Predicting Horizontal Shear Slowness- A Machine Learning Approach
Day 2 Tue, November 12, 2019(2019)
摘要
Abstract The Spraberry trend area is part of a larger oil-producing region within the Midland basin in United States. The main targets, Spraberry, Dean and Wolfcamp, are reservoirs of shales interbedded with clastic formations. Thus, the reservoirs exhibit TIV (transverse isotropy vertical) anisotropy due to thin laminations. A pilot well was drilled vertically in the complex lithology and logged with the advanced acoustics measurements. Shallow penetration of Stoneley energy into the formation raised concerns about the depth resolution of the inverted shear slowness derived from it. It is very difficult to get a reliable horizontal shear slowness from Stoneley when the borehole condition is rugose, there is a complex mud rheology and gas influx inside the borehole. A machine learning based approach integrating the advanced acoustics measurements and petrophysical interpretation is adopted to provide the solution to get the lithology-based horizontal shear slowness. To eliminate the variability of getting the horizontal shear slowness from Stoneley wave, to process for an advanced geomechanics product like for TIV anisotropy analysis, two machine learning algorithms are used. First one is a very commonly used linear supervised learning algorithm multi-linear regression (MLR) and second is random forest (RF) a nonlinear supervised learning algorithm. These algorithms take inputs from formation evaluation and advanced acoustics to predict the horizontal shear slowness. The random forest algorithm being an ensemble learning method have greater predictive capabilities compared with any linear supervised learning models and many of the non-linear supervised learning algorithms. The inputs for RF and MLR regressions are values of dry weight fractions of calcite, dolomite, quartz, illite, total porosity, permeability, gamma ray, compressional slowness and fast shear slowness. These values are obtained for the entire depth of interest from advance logging tools and interpretation techniques. To check the performance of the model, standard machine learning techniques such as the error evaluation metrics of the mean squared error and the coefficient of determination (R-squared or R2) were considered. The model has been trained over 90% of data and 10% of the data was used to cross-validate the model. Hyperparameter tuning of the RF model has been done to improve upon the prediction accuracy. After the parameters are tuned, the mean squared error and R2 value of the training dataset are 1.77 and 0.98; while that for the testing dataset, they are 13.26 and 0.89 respectively. The closeness of the R2 value for both the training and testing dataset to 1, implies that the RF model is successfully able to explain the variance of the given data.
更多查看译文
AI 理解论文
溯源树
样例
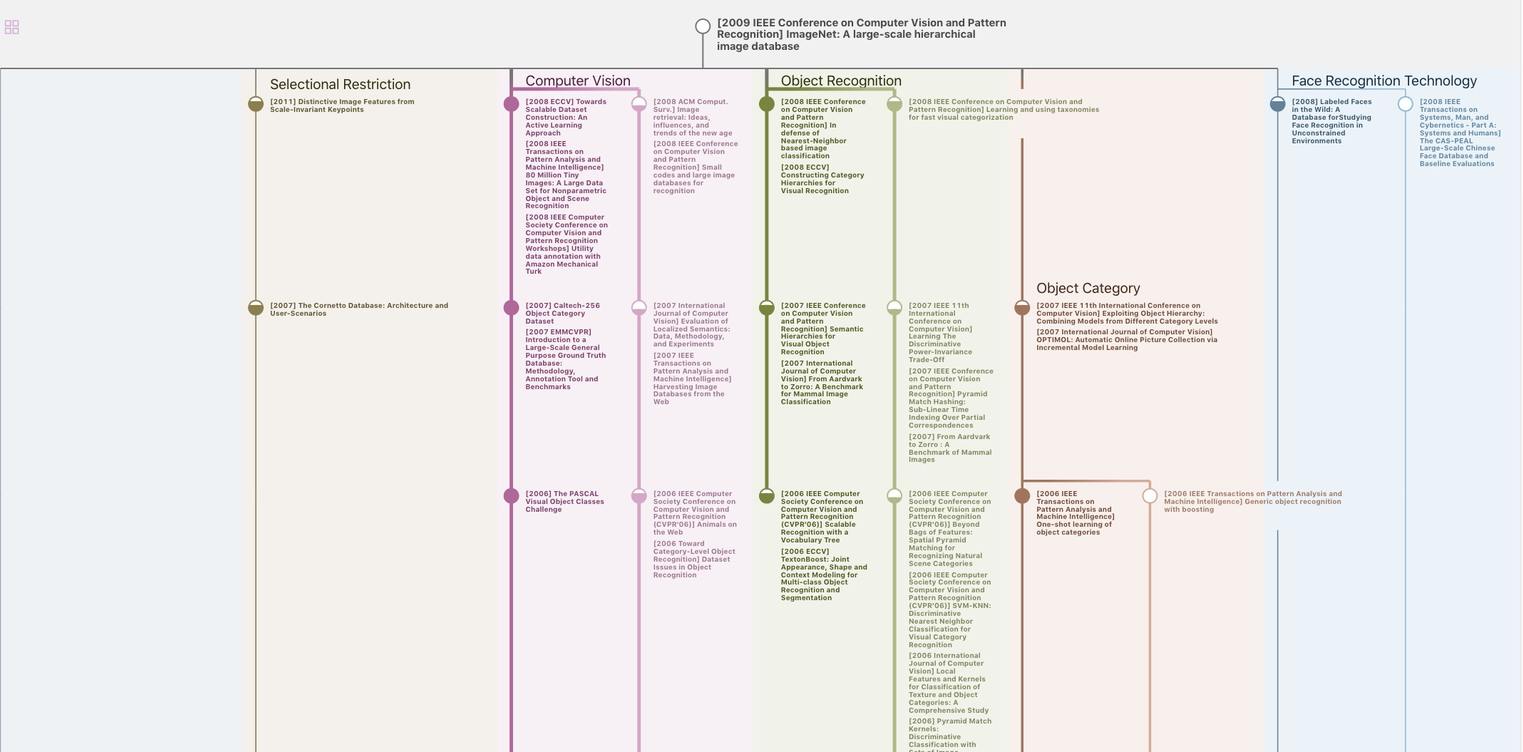
生成溯源树,研究论文发展脉络
Chat Paper
正在生成论文摘要