Partial Robust M-Regression Estimator in the Presence of Multicollinearity and Vertical Outliers
Journal of Physics: Conference Series(2020)
Abstract
Abstract The objective of using regression is to explain the variation in one or more response variables by associating the variation with proportional variation in one or more explanatory variables. However, if the number of independent variables is multiple, they tend to be highly collinear and this contributes to multicollinearity problem. For instance, Ridge Regression (RR), Principal Component Regression (PCR), and Partial Least Squares Regression (PLSR) are some of the prediction methods used to handle dataset with multicollinearity. In addition, another problem that arises is the existence of outlying objects in a dataset. The effect of outlying data points in the presence of multicollinearity problem could be reduced with the implementation of robust regression method. A recently studied robust PLSR, which is called Partial Robust M-Regression (PRM) is found to be able in dealing with multicollinearity and outliers simultaneously. This method was employed in this study. Throughout this study, five methods of regression were chosen; OLS, RR, PCR, PLSR, and PRM, to compare which is the best method in their predictive ability. To compare these five regression methods, a simulation study had been conducted, and the mean square error of β estimate (MSE(β)) was calculated. The simulation results show that PRM outperforms other method in the presence of multicollinearity, outliers and leverage points.
MoreTranslated text
Key words
vertical outliers,multicollinearity,m-regression
AI Read Science
Must-Reading Tree
Example
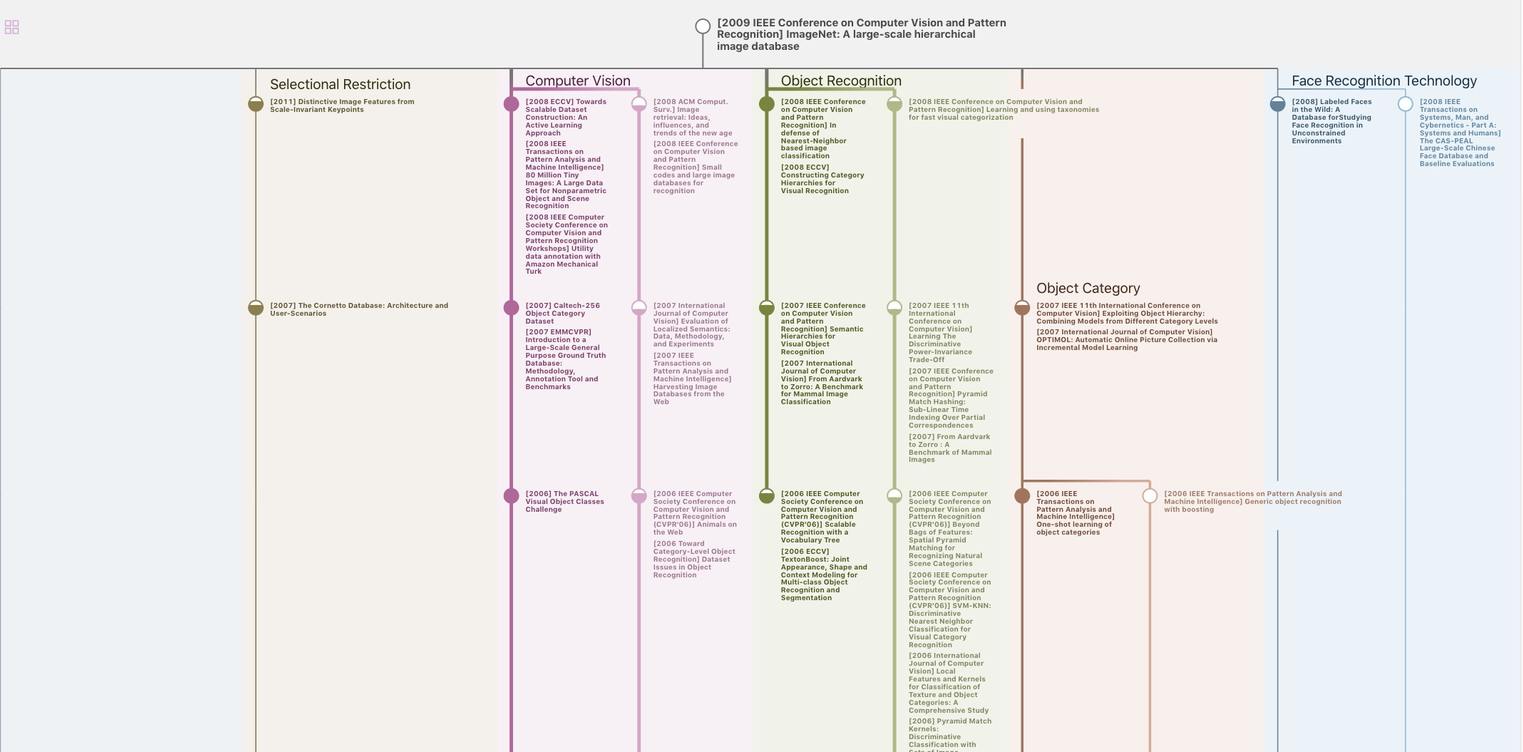
Generate MRT to find the research sequence of this paper
Chat Paper
Summary is being generated by the instructions you defined