Multi-scale Deep Convolutional Neural Networks for Emphysema Classification and Quantification
Intelligent Systems Reference LibraryDeep Learning in Healthcare(2019)
摘要
In this work, we aim at classification and quantification of emphysema in computed tomography (CT) images of lungs. Most previous works are limited to extracting low-level features or mid-level features without enough high-level information. Moreover, these approaches do not take the characteristics (scales) of different emphysema into account, which are crucial for feature extraction. In contrast to previous works, we propose a novel deep learning method based on multi-scale deep convolutional neural networks. There are three contributions for this paper. First, we propose to use a base residual network with 20 layers to extract more high-level information. Second, we incorporate multi-scale information into our deep neural networks so as to take full consideration of the characteristics of different emphysema. A 92.68% classification accuracy is achieved on our original dataset. Finally, based on the classification results, we also perform the quantitative analysis of emphysema in 50 subjects by correlating the quantitative results (the area percentage of each class) with pulmonary functions. We show that centrilobular emphysema (CLE) and panlobular emphysema (PLE) have strong correlation with the pulmonary functions and the sum of CLE and PLE can be used as a new and accurate measure of emphysema severity instead of the conventional measure (sum of all subtypes of emphysema). The correlations between the new measure and various pulmonary functions are up to |r| \(= 0.922\) (r is correlation coefficient).
更多查看译文
关键词
emphysema classification,multi-scale
AI 理解论文
溯源树
样例
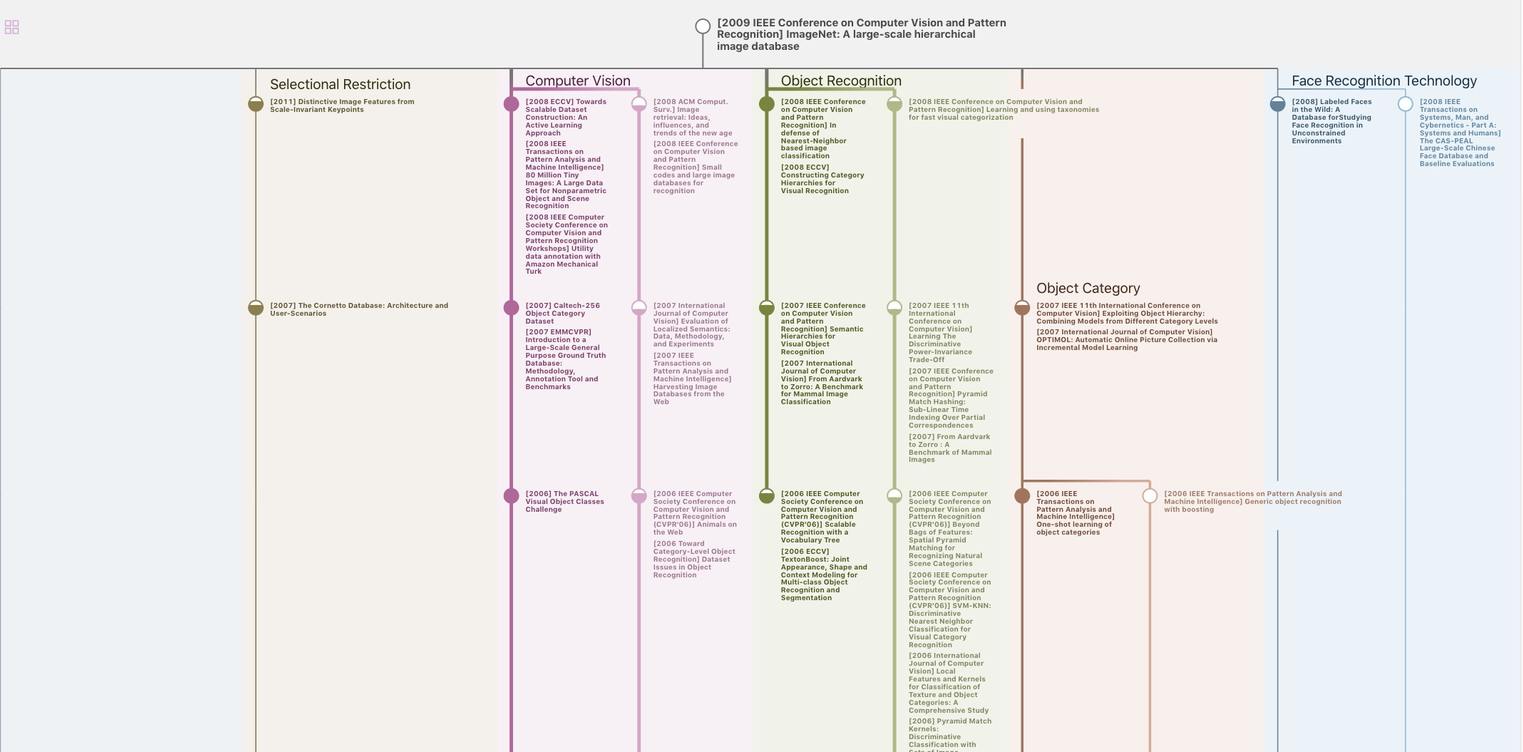
生成溯源树,研究论文发展脉络
Chat Paper
正在生成论文摘要