Cross-Boosted Multi-Target Domain Adaptation for Multi-Modality Histopathology Image Translation and Segmentation
IEEE Journal of Biomedical and Health Informatics(2022)
Abstract
Recent digital pathology workflows mainly focus on mono-modality histopathology image analysis. However, they ignore the complementarity between Haematoxylin & Eosin (H&E) and Immunohistochemically (IHC) stained images, which can provide comprehensive gold standard for cancer diagnosis. To resolve this issue, we propose a cross-boosted multi-target domain adaptation pipeline for multi-modality histopathology images, which contains Cross-frequency Style-auxiliary Translation Network (CSTN) and Dual Cross-boosted Segmentation Network (DCSN). Firstly, CSTN achieves the one-to-many translation from fluorescence microscopy images to H&E and IHC images for providing source domain training data. To generate images with realistic color and texture, Cross-frequency Feature Transfer Module (CFTM) is developed to pertinently restructure and normalize high-frequency content and low-frequency style features from different domains. Then, DCSN fulfills multi-target domain adaptive segmentation, where a dual-branch encoder is introduced, and Bidirectional Cross-domain Boosting Module (BCBM) is designed to implement cross-modality information complementation through bidirectional inter-domain collaboration. Finally, we establish Multi-modality Thymus Histopathology (MThH) dataset, which is the largest publicly available H&E and IHC image benchmark. Experiments on MThH dataset and several public datasets show that the proposed pipeline outperforms state-of-the-art methods on both histopathology image translation and segmentation.
MoreTranslated text
Key words
Benchmarking,Diffusion Magnetic Resonance Imaging,Humans,Image Processing, Computer-Assisted,Microscopy, Fluorescence,Workflow
AI Read Science
Must-Reading Tree
Example
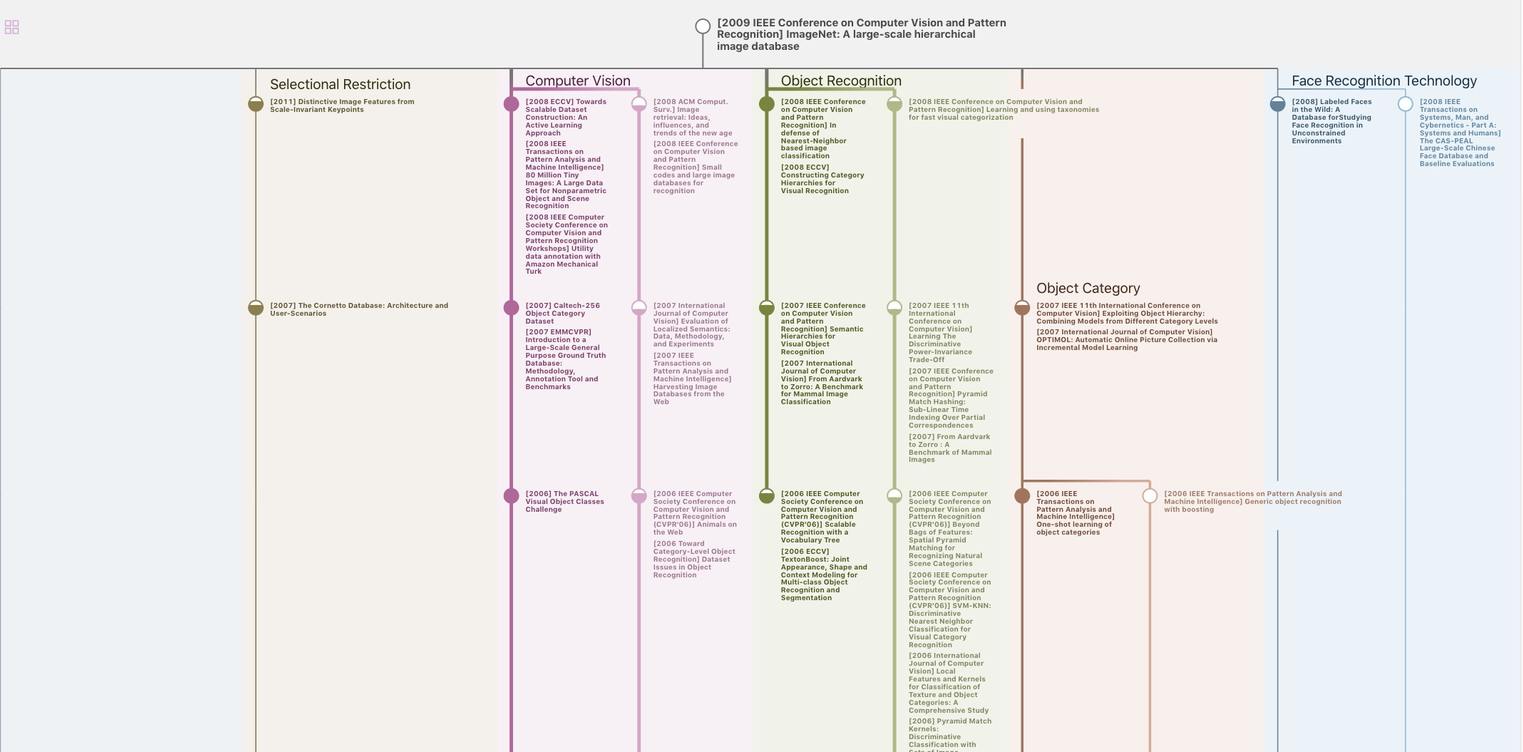
Generate MRT to find the research sequence of this paper
Chat Paper
Summary is being generated by the instructions you defined