A preliminary study for development of an automatic incident detection system on CCTV in tunnels based on a machine learning algorithm
Journal of Korean Tunnelling and Underground Space Association(2017)
Abstract
본 논문에서는 제도적으로 운영 중인 터널내 CCTV들로부터 실시간으로 들어오는 영상들을 최신 딥러닝 알고리즘을 이용, 학습시켜 다양한 조건의 터널환경에서 돌발 상황을 감지하고 그 돌발 상황의 종류들을 분류해 내는 시스템 개발을 위한 사전검토 연구를 수행하였다. 사전검토 연구를 위해, 2개의 도로현장의 교통류 CCTV영상 일부를 이용하여 가용한 전통적인 영상처리기법으로 영상내부로 집입하는 차량을 감지하고, 이동경로를 추적하여 일정 시간간격의 이동 차량의 좌표와 시간정보를 추출하고 학습자료를 구성하였다. 각 차량의 이동정보는 차선변경, 정차 등 6가지의 이벤트 정보와 연계된다. 차량 이동정보와 이벤트로 구성된 학습자료는 레질리언스(resilience) 기계학습 알고리즘을 이용하여 학습하였다. 2개의 은닉층을 설정하고, 각 은닉층의 노드수에 대한 9개의 은닉구조 모델을 설정하여 매개변수 연구를 수행하였다. 본 사전검토의 경우에는 첫 번째, 두 번째 은닉층 노드수가 각각 300개와 150개로 설정된 모델이 합리적으로 가장 추론정확도가 높은 것으로 평가되었다. 이로부터 일반화되기 매우 힘든 복잡한 교통류 상황을 기계학습을 이용하여 어떠한 사전 규칙설정 없이도 교통류의 특징들을 정확히 자동으로 감지할 수 있는 가능성을 보였다. 본 시스템은 시스템의 운용을 통해 지속적으로 교통류 영상과 이벤트 정보가 늘어난다면, 자동으로 그 시스템의 인지능력과 정확도가 자동으로 향상되는 효과도 기대할 수 있다. In this study, a preliminary study was undertaken for development of a tunnel incident automatic detection system based on a machine learning algorithm which is to detect a number of incidents taking place in tunnel in real time and also to be able to identify the type of incident. Two road sites where CCTVs are operating have been selected and a part of CCTV images are treated to produce sets of training data. The data sets are composed of position and time information of moving objects on CCTV screen which are extracted by initially detecting and tracking of incoming objects into CCTV screen by using a conventional image processing technique available in this study. And the data sets are matched with 6 categories of events such as lane change, stoping, etc which are also involved in the training data sets. The training data are learnt by a resilience neural network where two hidden layers are applied and 9 architectural models are set up for parametric studies, from which the architectural model, 300(first hidden layer)-150(second hidden layer) is found to be optimum in highest accuracy with respect to training data as well as testing data not used for training. From this study, it was shown that the highly variable and complex traffic and incident features could be well identified without any definition of feature regulation by using a concept of machine learning. In addition, detection capability and accuracy of the machine learning based system will be automatically enhanced as much as big data of CCTV images in tunnel becomes rich.
MoreTranslated text
Key words
automatic incident detection system,cctv,machine learning,tunnels
AI Read Science
Must-Reading Tree
Example
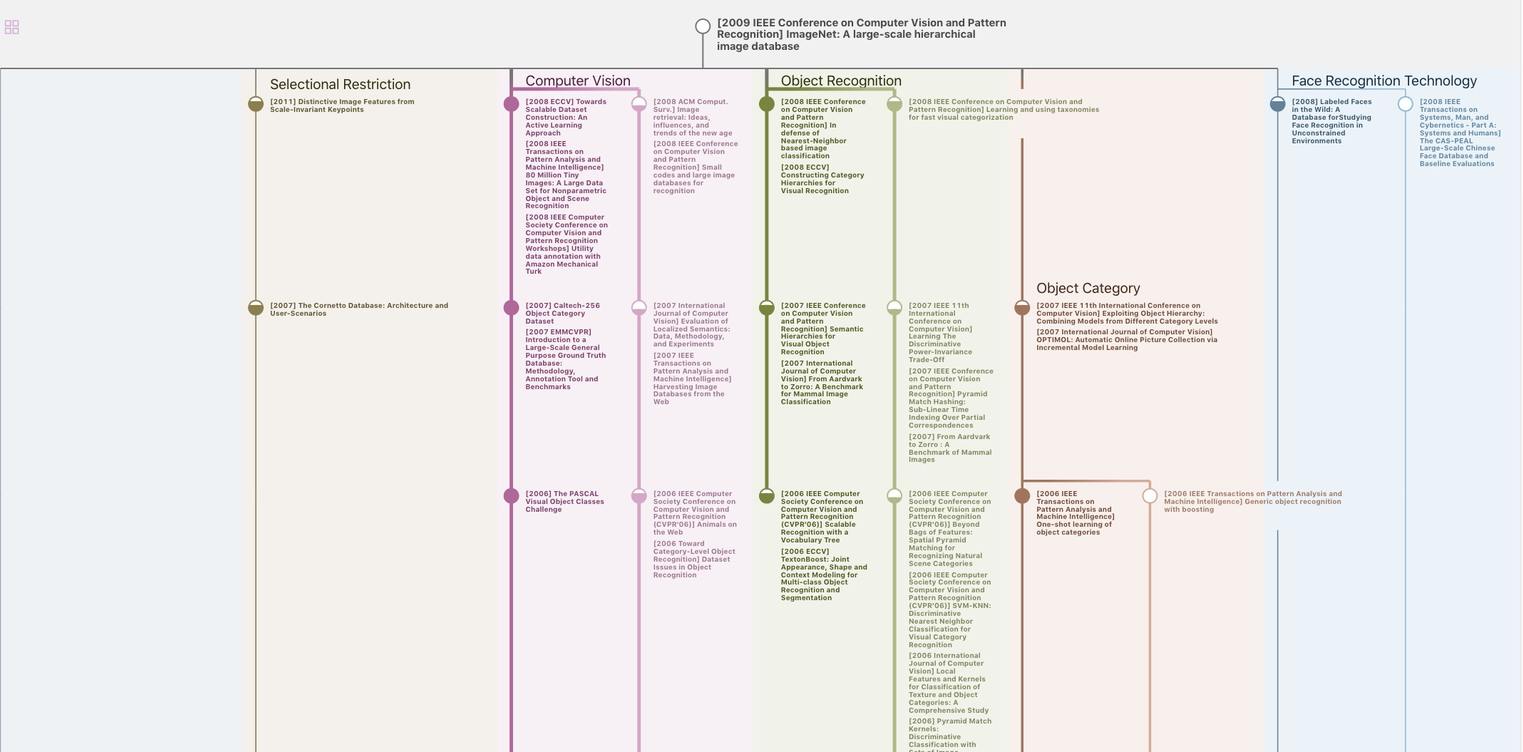
Generate MRT to find the research sequence of this paper
Chat Paper
Summary is being generated by the instructions you defined