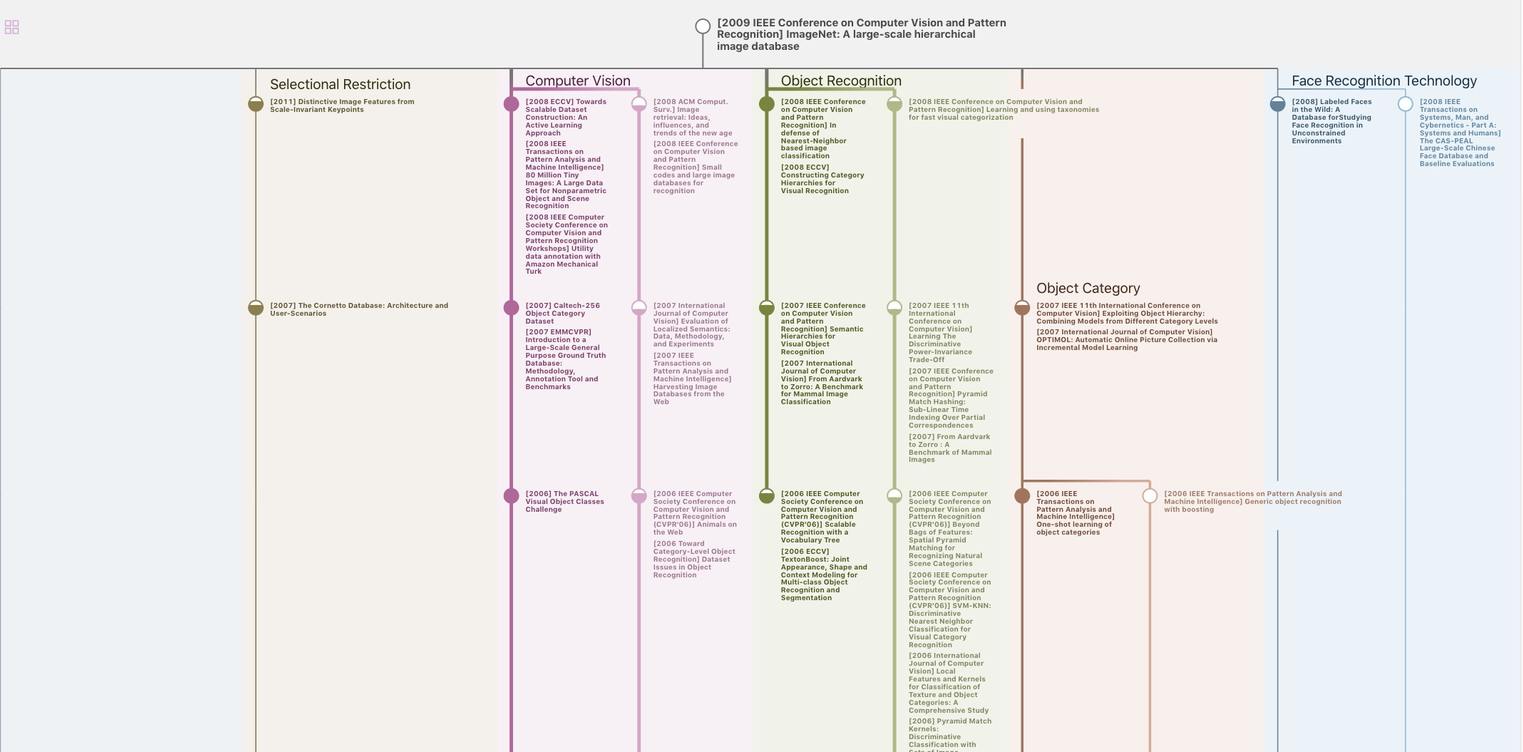
Generate MRT to find the research sequence of this paper
Chat Paper
Summary is being generated by the instructions you defined