Biomarker Identification in Colorectal Cancer Using Subnetwork Analysis with Feature Selection
Recent Advances in Information and Communication Technology 2020Advances in Intelligent Systems and Computing(2020)
摘要
Gene Sub-Network-based Feature Selection (GSNFS) is an efficient method for handling case-control and multiclass studies for gene sub-network biomarker identification by an integrated analysis of gene expression, gene-set and network data. However, GSNFS has produce considerably high number of sub-network and has not assessed the importance of each sub-network. Recently, we have incorporated 2 feature selection techniques; correlation-based and information gain into the GSNFS workflow to help reduce the number and assess the importance of each individual sub-network. The extended GSNFS method was clearly shown to identify good candidate gene subnetwork markers in lung cancer. In this work, we applied a similar work flow to colorectal cancer. First, the top- and bottom- 5 ranked gene-sets were selected and investigated the classification performance. Similarly, the top-ranked gene-sets showed a better performance than the bottom-ranked gene-sets. The identified top-ranked gene-sets such as TNF-beta and MAPK signaling pathway were known to relate to cancer. In addition, the characteristic of top identified pathway network was further analyzed and visualized. SMAD3, a gene that was reported to be related to cancer by many studies, was mostly found to have the highest neighbor in 4 datasets. The results in this study has confirmed that GSNFS combined with feature selection is very promising as significantly fewer subnetworks were needed to build a classifier and gave a comparable performance to a full dataset classifier.
更多查看译文
关键词
subnetwork analysis,feature selection,colorectal cancer
AI 理解论文
溯源树
样例
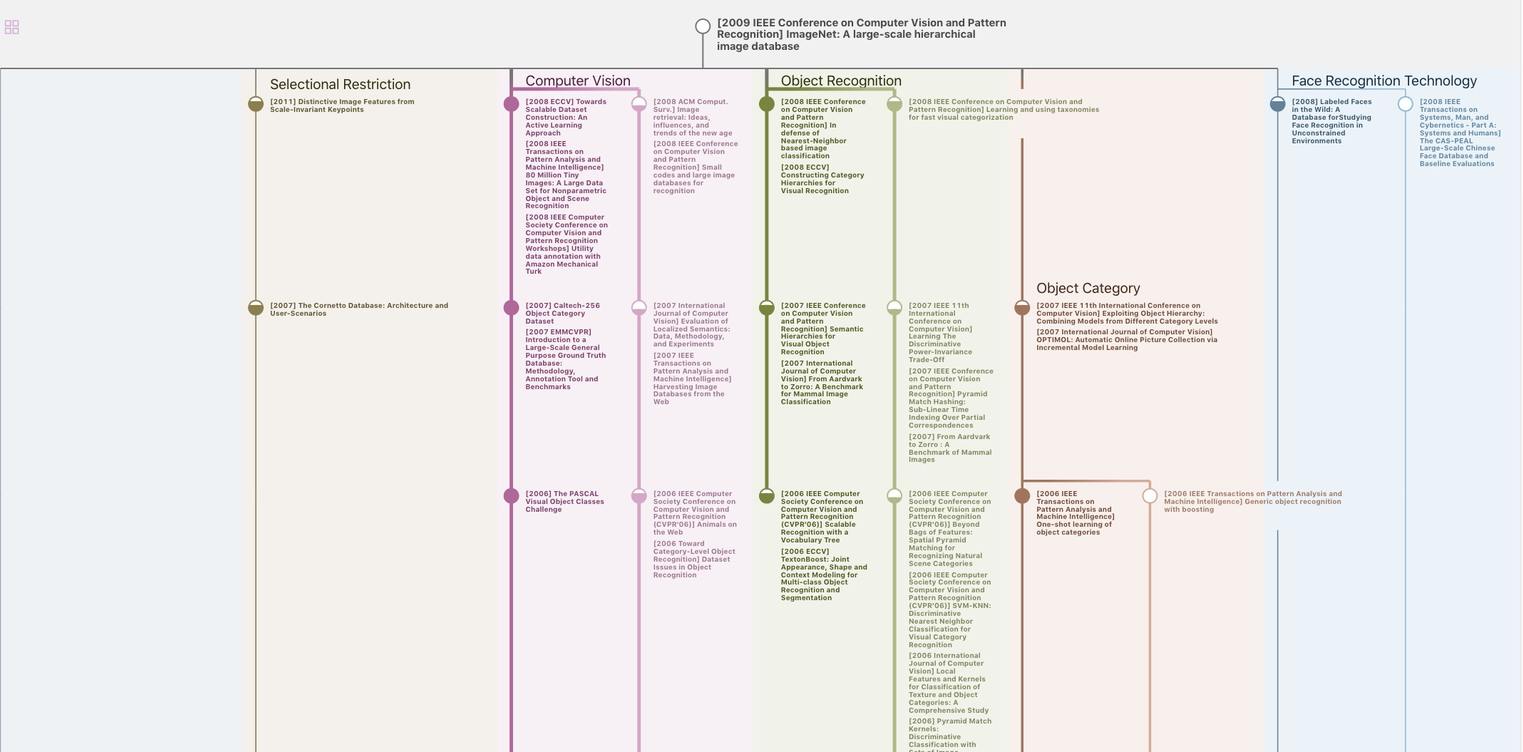
生成溯源树,研究论文发展脉络
Chat Paper
正在生成论文摘要