Ensemble Learning via Extreme Learning Machines for Imbalanced Data
Advances in Computational Intelligence and RoboticsInnovations, Algorithms, and Applications in Cognitive Informatics and Natural Intelligence(2020)
摘要
Ensembles are known to reduce the risk of selecting the wrong model by aggregating all candidate models. Ensembles are known to be more accurate than single models. Accuracy has been identified as an important factor in explaining the success of ensembles. Several techniques have been proposed to improve ensemble accuracy. But, until now, no perfect one has been proposed. The focus of this research is on how to create accurate ensemble learning machine (ELM) in the context of classification to deal with supervised data, noisy data, imbalanced data, and semi-supervised data. To deal with mentioned issues, the authors propose a heterogeneous ELM ensemble. The proposed heterogeneous ensemble of ELMs (AELME) for classification has different ELM algorithms, including regularized ELM (RELM) and kernel ELM (KELM). The authors propose new diverse AdaBoost ensemble-based ELM (AELME) for binary and multiclass data classification to deal with the imbalanced data issue.
更多查看译文
关键词
extreme learning machines,ensemble learning
AI 理解论文
溯源树
样例
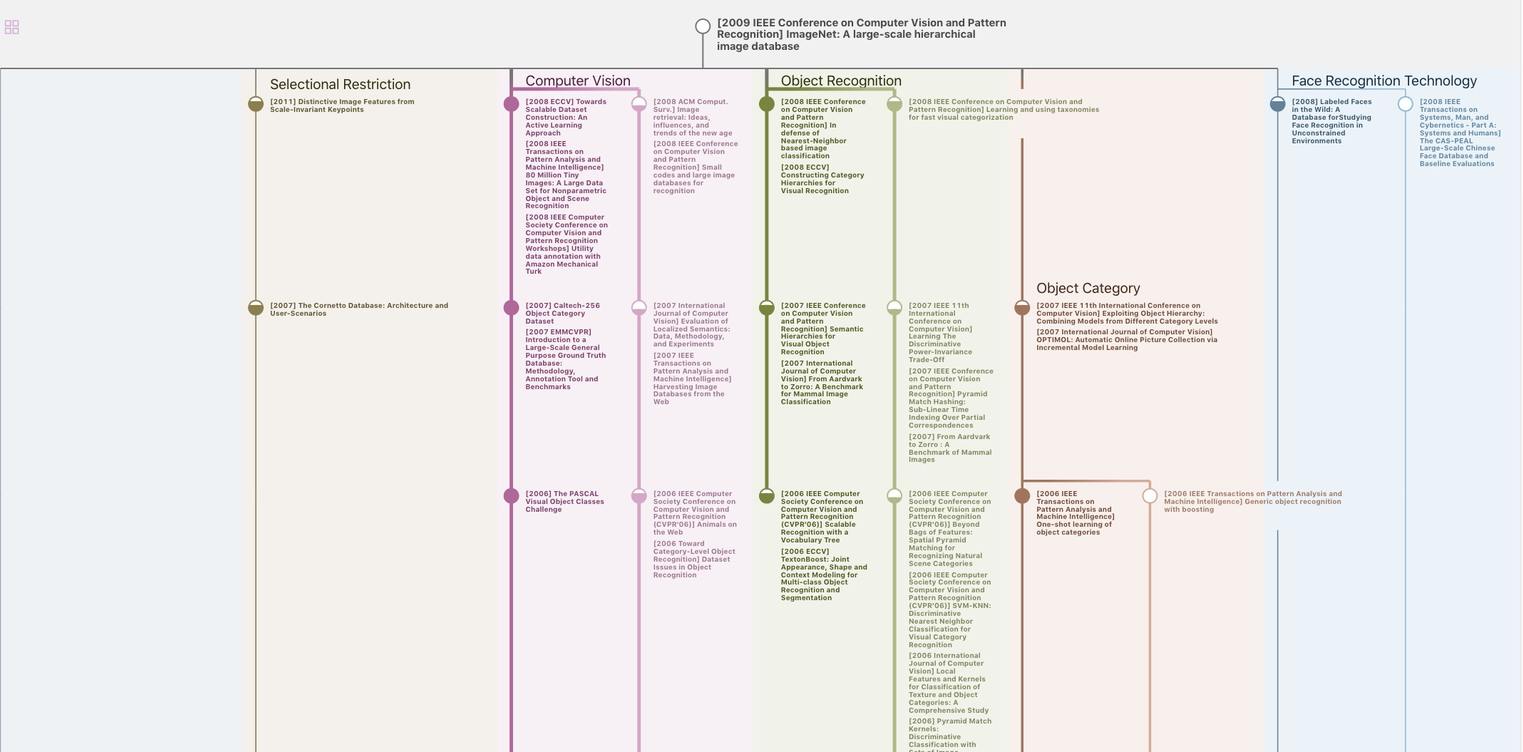
生成溯源树,研究论文发展脉络
Chat Paper
正在生成论文摘要