Visualizing ECG Contribution into Convolutional Neural Network Classification
Feature Engineering and Computational Intelligence in ECG Monitoring(2020)
摘要
Convolutional Neural Network (CNN) demonstrated impressive classification performance on ElectroCardioGram (ECG) analysis and has a great potential to extract salient patterns from signal. Visualizing local contributions of ECG input to a CNN classification model can both help us understand why CNN can reach such high-level performances and serve as a basis for new diagnosis recommendations. This chapter builds a single-layer 1-D CNN model on ECG classification with a 99% accuracy. The trained model is then used to build a 1-D Deconvolved Neural Network (1-D DeconvNet) for visualization. We propose Feature Importance Degree Heatmap (FIDH) to interpret the contribution of each point of ECG input to CNN classification results, and thus to show which part of ECG raises attention of the classifier. We also illustrate the correlation between two visualization methods: first-order Taylor expansion and multilayer 1-D DeconvNet.
更多查看译文
关键词
ecg contribution,convolutional neural network,classification
AI 理解论文
溯源树
样例
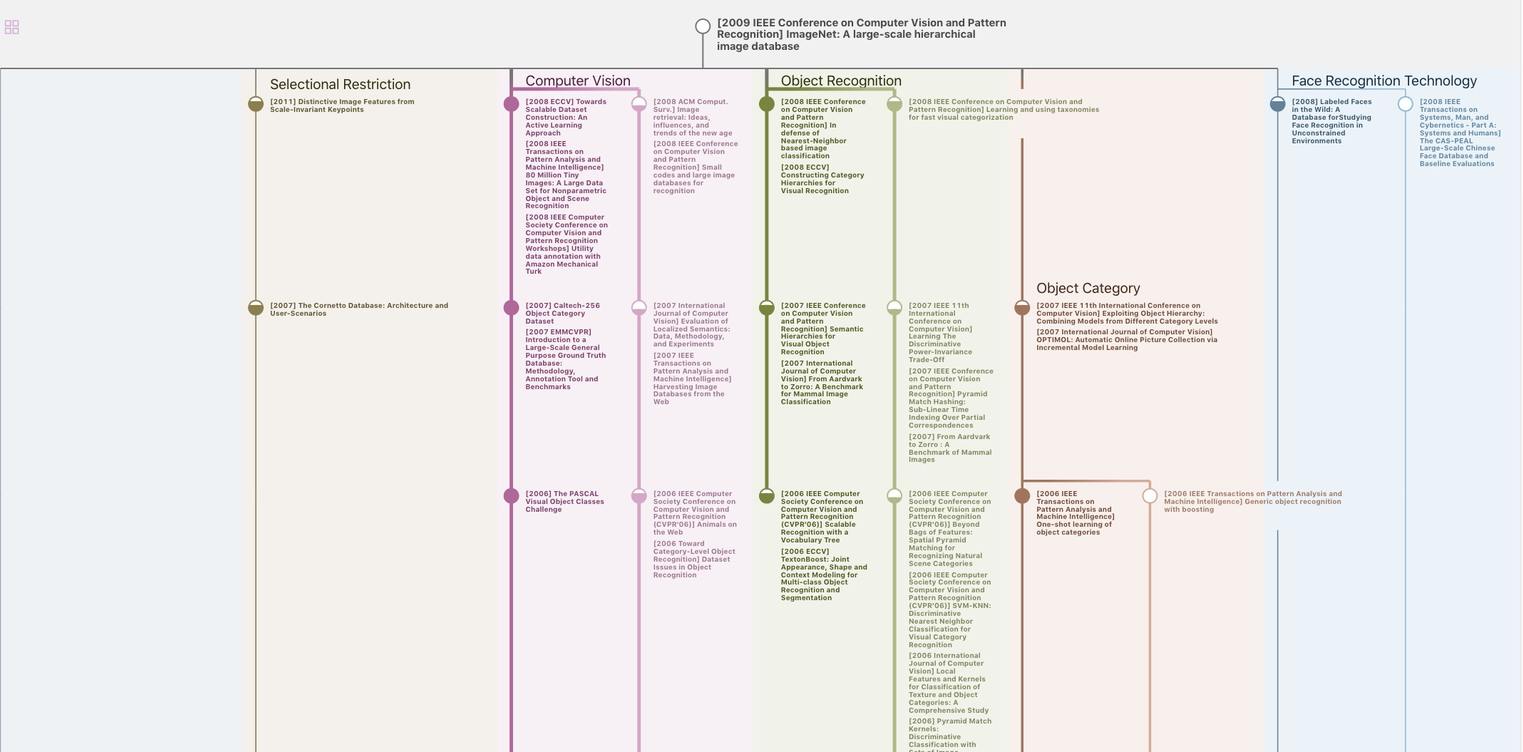
生成溯源树,研究论文发展脉络
Chat Paper
正在生成论文摘要