Ternary Compute-Enabled Memory using Ferroelectric Transistors for Accelerating Deep Neural Networks
2020 Design, Automation & Test in Europe Conference & Exhibition (DATE)(2020)
Abstract
Ternary Deep Neural Networks (DNNs), which employ ternary precision for weights and activations, have recently been shown to attain accuracies close to full-precision DNNs, raising interest in their efficient hardware realization. In this work we propose a Non-Volatile Ternary Compute-Enabled memory cell (TeC-Cell) based on ferroelectric transistors (FEFETs) for inmemory computing in the signed ternary regime. In particular, the proposed cell enables storage of ternary weights and employs multi-word-line assertion to perform massively parallel signed dot-product computations between ternary weights and ternary inputs. We evaluate the proposed design at the array level and show 72% and 74% higher energy efficiency for multiply-andaccumulate (MAC) operations compared to standard nearmemory computing designs based on SRAM and FEFET, respectively. Furthermore, we evaluate the proposed TeC-Cell in an existing ternary in-memory DNN accelerator. Our results show 3.3X-3.4X reduction in system energy and 4.3X-7X improvement in system performance over SRAM and FEFET based nearmemory accelerators, across a wide range of DNN benchmarks including both deep convolutional and recurrent neural networks.
MoreTranslated text
Key words
Deep Neural Networks,Dot-Product,Ferroelectric Transistors,In-Memory Computing,Low-Precision,Multiply-andAccumulate,Ternary DNN
AI Read Science
Must-Reading Tree
Example
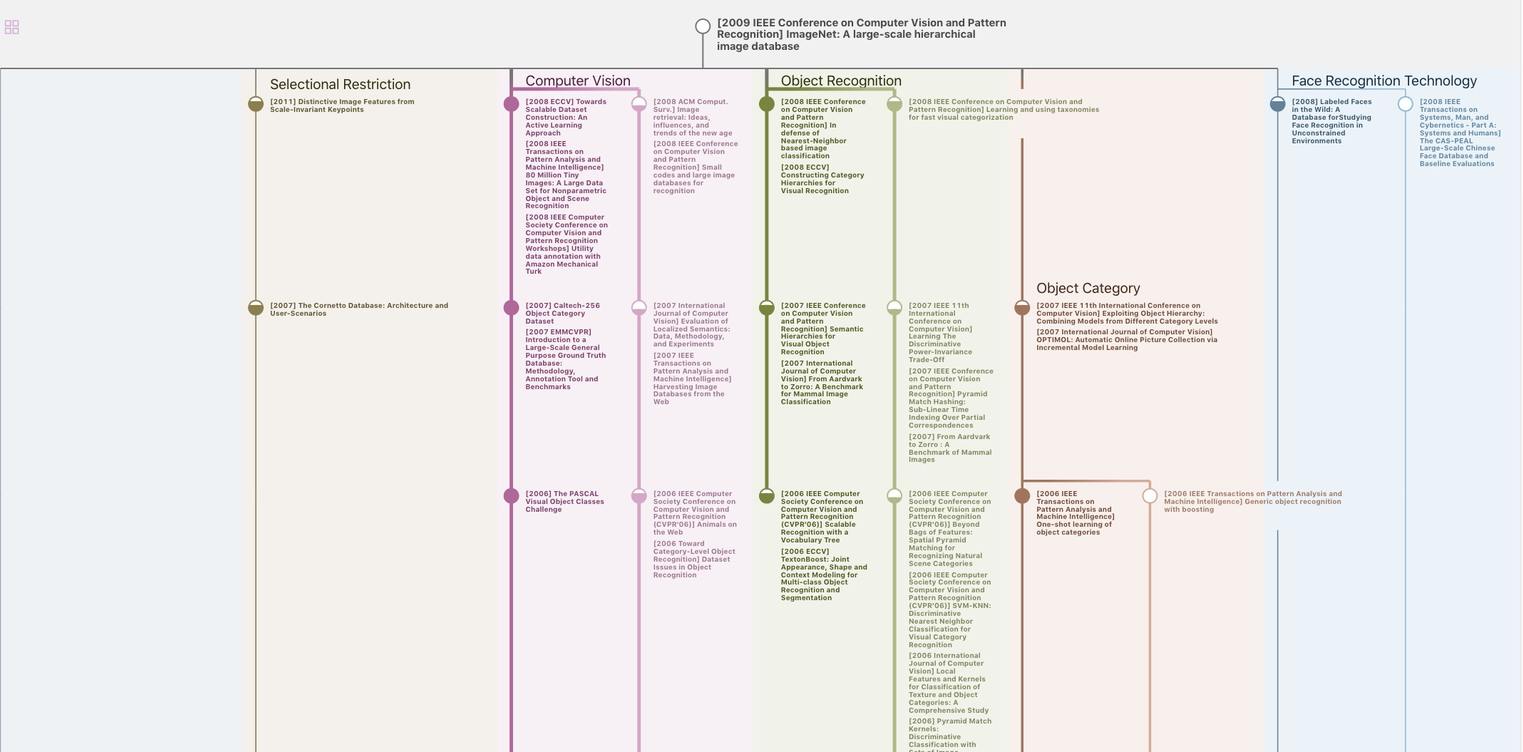
Generate MRT to find the research sequence of this paper
Chat Paper
Summary is being generated by the instructions you defined