Expectile regression via deep residual networks
STAT(2021)
摘要
Expectile is a generalization of the expected value in probability and statistics. In finance and risk management, the expectile is considered to be an important risk measure due to its connection with gain-loss ratio and its coherent and elicitable properties. Linear multiple expectile regression was proposed in 1987 for estimating the conditional expectiles of a response given a set of covariates. Recently, more flexible nonparametric expectile regression models were proposed based on gradient boosting and kernel learning. In this paper, we propose a new nonparametric expectile regression model by adopting the deep residual network learning framework and name it Expectile NN. Extensive numerical studies on simulated and real datasets demonstrate that Expectile NN has very competitive performance compared with existing methods. We explicitly specify the architecture of Expectile NN so that it is easy to be reproduced and used by others. Expectile NN is the first deep learning model for nonparametric expectile regression.
更多查看译文
关键词
deep learning,expectile regression,residual networks
AI 理解论文
溯源树
样例
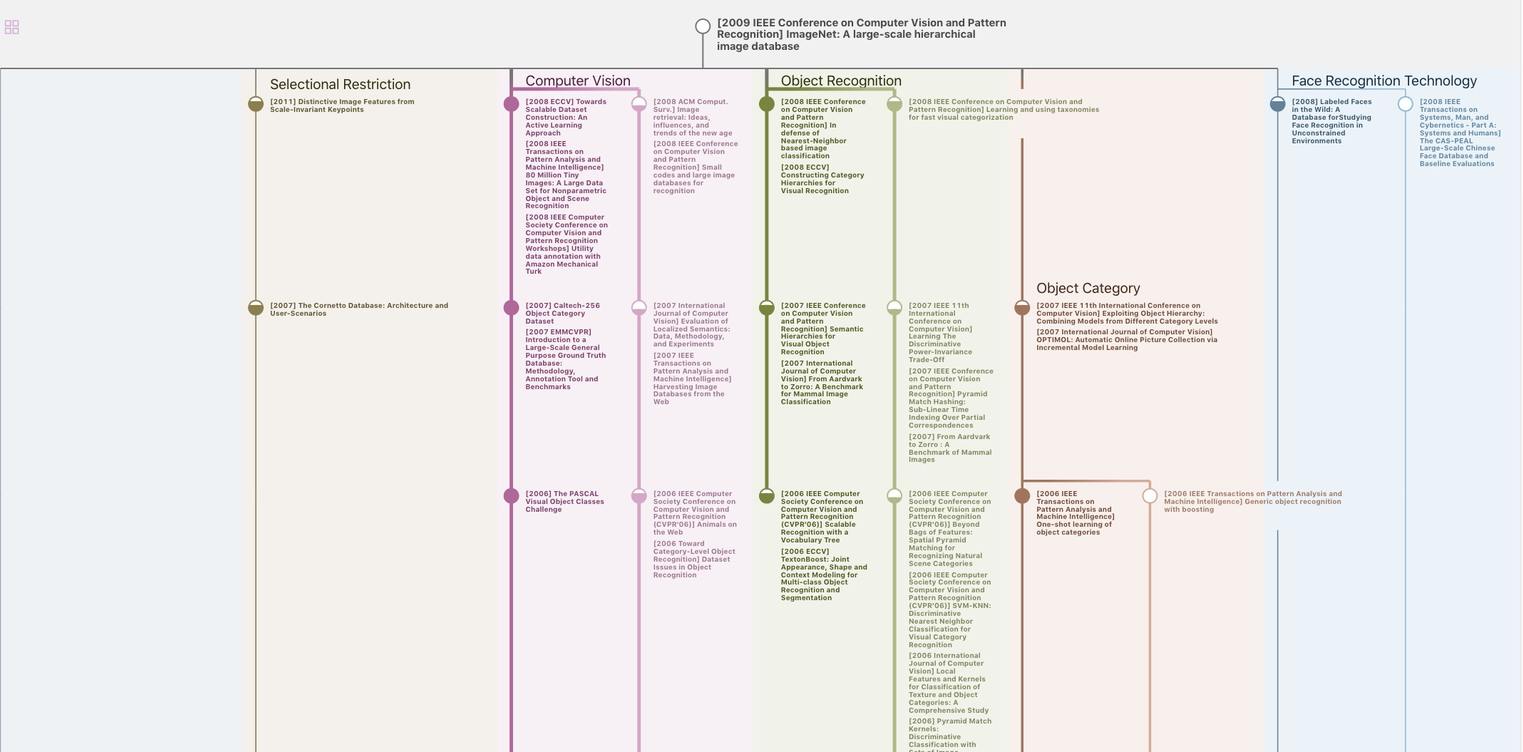
生成溯源树,研究论文发展脉络
Chat Paper
正在生成论文摘要