Exploration of Long Time-of-Flight Three-Body Transfers Using Deep Reinforcement Learning
AIAA Scitech 2020 Forum(2020)
摘要
Low-energy transfers reduce the total ∆V in deep space missions by exploiting multibody dynamics. However, searching for initial guesses in highly nonlinear systems is often a demanding task and it has been heavily dependent on professionals. In this paper, we aim to automate this initial guess search by using deep reinforcement learning (RL) algorithms. Minimum fuel transfer problems from low-Earth orbit to three-body orbits are redefined in the RL framework with the ∆V vector as an action. Since a common RL algorithm, deep deterministic policy gradient, fails to find long time-of-flight transfers, we extend it by explicitly separating a null action from the continuous action space. We demonstrate our algorithm successfully finds long transfers from a wide variety of initial states in both two and three dimensional problems.
更多查看译文
关键词
deep reinforcement learning,exploration,time-of-flight,three-body
AI 理解论文
溯源树
样例
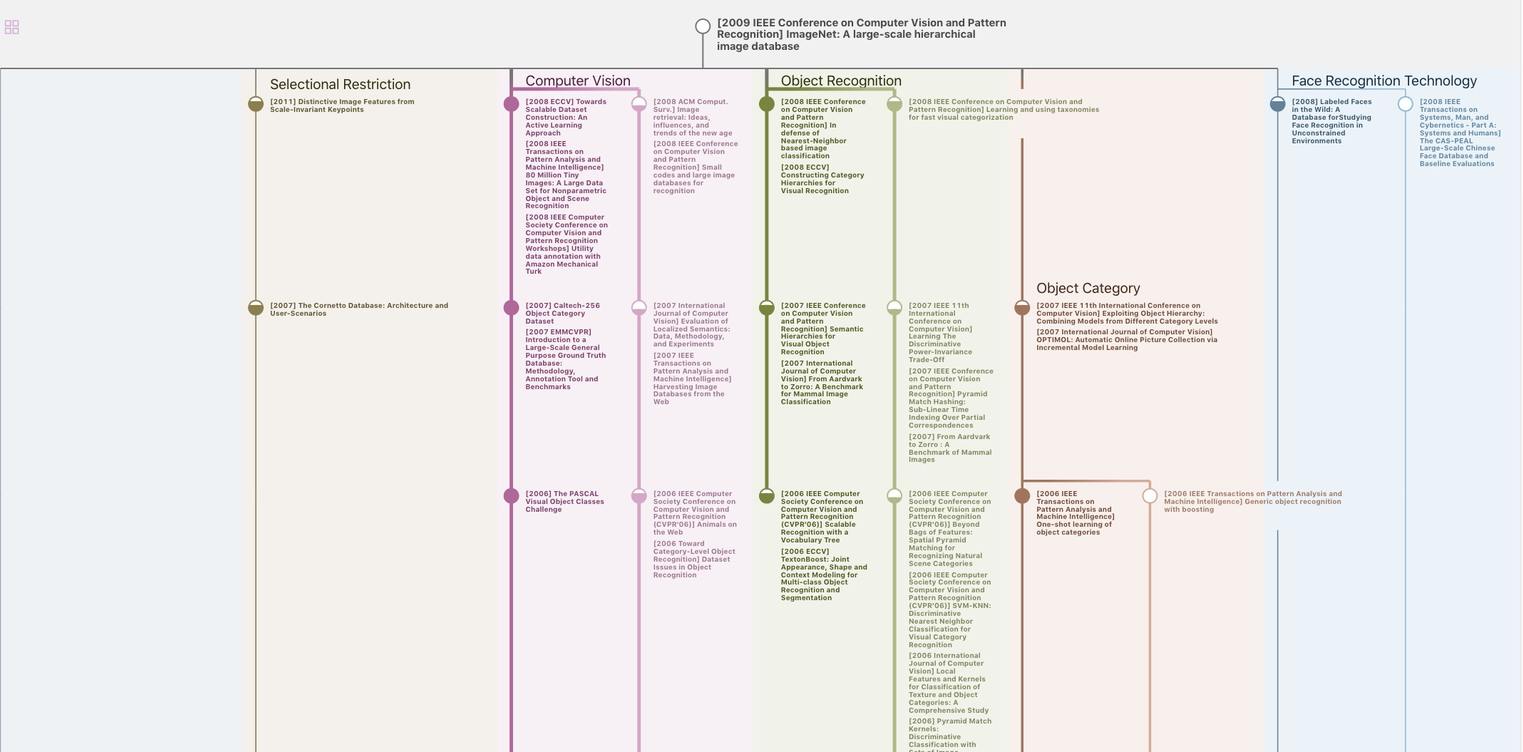
生成溯源树,研究论文发展脉络
Chat Paper
正在生成论文摘要