Segmentation using transfer learning in robotic microvascular anastomosis
The Proceedings of JSME annual Conference on Robotics and Mechatronics (Robomec)(2019)
摘要
Robotic surgical automation has the potential to stably provide good clinical outcomes and reduce fatigues, operation time and medical errors. In order to automate surgical tasks, autonomous environment recognition is required. In this paper, we proposed a segmentation method using deep convolutional neural networks for robotic microvascular anastomosis. In order to train the deep convolutional neural networks, enormous training data is required. However, manual annotation of ground truth mask is a time-consuming task. Therefore, we proposed transfer learning using simulated images. After our network is trained on a large data set of simulated images, our network is trained on a small data set of real images. In the experiment, the network trained with the transfer learning achieved high accuracy for robotic tools and blood vessels despite that we used a small number of real images. However, we need to solve the imbalanced data problem to improve accuracy for a surgical needle.
更多查看译文
关键词
transfer learning,segmentation,microvascular
AI 理解论文
溯源树
样例
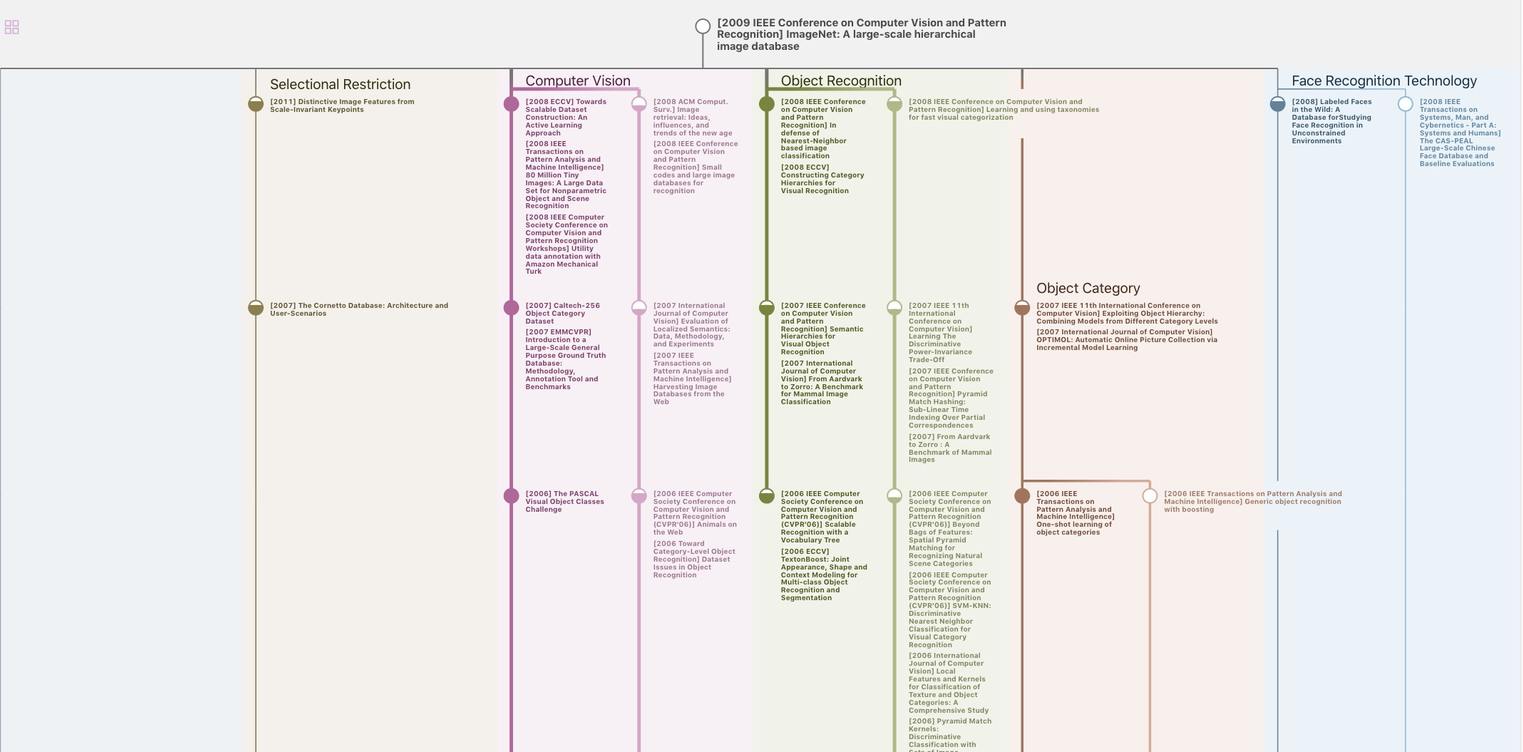
生成溯源树,研究论文发展脉络
Chat Paper
正在生成论文摘要