Network Representation Learning Based Extended Matrix Factorization for Recommendation
Communications in Computer and Information ScienceArtificial Intelligence and Security(2020)
摘要
The growing heterogeneous data in the Internet effectively improves the performance of recommender systems (RS). The main problem faced by the traditional matrix factorization (MF) is how to fuse more heterogeneous information data in MF to improve the performance of RS. In view of this, this paper proposes an Extended Matrix Factorization (EMF) based on network representation learning. EMF integrates multiple types of data in Heterogeneous Information Network (HIN) to improve the accuracy of prediction rating. The nodes in HIN are first mapped to a low-dimensional representation vector by network representation learning (NRL). Then the representation vector is used as the input of the EMF, the parameters are optimized by the gradient descent, and finally the prediction model is obtained. The experiments on two real data sets show the effectiveness of the EMF. Compared with the baseline algorithms, the EMF model can obtain more accurate prediction rating.
更多查看译文
关键词
recommendation,matrix
AI 理解论文
溯源树
样例
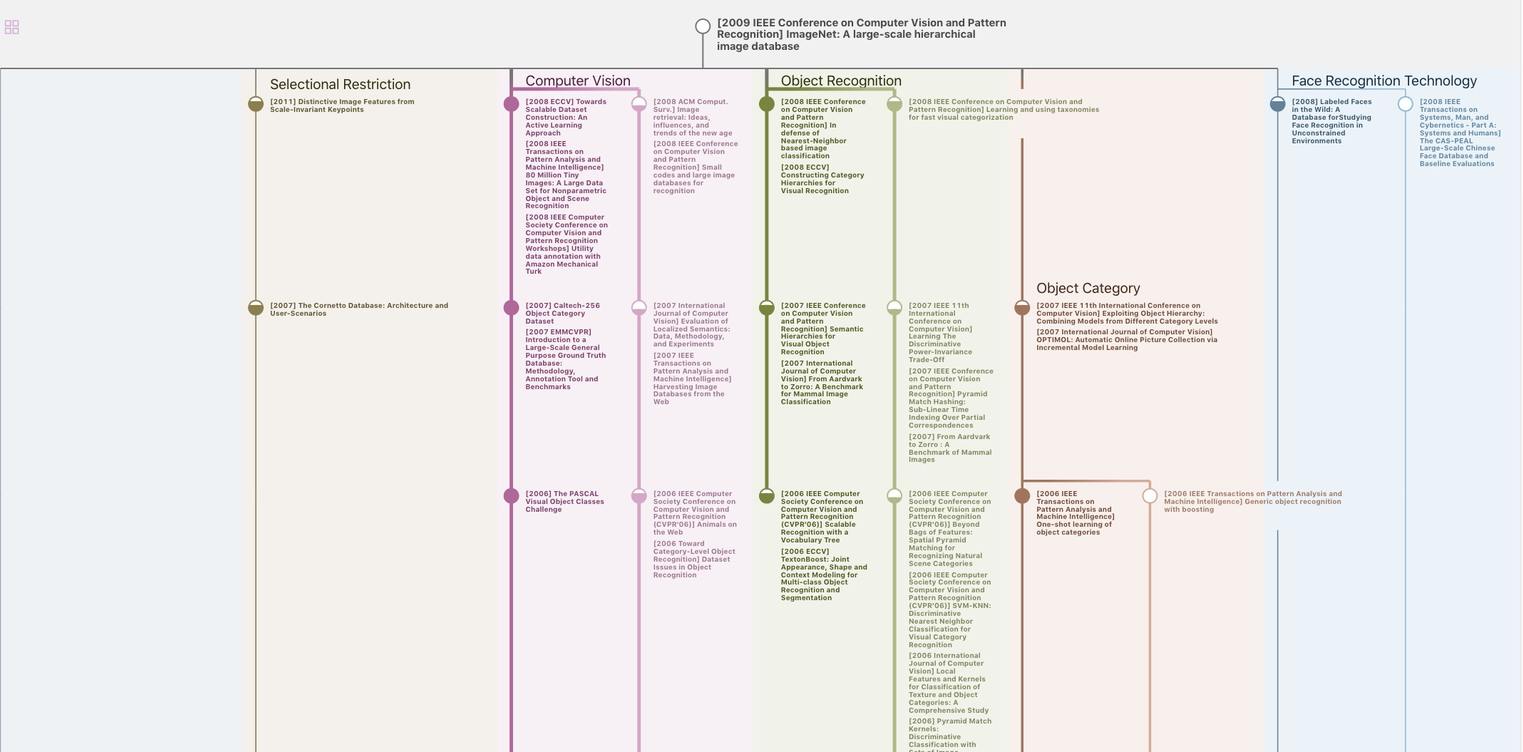
生成溯源树,研究论文发展脉络
Chat Paper
正在生成论文摘要