Reconstructing Bifurcation Diagrams of Induction Motor Drives Using an Extreme Learning Machine
Proceedings in Adaptation, Learning and OptimizationProceedings of ELM-2017(2018)
Abstract
We describe a method to reconstruct the bifurcation diagrams of a mathematical model of induction motor drives using extreme learning machines. The reconstruction of a bifurcation diagram estimates the oscillatory patterns of a target system when its parameters are changed. These patterns can be periodic, quasi-periodic, or chaotic. Examples of the parameters are electric current and power, temperature, pressure, and concentration. We also estimate Lyapunov exponents so that the reconstructed bifurcation diagram can be quantitatively evaluated. We use typical chaotic systems as target for the reconstruction of bifurcation diagrams, such as logistic and Henon maps, as well as Rossler equations. Using numerical simulations, we show that the reconstruction of bifurcation diagrams can be useful for real-world systems, such as induction motor drives. For applying the method to the real-world systems, we evaluate robustness for observation noise. Therefore, we attempt to reconstruct the bifurcation diagram of the induction motor drives using noisy time-series datasets.
MoreTranslated text
Key words
Extreme learning machine, Chaos, Reconstruction of bifurcation diagrams, Time-series prediction, Induction motor drive
AI Read Science
Must-Reading Tree
Example
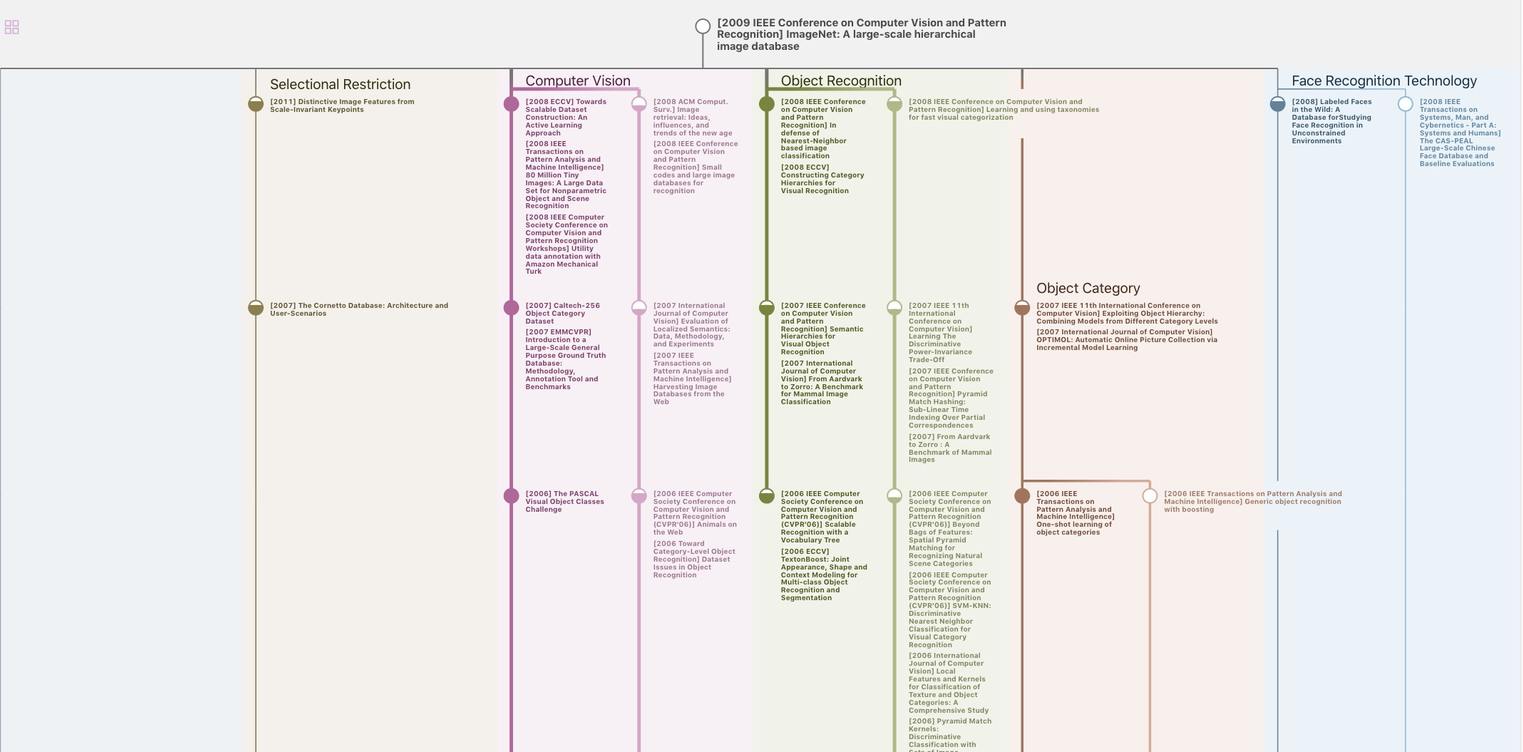
Generate MRT to find the research sequence of this paper
Chat Paper
Summary is being generated by the instructions you defined