High‐Throughput Approaches for Phenotyping Alfalfa Germplasm under Abiotic Stress in the Field
The Plant Phenome Journal(2019)
摘要
Core Ideas Remote sensing technologies enable rapid and nondestructive phenotyping of plants in the field. Biomass estimate accuracy from images and sensors was similar to yields harvested manually. Biomass yield variation identified the most productive accessions under low‐input conditions. High‐throughput phenotyping technologies enable monitoring plant growth and development nondestructively throughout the growing season. Crop losses associated with abiotic and biotic factors threaten the sustainability of crops used for feed, fiber, and fuel. The process to develop improved cultivars with enhanced crop yields includes phenotyping hundreds of plants under a target set of conditions. However, the manual collection of data is often laborious and time consuming. Strategies that integrate remote sensing technologies including unmanned aerial vehicles (UAVs) and sensors mounted on “phenomobiles” can streamline phenotyping efforts in plant breeding programs. The objectives of this study were to compare the phenotypic data collected from the field using UAVs, sensors, and manual approaches and to assess the potential of high‐throughput approaches to rank the productivity of alfalfa ( Medicago sativa L.) accessions growing in the field. Phenotypic data were collected from 100 alfalfa accessions established and grown under low‐input conditions. Specific traits evaluated using both UAVs and sensors mounted on a phenomobile prior to physically harvesting the biomass during four harvests include biomass yield, plant height, normalized difference vegetation index, leaf area index, and ground coverage. The results from both the UAV and the sensors were highly correlated to the physical measurements obtained for the multiple traits evaluated. Therefore, field‐based high‐throughput phenotyping strategies represent a viable option for efficiently screening germplasm in the field to increase phenotyping efficiencies in plant breeding programs.
更多查看译文
关键词
Biomass Estimation,Crop Yield Prediction,Plant Disease Detection,Vegetation Monitoring,Smart Farming
AI 理解论文
溯源树
样例
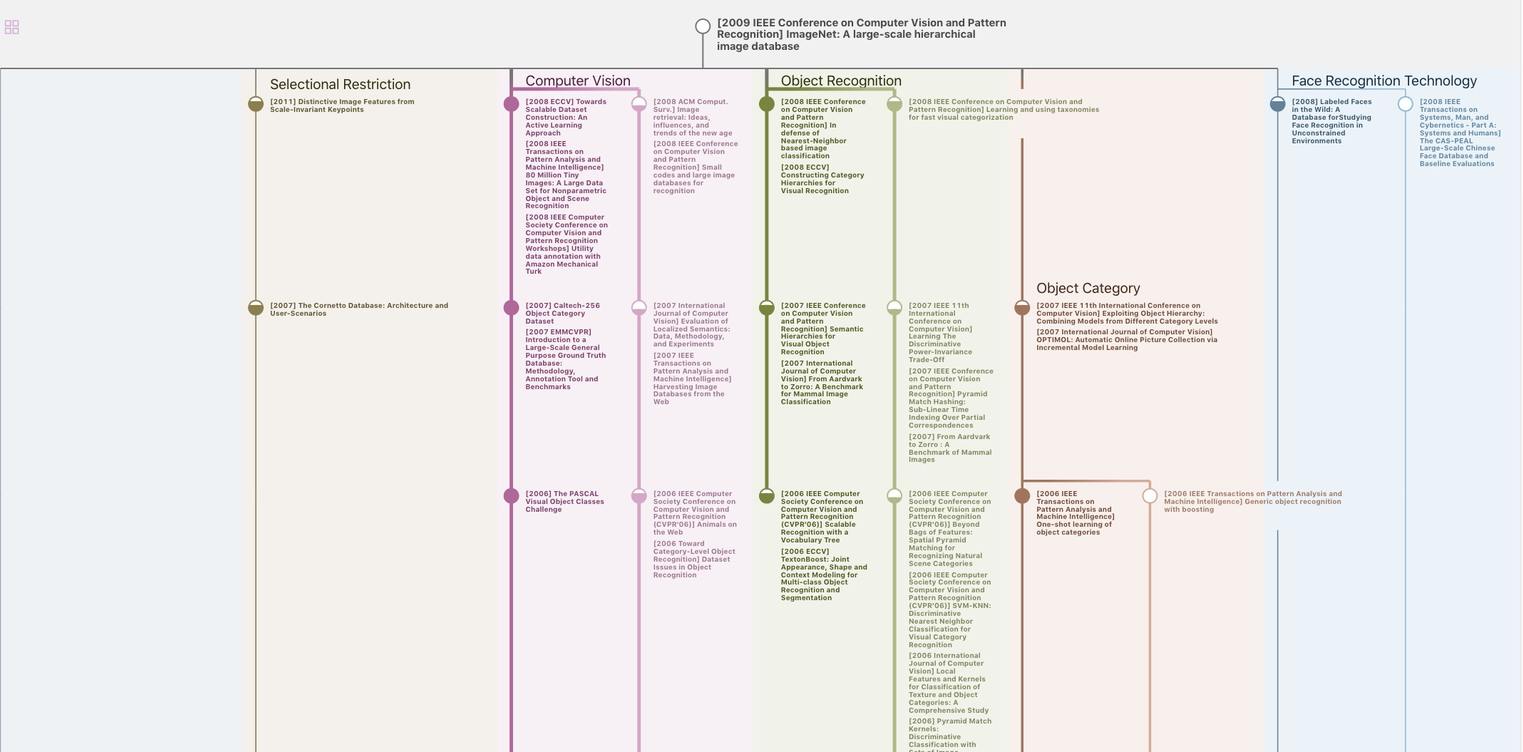
生成溯源树,研究论文发展脉络
Chat Paper
正在生成论文摘要