A Probabilistic View of EOR Lab Experiments: An Example of Low Salinity Flooding
Day 2 Tue, September 01, 2020(2020)
摘要
Abstract During implementation of an EOR technique, such as low salinity watearflooding, the first step generally consists of a coreflood to obtain an initial estimate of possible production and recovery rates. Most of the parameters believed to be relevant for recovery cannot be directly observed or measured. Instead, the physical response measured in the lab is used to infer these parameters. Mechanistic reservoir simulations provide the interconnection between the measured physical response and parameters thereby enabling us to estimate the parameter values. Due to the nonlinear nature of a displacement, multiple parameters may describe a given measurement. This inversion problem is generally addressed using manual or semi-automated history matching that can be difficult and time-consuming to employ, especially when chemical processes are involved because a significant number of additional parameters are added. This conventional approach is deterministic. This paper presents an alternative stochastic formulation of the inversion problem for low-salinity waterflood based on Bayesian statistics. Instead of directly constructing a core model that matches the observed measurements, apriori information regarding the parameters obtained from expert opinion is used to construct a set of prior core models. By forward simulating these prior models, we obtain a set of predictions (or expectations) for given parameter values. While none of these prior predictions may match exactly our observed measurement, we use the predictions to infer a statistical relationship between the parameter and the measurements. Such relationships allow us to compute the conditional probability of a parameter given our observed measurement. One of the challenges associated with statistical modeling of time series measurements is the large dimensionality. We used Functional Data Analysis and Mixed Principal Component Analysis to reduce the dimensionality of the measurements. Kernel Density Estimation is then used to estimate a posterior distribution for each of the uncertain system parameters. The proposed workflow is applicable to any physical process where parameters of interest cannot be directly observed. To demonstrate the ability of the proposed workflow, a low salinity core flood is used as an example of an EOR process. The results show that the method is indeed able to reduce the uncertainty in parameter values when compared to the prior probability distribution.
更多查看译文
AI 理解论文
溯源树
样例
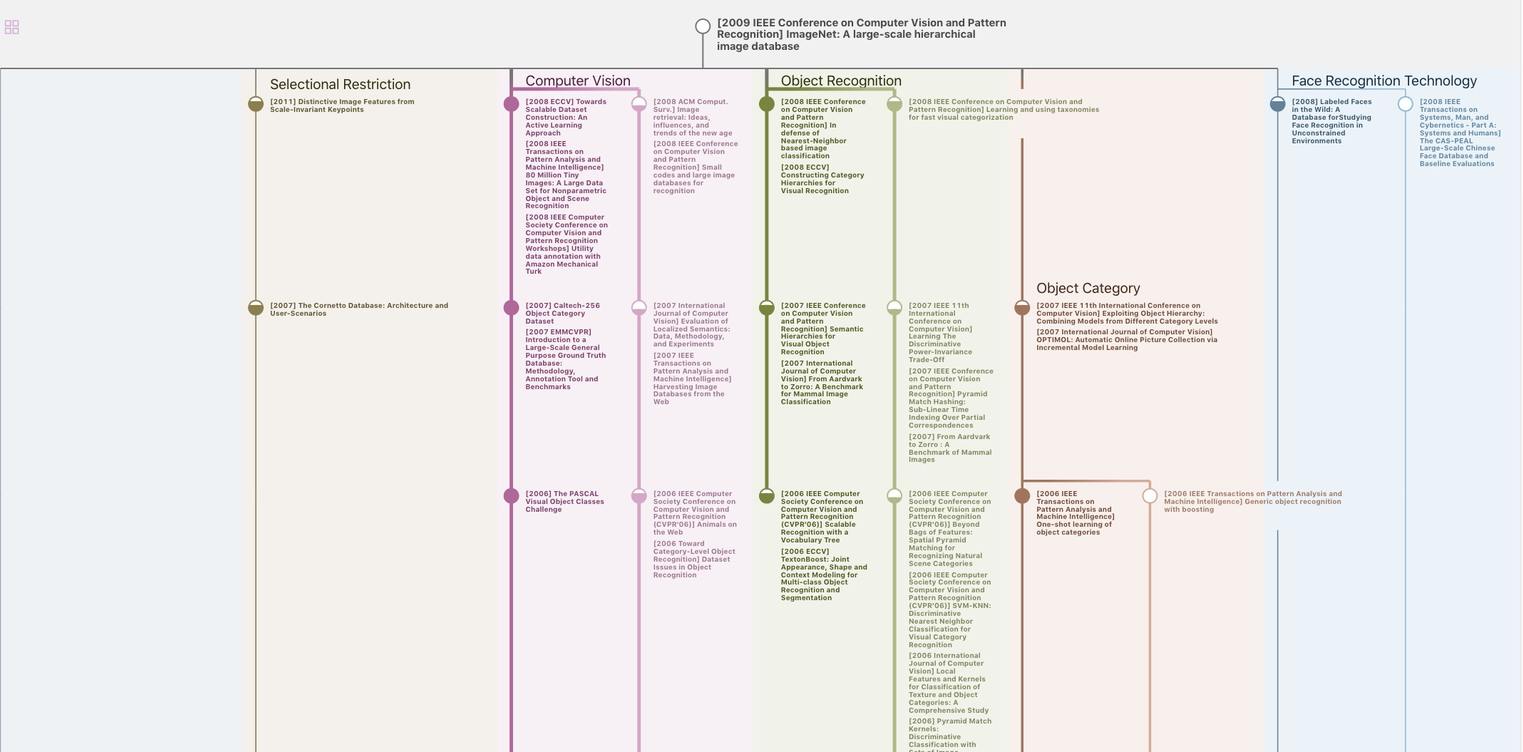
生成溯源树,研究论文发展脉络
Chat Paper
正在生成论文摘要