Connected Graphs with a Given Degree Sequence: Efficient Sampling, Correlations, Community Detection and Robustness
Proceedings of NetSci-X 2020: Sixth International Winter School and Conference on Network ScienceSpringer Proceedings in Complexity(2020)
摘要
Random graph models can help us assess the significance of the structural properties of real complex systems. Given the value of a graph property and its value in a randomized ensemble, we can determine whether the property is explained by chance by comparing its real value to its value in the ensemble. The conclusions drawn with this approach obviously depend on the choice of randomization. We argue that keeping graphs in one connected piece, or component, is key for many applications where complex graphs are assumed to be connected either by definition (e.g. the Internet) or by construction (e.g. a crawled subset of the World-Wide Web obtained only by following hyperlinks). Using an heuristic to quickly sample the ensemble of small connected simple graphs with a fixed degree sequence, we investigate the significance of the structural patterns found in real connected graphs. We find that, in sparse networks, the connectedness constraint changes degree correlations, the outcome of community detection with modularity, and the predictions of percolation on the ensemble.
更多查看译文
关键词
community detection,graphs,efficient sampling,correlations
AI 理解论文
溯源树
样例
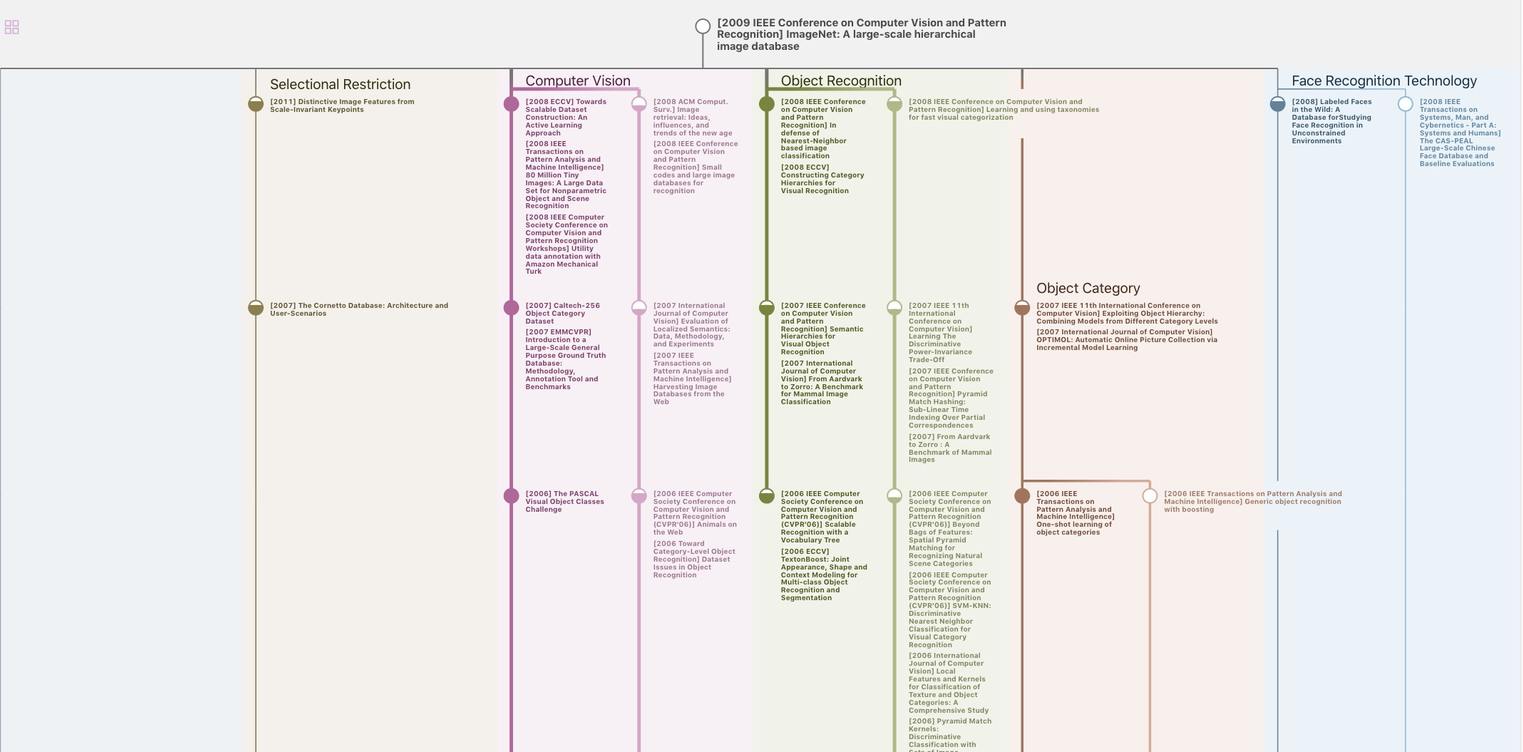
生成溯源树,研究论文发展脉络
Chat Paper
正在生成论文摘要