Anomaly Detection for Hyperspectral Images Based on Anisotropic Spatial-Spectral Total Variation and Sparse Constraint
MATEC Web of Conferences(2018)
摘要
A novel anomaly detection method for hyperspectral images (HSIs) is proposed based on anisotropic spatial-spectral total variation and sparse constraint. HSIs are assumed to be not only smooth in spectral dimension but also piecewise smooth in spatial dimension. The proposed method adopts the anisotropic spatial-spectral total variation model which combines 2D spatial total variation and 1D spectral variation to explore the spatial-spectral smooth property of HSIs. Meanwhile, the sparse property of anomalies is exploited for its low probability in the image. To utilize both the spatial and spectral information of HSIs, we preserve the original cubic form of HSIs and divide the HSIs into three 3D arrays, each representing the background, the anomaly, and the noise respectively. By using anisotropic spatial-spectral total variation regularization on the background component and sparse constraint on the anomaly component, this anomaly detection problem has therefore been formulated as a constraint optimization problem whose solution has been derived by alternately using Split Bregman Method and Go Decomposition (GoDec) Method. Experimental results on hyperspectral datasets illustrate that our proposed method has a better detection performance than state-of-the-art hyperspectral anomaly detection methods.
更多查看译文
关键词
Hyperspectral Imaging,Hyperspectral,Spatial Pattern Analysis,Sparse Representations,Image Denoising
AI 理解论文
溯源树
样例
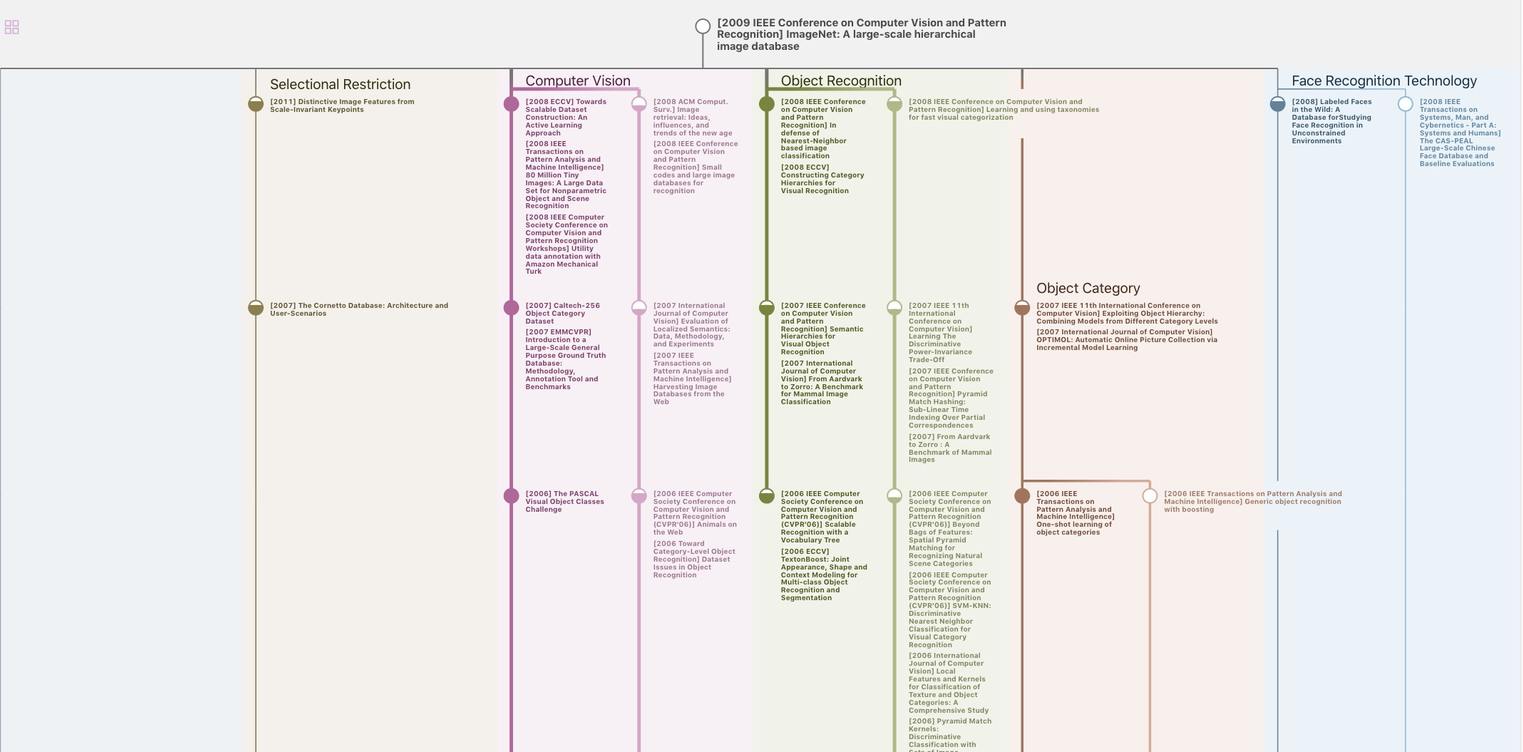
生成溯源树,研究论文发展脉络
Chat Paper
正在生成论文摘要