Assessment of Big Data Analytics Based Ensemble Estimator Module for the Real-Time Prediction of Reservoir Recovery Factor
Day 4 Thu, March 21, 2019(2019)
摘要
Abstract Production of oil & gas depends upon the recoverable amount of hydrocarbon existing beneath the underlying reservoir. Reservoir recovery factor provides of the production potential of ‘proven reservoirs’ which helps the planning of field development and production. Estimation of reservoir recovery factor, with a good degree of accuracy, is still a challenging task for engineers due to the high level of uncertainty, large inexactness, noise, and high dimensionality associated with reservoir measurements. In this paper, we propose a big data-driven ‘ensemble estimator’ (E2) module, comprising of wavelet associated ensemble models for the estimation of reservoir recovery factor. All the ensemble models in E2 were trained on big reservoir data and tested with unknown reservoir data samples obtained from U.S.A. oil & gas fields. Bagging and Random forest ensembles have been utilized to correlate several reservoir properties with reservoir recovery factor. Further, E2 utilizes Relief algorithm to understand the significance of reservoir properties effecting the recovery factor of a reservoir. The proposed E2 module has provided impressive estimation results for the determination of reservoir recovery factor with minimum prediction error. Random forest has given the highest coefficient of correlation (R2=0.9592) and minimum estimation errors viz. mean absolute error (MAE=0.0234) and root mean square error (RMSE=0.0687). The performance of the proposed E2 module was also compared with conventional estimators viz. Radial basis function, Multilayer perceptron, Regression tree and Support vector regression. The experimental results have demonstrated the supremacy of E2 over conventional learners for the estimation of reservoir recovery factor.
更多查看译文
AI 理解论文
溯源树
样例
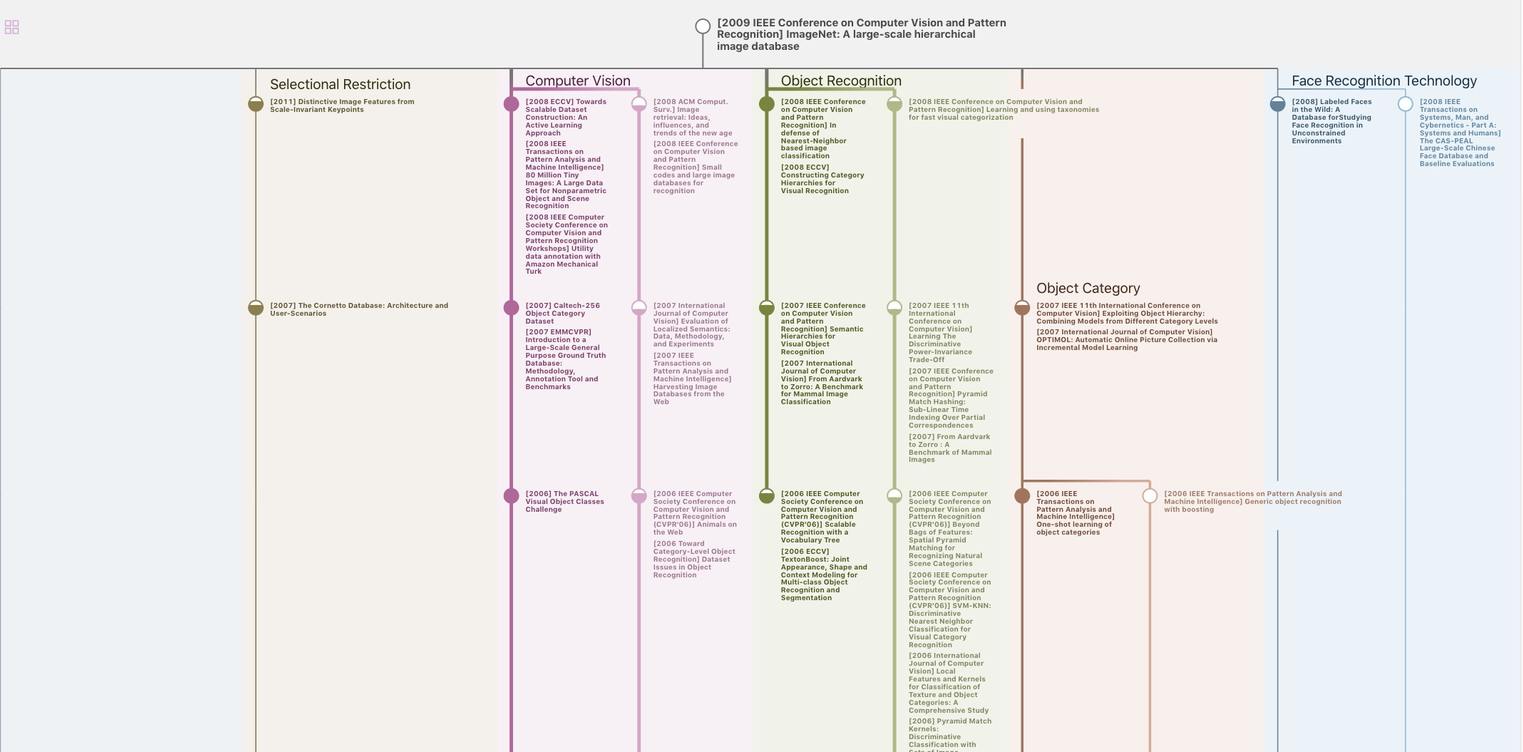
生成溯源树,研究论文发展脉络
Chat Paper
正在生成论文摘要