Time Series Forecasting for Healthcare Diagnosis and Prognostics with the Focus on Cardiovascular Diseases
6th International Conference on the Development of Biomedical Engineering in Vietnam (BME6)IFMBE Proceedings(2017)
Abstract
Time series forecasting has been a prosperous filed of science due to its popularity in real-world applications, yet being challenge in method developments. In medical applications, time series forecasting models have been successfully applied to predict the progress of the disease, estimate the mortality rate and assess the time dependent risk. However, the vast availability of many different techniques, in which each type excels in particular situations, makes the process of choosing an appropriate model more challenging. Therefore, the aim of this paper is to summarize and review different types of forecasting model that have been tremendously cultured for medical purposes using time series based forecasting methods. For each type of model, we will list the current related research papers, briefly describe the underlying theories, and discuss its advantages and disadvantages within different clinical situations. At the end, this paper also provides a robust and purpose-oriented classification of about 60 different forecasting models, therefore providing a comprehensive references for scientists and researchers to determine the suitable forecasting models for their case of study.
MoreTranslated text
Key words
Healthcare diagnostics and prognostics, Cardiovascular disorder, Time series forecasting
AI Read Science
Must-Reading Tree
Example
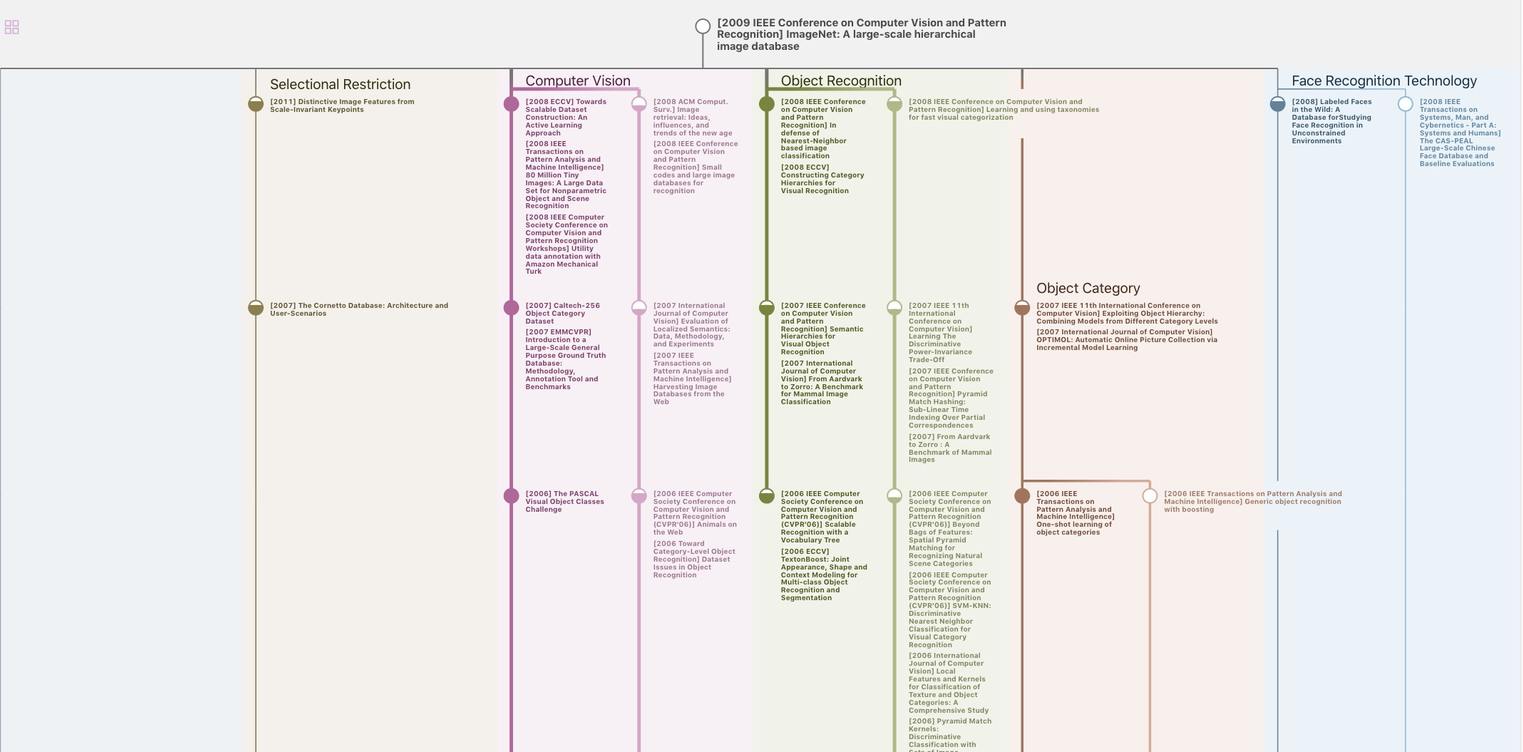
Generate MRT to find the research sequence of this paper
Chat Paper
Summary is being generated by the instructions you defined