Holistic Affect Recognition Using PaNDA: Paralinguistic Non-metric Dimensional Analysis
IEEE Transactions on Affective Computing(2020)
摘要
Humans perceive emotion from each other using a holistic perspective, accounting for diverse personal, non-emotional variables, such as age and personality, that shape expression. In contrast, today’s algorithms are mainly designed to recognize emotion in isolation, and are usually demonstrated only within one relatively narrow database. In this article, we propose a multi-task learning approach to jointly learn the recognition of affective states from speech along with various speaker attributes. A problem with multi-task learning is that sometimes inductive transfer can negatively impact performance. To mitigate negative transfer, we introduce the Paralinguistic Non-metric Dimensional Analysis (PaNDA) method that systematically measures task relatedness and also enables visualizing the topology of affective phenomena as a whole. In addition, we present a generic framework that conflates the concepts of single-task and multi-task learning. Using this framework, we construct two models that demonstrate holistic affect recognition: one treats all tasks as equally related, whereas the other one incorporates the task correlations between a main task and its supporting tasks obtained from PaNDA. Both models employ a multi-task deep neural network, in which separate output layers are used to predict discrete and continuous attributes, while hidden layers are shared across different tasks. On average across 18 classification and regression tasks, the weighted multi-task learning with PaNDA significantly improves performance compared to single-task and unweighted multi-task learning.
更多查看译文
关键词
Holistic context,speaker attributes,affective space,task relatedness,multi-task learning
AI 理解论文
溯源树
样例
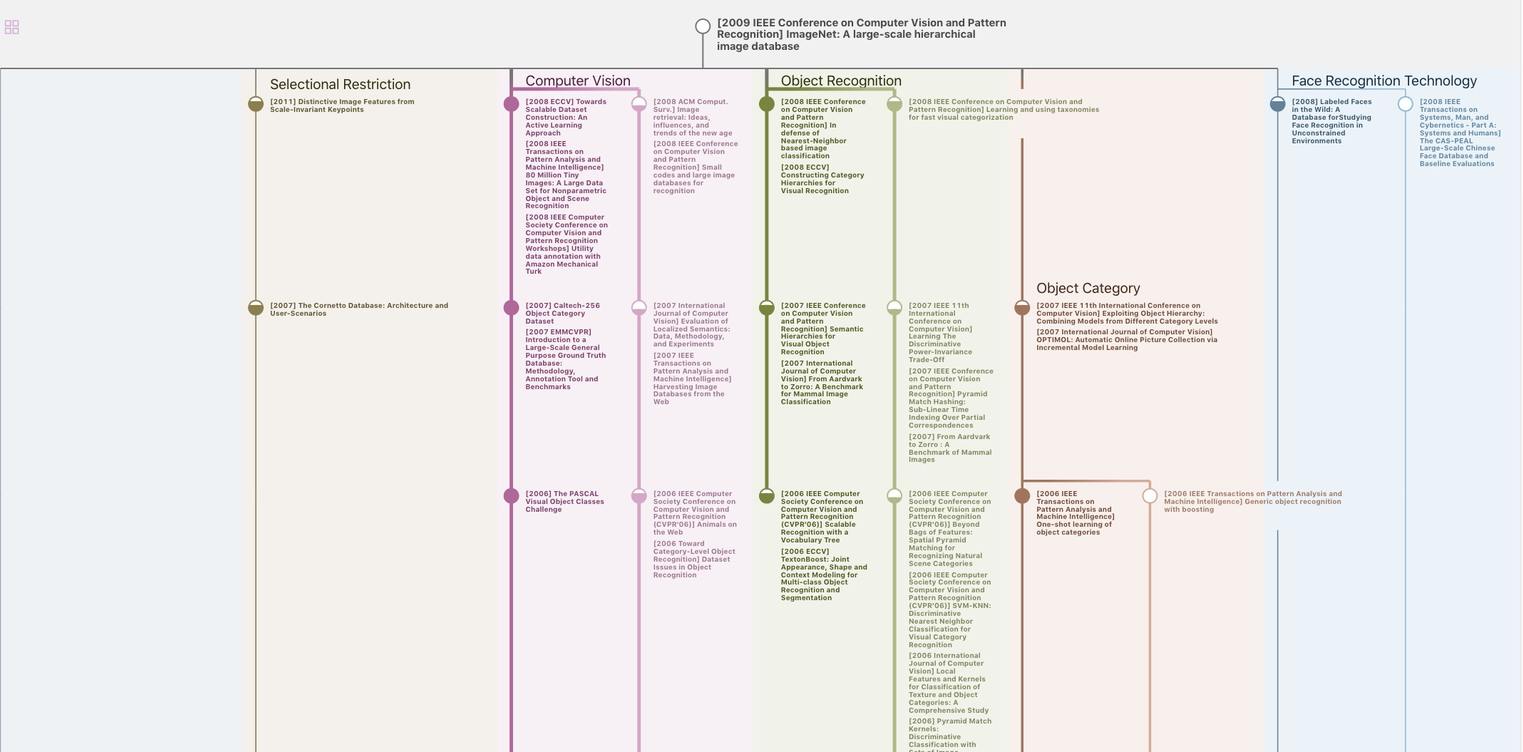
生成溯源树,研究论文发展脉络
Chat Paper
正在生成论文摘要