Connectedness matters: construction and exact random sampling of connected networks
Journal of Physics: Complexity(2021)
摘要
Abstract We describe a new method for the random sampling of connected networks with a specified degree sequence. We consider both the case of simple graphs and that of loopless multigraphs. The constraints of fixed degrees and of connectedness are two of the most commonly needed ones when constructing null models for the practical analysis of physical or biological networks. Yet handling these constraints, let alone combining them, is non-trivial. Our method builds on a recently introduced novel sampling approach that constructs graphs with given degrees independently (unlike edge-switching Markov chain Monte Carlo methods) and efficiently (unlike the configuration model), and extends it to incorporate the constraint of connectedness. Additionally, we present a simple and elegant algorithm for directly constructing a single connected realization of a degree sequence, either as a simple graph or a multigraph. Finally, we demonstrate our sampling method on a realistic scale-free example, as well as on degree sequences of connected real-world networks, and show that enforcing connectedness can significantly alter the properties of sampled networks.
更多查看译文
关键词
networks,random graphs,degree sequence,connected graphs,null models,algorithms,random sampling
AI 理解论文
溯源树
样例
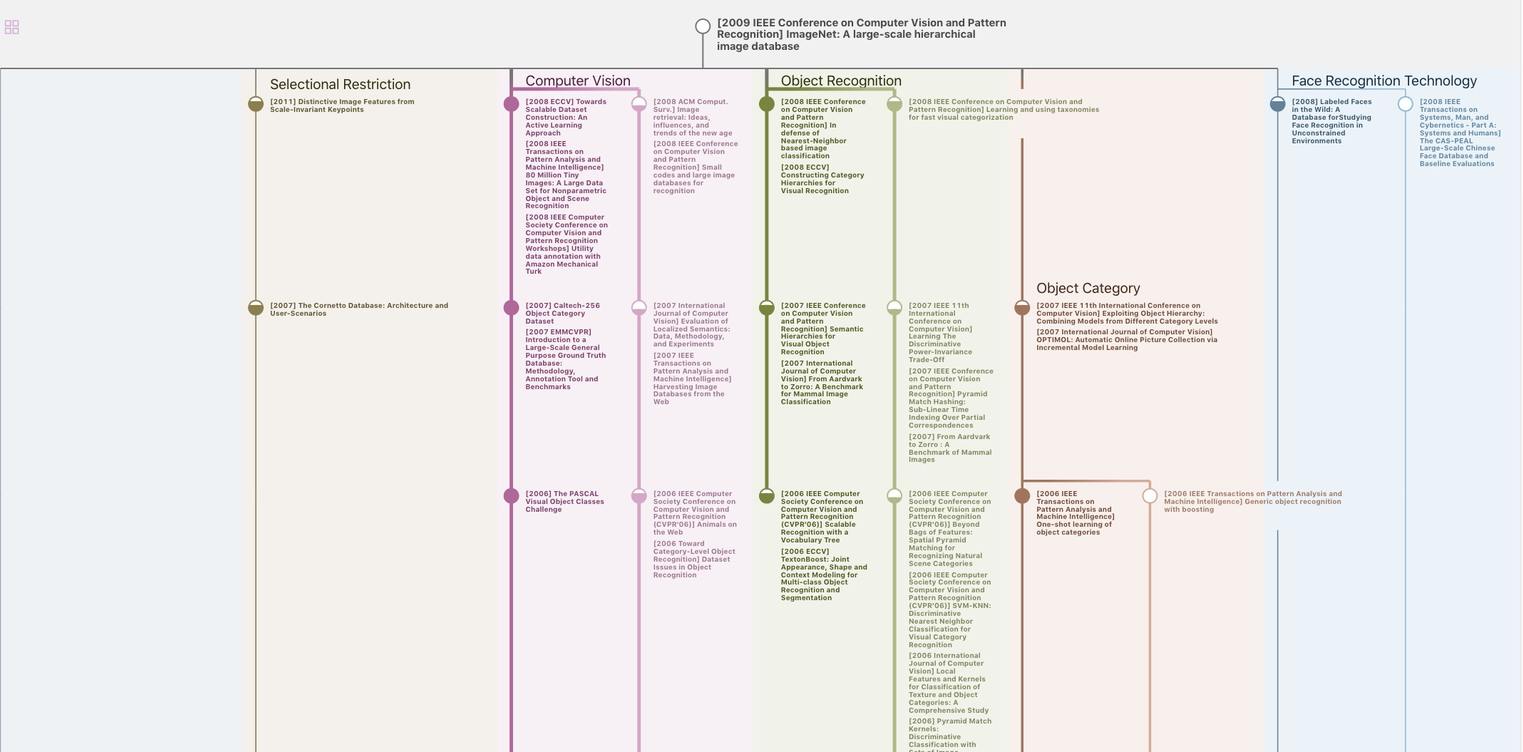
生成溯源树,研究论文发展脉络
Chat Paper
正在生成论文摘要