Discrete Shearlets as a Sparsifying Transform in Low-Rank Plus Sparse Decomposition for Undersampled (k, t)-Space MR Data
JOURNAL OF IMAGING(2022)
摘要
The discrete shearlet transformation accurately represents the discontinuities and edges occurring in magnetic resonance imaging, providing an excellent option of a sparsifying transform. In the present paper, we examine the use of discrete shearlets over other sparsifying transforms in a low-rank plus sparse decomposition problem, denoted by L + S. The proposed algorithm is evaluated on simulated dynamic contrast enhanced (DCE) and small bowel data. For the small bowel, eight subjects were scanned; the sequence was run first on breath-holding and subsequently on free-breathing, without changing the anatomical position of the subject. The reconstruction performance of the proposed algorithm was evaluated against k-t FOCUSS. L + S decomposition, using discrete shearlets as sparsifying transforms, successfully separated the low-rank (background and periodic motion) from the sparse component (enhancement or bowel motility) for both DCE and small bowel data. Motion estimated from low-rank of DCE data is closer to ground truth deformations than motion estimated from L and S. Motility metrics derived from the S component of free-breathing data were not significantly different from the ones from breath-holding data up to four-fold undersampling, indicating that bowel (rapid/random) motility is isolated in S. Our work strongly supports the use of discrete shearlets as a sparsifying transform in a L S decomposition for undersampled MR data.
更多查看译文
关键词
MRI, image reconstruction, robust principal component analysis (RPCA), discrete shearlet transform, low-rank plus sparse decomposition, dynamic contrast enhanced (DCE), small bowel imaging
AI 理解论文
溯源树
样例
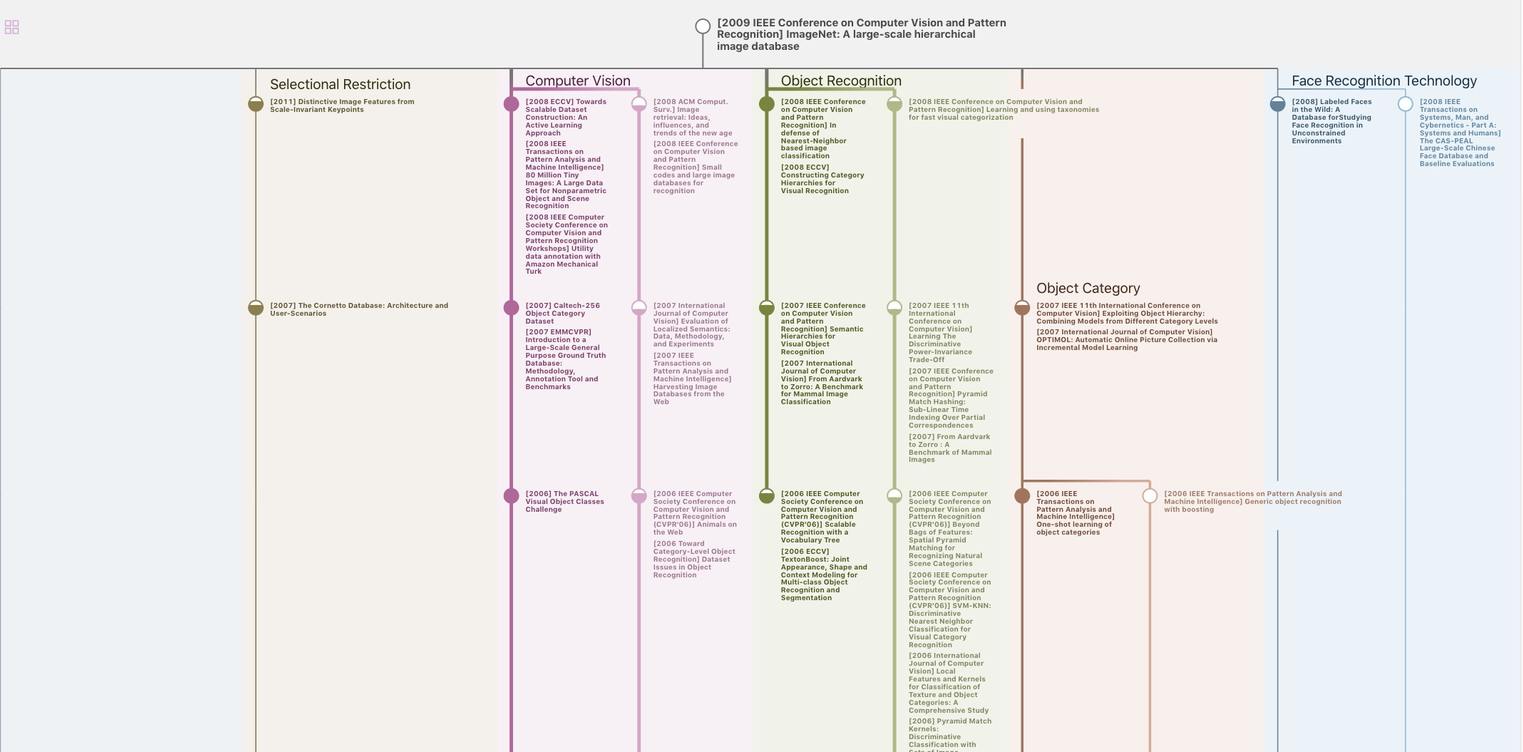
生成溯源树,研究论文发展脉络
Chat Paper
正在生成论文摘要